Neural network modeling of greenhouse tomato yield, growth and water use from automated crop monitoring data
Computers and Electronics in Agriculture(2011)
摘要
The recent development of tools to automatically monitor important crop attributes in situ such as yield, growth and water use offers an opportunity to relate real-time crop status to current environmental conditions. In this study, continuous minute-by-minute measurements of crop yield, growth and water use averaged over weekly, daily, or hourly intervals throughout the growing season were used to determine crop response to changes in the greenhouse environment. The data were obtained from crop monitoring stations established in both commercial and research greenhouses. Crop yield measurements obtained from the monitoring system were generally in very close agreement with yields recorded over a much larger area in the commercial greenhouse. Yield was more closely related (R^2=0.65) to radiation from the previous week than to radiation in the current week (R^2=0.56). In addition, a neural network (NN) model of yield which included radiation as an input was better at predicting yield in the following week (R^2=0.70) than yield in the current week (R^2=0.57). These results indicate a lag effect of radiation on yield. Similarly, yield was more positively related to growth from the previous week (R^2=0.32) than to growth from the current week (R^2=0.17). Neural network models of daily growth at both sites (R^2=0.74, 0.69) included day of the year, temperature and CO"2 as inputs. A negative relationship between day of the year and daily growth indicates a decline in crop vigor through the measurement period. Neural network models of daily crop water use for the two sites were stronger (R^2=0.91, 0.85) than those for growth, highlighting the difference in physiological complexity between the two. A model of canopy water status as affected by environmental conditions was generated using hourly measures of tomato canopy mass change. Although the rate of canopy mass gain through the day was often constant, there were days when the plant experienced periods of reduced mass gain mid-day. On those days, the amount of deviation from a constant rate was positively related to radiation, day temperature and water use, suggesting periods of water stress. With subsequent recovery of mass gain rates late afternoon, these deviations did not affect canopy growth for the day. Overall, automated monitoring provides new information on the crop which may readily be incorporated into models of crop performance.
更多查看译文
关键词
Automation,Crop monitoring,Crop models,Greenhouse,Neural networks,Tomato
AI 理解论文
溯源树
样例
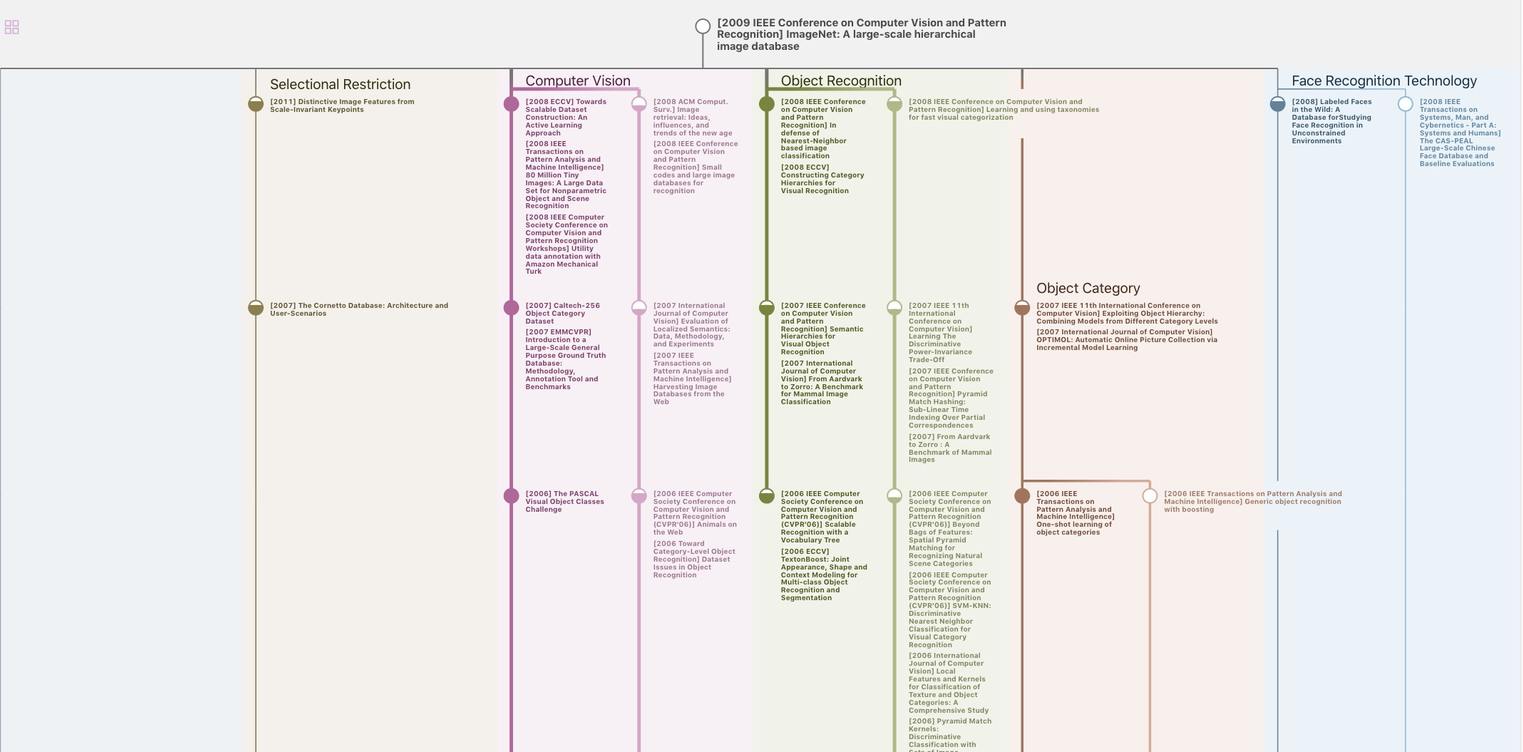
生成溯源树,研究论文发展脉络
Chat Paper
正在生成论文摘要