Fusing appearance and distribution information of interest points for action recognition
Pattern Recognition(2012)
摘要
Most of the existing action recognition methods represent actions as bags of space-time interest points. Specifically, space-time interest points are detected from the video and described using appearance-based descriptors. Each descriptor is then classified as a video-word and a histogram of these video-words is used for recognition. These methods therefore rely solely on the discriminative power of individual local space-time descriptors, whilst ignoring the potentially useful information about the global spatio-temporal distribution of interest points. In this paper we propose a novel action representation method which differs significantly from the existing interest point based representation in that only the global distribution information of interest points is exploited. In particular, holistic features from clouds of interest points accumulated over multiple temporal scales are extracted. Since the proposed spatio-temporal distribution representation contains different but complementary information to the conventional Bag of Words representation, we formulate a feature fusion method based on Multiple Kernel Learning. Experiments using the KTH and WEIZMANN datasets demonstrate that our approach outperforms most existing methods, in particular under occlusion and changes in view angle, clothing, and carrying condition.
更多查看译文
关键词
interest point,fusing appearance,existing action recognition method,existing method,complementary information,space-time interest point,global distribution information,words representation,existing interest point,proposed spatio-temporal distribution representation,novel action representation method
AI 理解论文
溯源树
样例
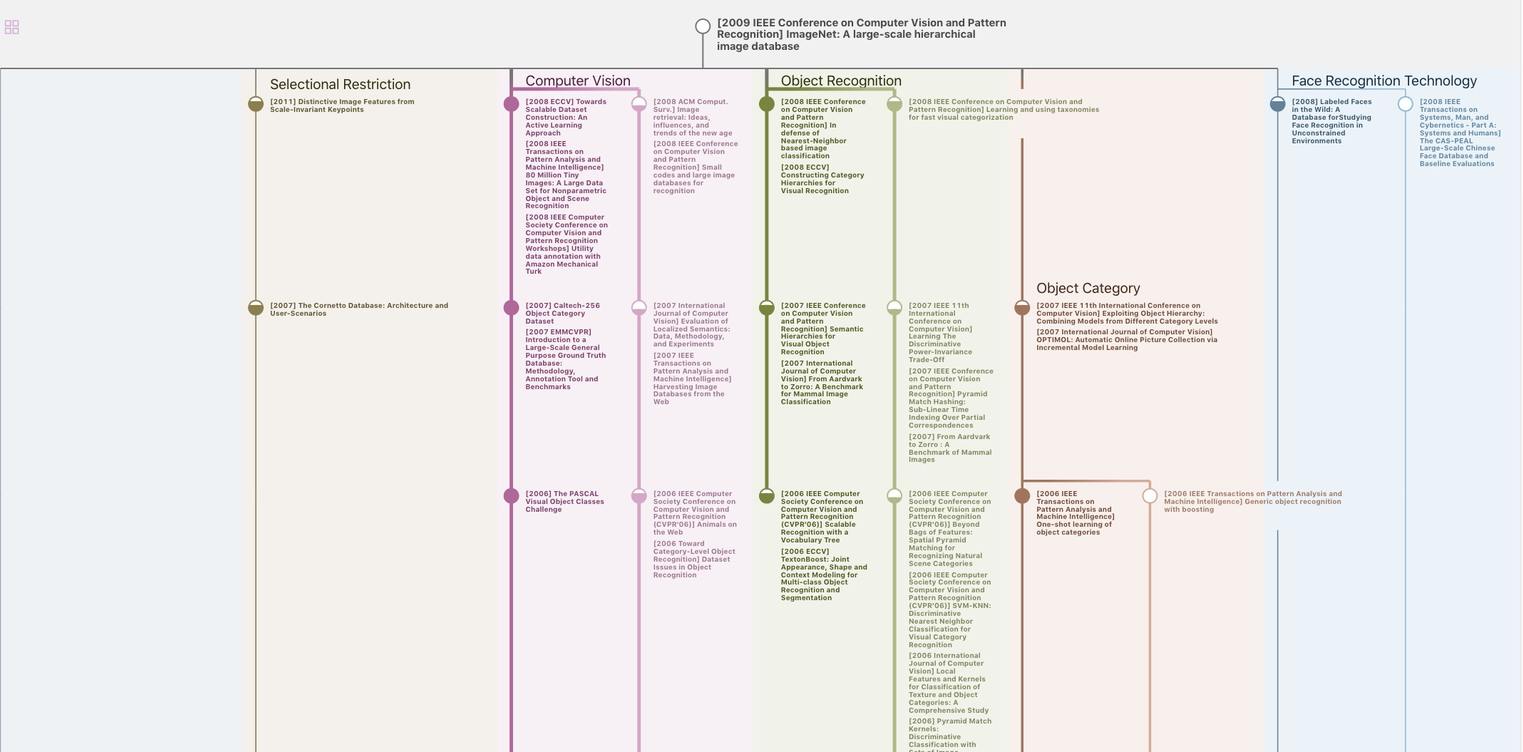
生成溯源树,研究论文发展脉络
Chat Paper
正在生成论文摘要