A hierarchical latent topic model based on sparse coding
Neurocomputing(2012)
摘要
We propose a novel hierarchical latent topic model based on sparse coding in this paper. Unlike the other topic models applied in the computer vision field, the words in our model are not discrete but continuous. They are generated by sparse coding and represented with n-dimensional vectors in R^n. In sparse coding, only a small set of components of each word is active, so we assume the probability distribution over these continuous words is Laplace and the parameters of the Laplace distribution depend on topics, which are the latent variables in this model. The relationship among word, topic, document and corpus in our model is similar to Latent Dirichlet Allocation (LDA). Thereby this model is a generalization of the traditional LDA by introducing the concept-continuous words. We use an EM algorithm to estimate the parameters in our model. And the proposed method is applied to some significant computer vision problems such as natural scene categorization and object classification. The experimental results show the method is a valuable direction to generalize topic models.
更多查看译文
关键词
continuous word,hierarchical latent topic model,concept-continuous word,sparse coding,novel hierarchical latent topic,topic model,latent variable,laplace distribution,computer vision field,probability distribution
AI 理解论文
溯源树
样例
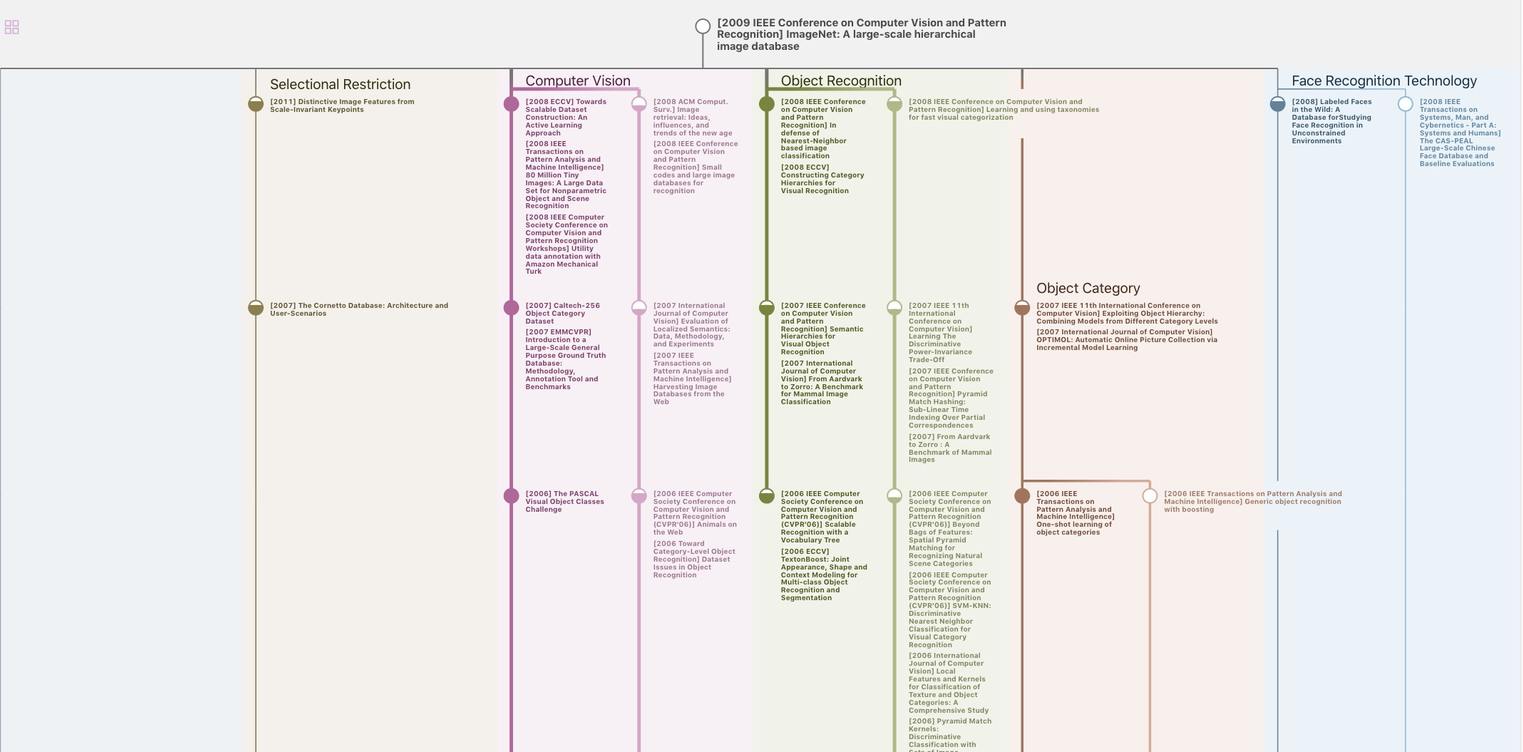
生成溯源树,研究论文发展脉络
Chat Paper
正在生成论文摘要