Fast Classification of Electrocardiograph Signals via Instance Selection
Healthcare Informatics, Imaging and Systems Biology(2011)
摘要
In clinical practice, electrocardiographs (ECG)are used in various ways. In the most simple case, directly after the ECG has been recorded, the doctor analyses it and makes the diagnosis. In other cases, e.g. when the abnormality can only be observed occasionally, at a previously unknown time, the ECG is being recorded continuously. Fast automatic recognition of abnormalities of ECG signals may substantially support doctors' work in both cases: either by immediately displaying a warning or calling the emergency service in case of danger or by pointing to the abnormal parts of a long ECG-signal in order to support analysis and diagnosis. In this paper, we focus on the (semi-)automated recognition of abnormal ECG signals. We formulate the task as a time-series classification problem, point out that state-of-the-art solutions are capable to solve this problem with a high accuracy. The recognition time is, however, crucial in our case. Therefore, as major contribution, we aim at speeding up the recognition by a new instance selection technique. We describe this technique and discuss its theoretical background. In our experiments on publicly available real ECG-data, we empirically evaluate our approach and show that it outperforms a state-of-the-art instance selection technique.
更多查看译文
关键词
instance selection,abnormal ecg signal,recognition time,state-of-the-art instance selection technique,ecg signal,automatic recognition,state-of-the-art solution,fast classification,abnormal part,electrocardiograph signals,new instance selection technique,simple case,automated recognition,scaling,accuracy,time series analysis,time series,heart
AI 理解论文
溯源树
样例
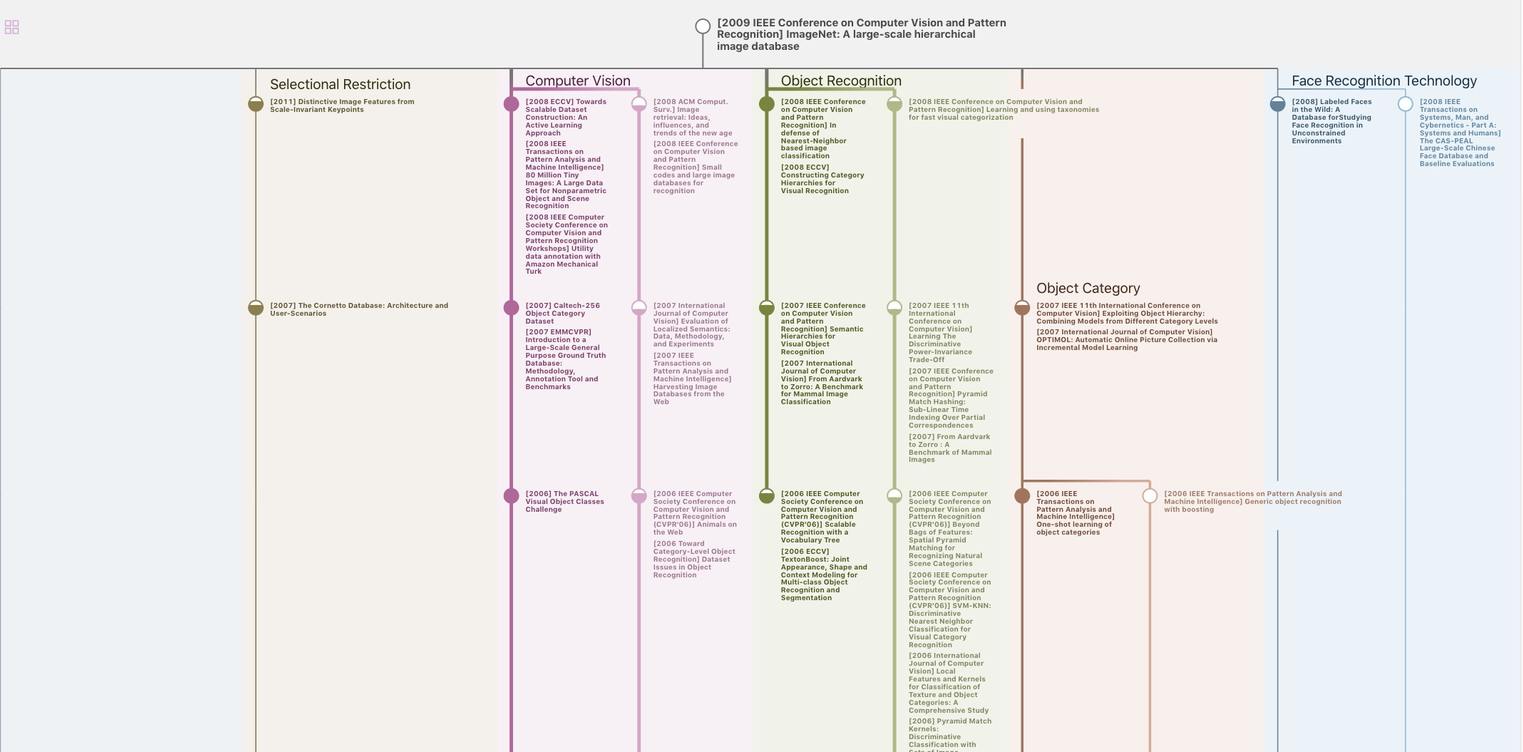
生成溯源树,研究论文发展脉络
Chat Paper
正在生成论文摘要