Joining distributed pattern processing and homeostatic plasticity in recurrent on-center off-surround shunting networks: noise, saturation, short-term memory, synaptic scaling, and BDNF.
Neural Networks(2012)
摘要
The activities of neurons vary within small intervals that are bounded both above and below, yet the inputs to these neurons may vary many-fold. How do networks of neurons process distributed input patterns effectively under these conditions? If a large number of input sources intermittently converge on a cell through time, then a serious design problem arises: if cell activities are sensitive to large inputs, then why do not small inputs get lost in internal system noise? If cell activities are sensitive to small inputs, then why do they not all saturate at their maximum values in response to large inputs and thereby become incapable of processing analog differences in inputs across an entire network? Grossberg (1973) solved this noise-saturation dilemma using neurons that obey the membrane, or shunting, equations of neurophysiology interacting in recurrent and non-recurrent on-center off-surround networks, and showed how different signal functions can influence the activity patterns that the network stores in short-term memory. These results demonstrated that maintaining a balance between excitation and inhibition in a neural network is essential to process distributed patterns of inputs and signals without experiencing the catastrophies of noise or saturation. However, shunting on-center off-surround networks only guarantee that cell activities remain sensitive to the relative sizes of inputs and recurrent signals, but not that they will use the full dynamic range that each cell can support. Additional homeostatic plasticity mechanisms are needed to anchor the activities of networks to exploit their full dynamic range. This article shows how mechanisms of synaptic scaling can be incorporated within recurrent on-center off-surround networks in such a way that their pattern processing capabilities, including the ability to make winner-take-all decisions, is preserved. This model generalizes the synaptic scaling model of van Rossum, Bi, & Turrigiano (2000) for a single cell to a pattern-processing network of shunting cells that is capable of short-term memory storage, including a representation of how BDNF may homeostatically scale the strengths of excitatory and inhibitory synapses in opposite directions.
更多查看译文
关键词
neural network,cell activity,full dynamic range,pattern processing,shunting cell,large input,small input,short-term memory,network store,recurrent on-center off-surround,entire network,homeostatic plasticity,synaptic scaling,single cell,neurons process,automatic gain control,anchoring,short term memory,winner take all
AI 理解论文
溯源树
样例
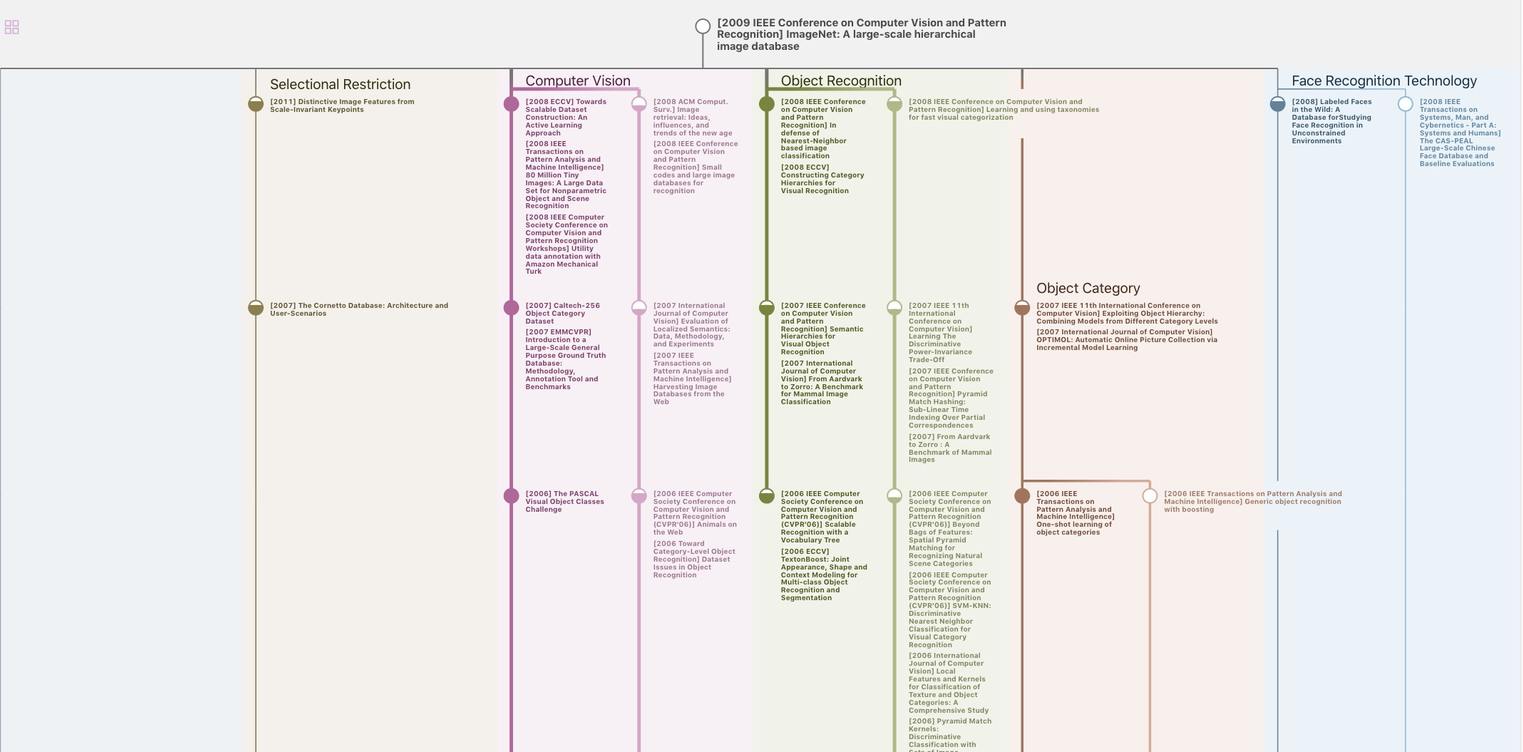
生成溯源树,研究论文发展脉络
Chat Paper
正在生成论文摘要