A hybrid dynamic multi-objective immune optimization algorithm using prediction strategy and improved differential evolution crossover operator
NEURAL INFORMATION PROCESSING, PT II(2011)
摘要
In this paper, a hybrid dynamic multi-objective immune optimization algorithm is proposed. In the algorithm, when a change in the objective space is detected, aiming to improve the ability of responding to the environment change, a forecasting model, which is established by the non-dominated antibodies in previous optimum locations, is used to generate the initial antibodies population. Moreover, in order to speed up convergence, an improved differential evolution crossover with two selection strategies is proposed. Experimental results indicate that the proposed algorithm is promising for dynamic multi-objective optimization problems.
更多查看译文
关键词
environment change,dynamic multi-objective immune optimization,improved differential evolution crossover,dynamic multi-objective optimization problem,objective space,initial antibodies population,prediction strategy,non-dominated antibody,forecasting model,proposed algorithm,differential evolution
AI 理解论文
溯源树
样例
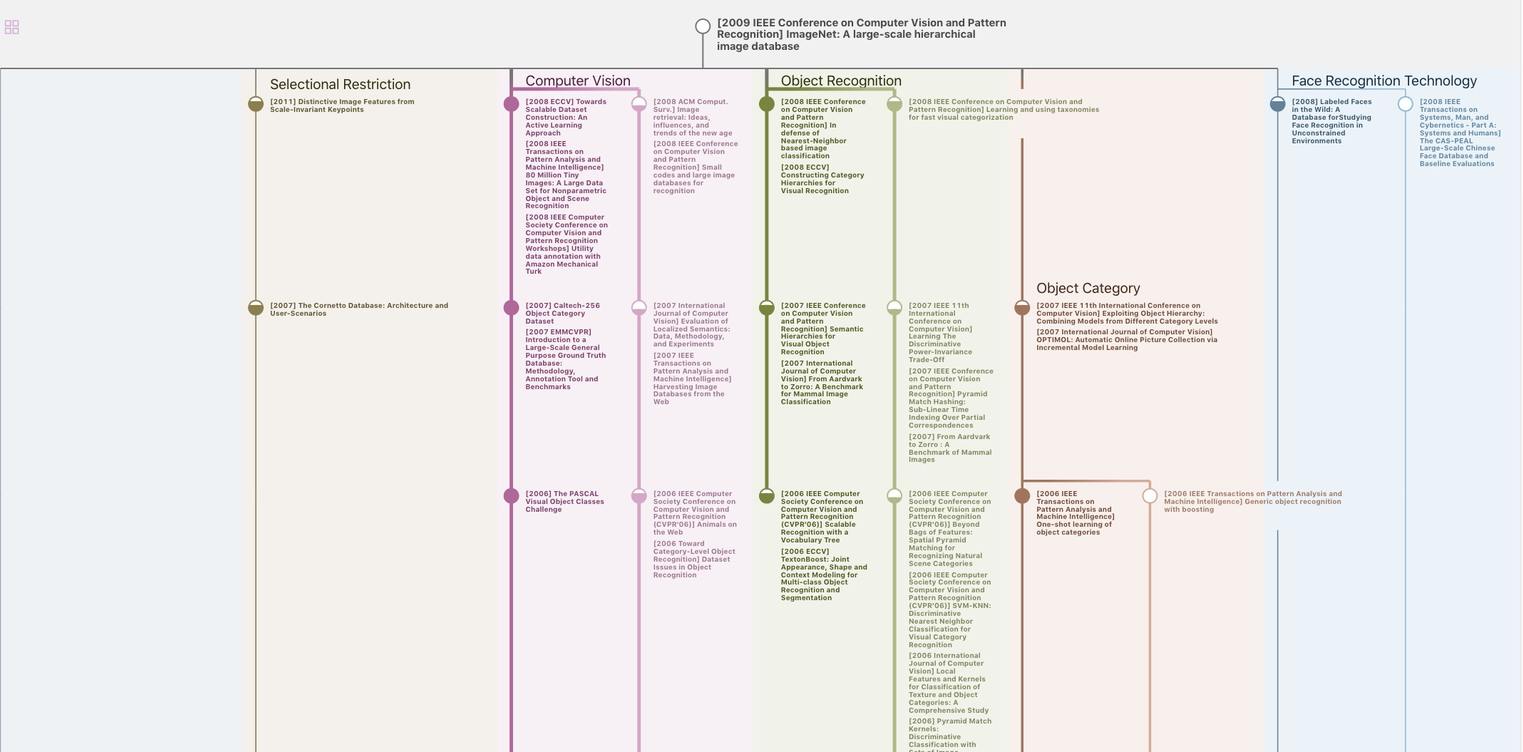
生成溯源树,研究论文发展脉络
Chat Paper
正在生成论文摘要