Imitating Inscrutable Enemies: Learning from Stochastic Policy Observation, Retrieval and Reuse
CASE-BASED REASONING RESEARCH AND DEVELOPMENT, 18TH INTERNATIONAL CONFERENCE ON CASE-BASED REASONING, ICCBR 2010(2010)
摘要
In this paper we study the topic of CBR systems learning from observations in which those observations can be represented as stochastic policies. We describe a general framework which encompasses three steps: (1) it observes agents performing actions, elicits stochastic policies representing the agents’ strategies and retains these policies as cases. (2) The agent analyzes the environment and retrieves a suitable stochastic policy. (3) The agent then executes the retrieved stochastic policy, which results in the agent mimicking the previously observed agent. We implement our framework in a system called JuKeCB that observes and mimics players playing games. We present the results of three sets of experiments designed to evaluate our framework. The first experiment demonstrates that JuKeCB performs well when trained against a variety of fixed strategy opponents. The second experiment demonstrates that JuKeCB can also, after training, win against an opponent with a dynamic strategy. The final experiment demonstrates that JuKeCB can win against "new" opponents (i.e. opponents against which JuKeCB is untrained).
更多查看译文
关键词
learning from observation,case capture and reuse,policy
AI 理解论文
溯源树
样例
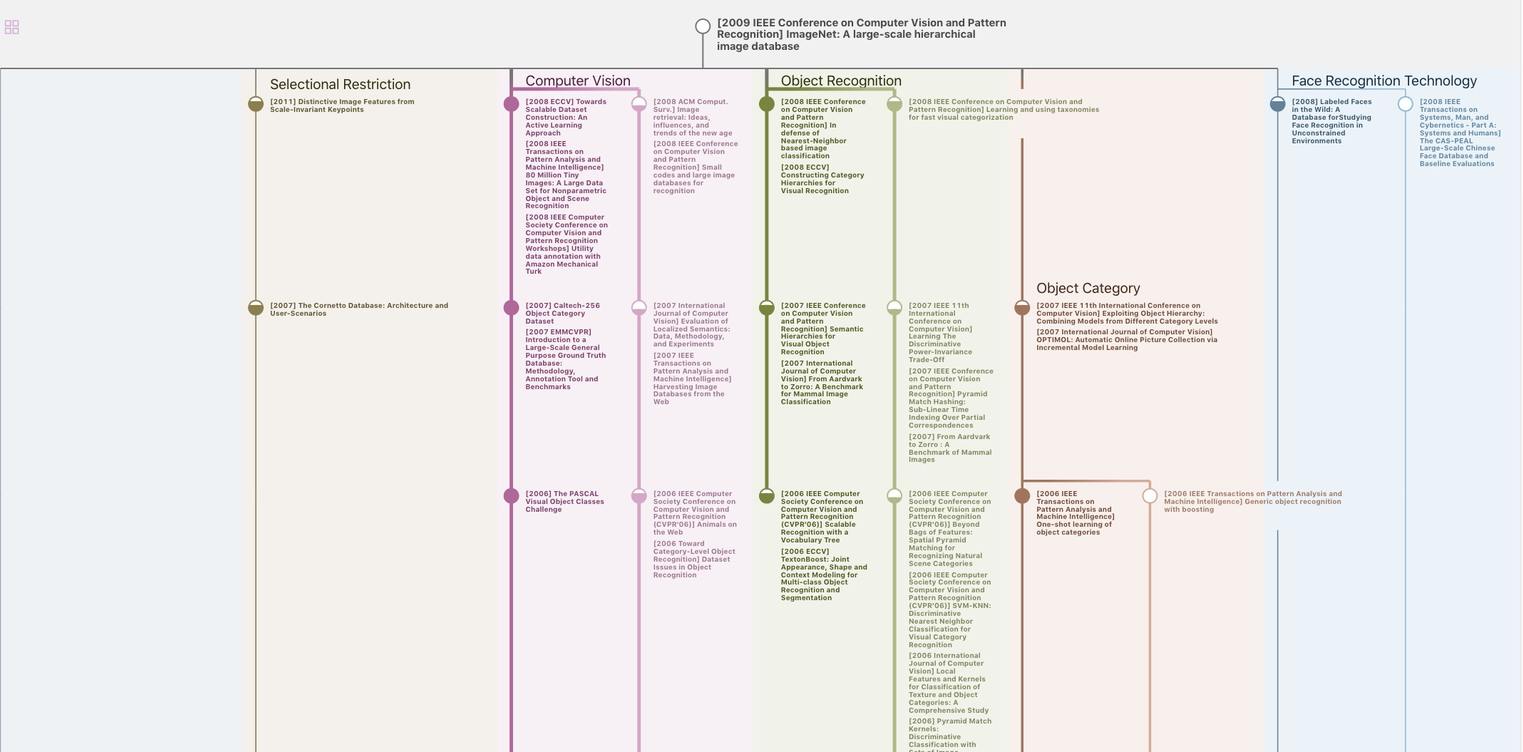
生成溯源树,研究论文发展脉络
Chat Paper
正在生成论文摘要