Automated Recognition of Robotic Manipulation Failures in High-throughput Biodosimetry Tool.
EXPERT SYSTEMS WITH APPLICATIONS(2012)
摘要
A completely automated, high-throughput biodosimetry workstation has been developed by the Center for Minimally Invasive Radiation Biodosimetry at Columbia University over the past few years. To process patients' blood samples safely and reliably presents a significant challenge in the development of this biodosimetry tool. In this paper, automated failure recognition methods of robotic manipulation of capillary tubes based on a torque/force sensor are described. The characteristic features of sampled raw signals are extracted through data preprocessing. The twelve-dimensional (12D) feature space is projected onto a two-dimensional (2D) feature plane by the optimized Principal Component Analysis (PCA) and Fisher Discrimination Analysis (FDA) feature extraction functions. For the three-class manipulation failure problem in the cell harvesting module, FDA yields better separability index than that of PCA and produces well separated classes. Three classification methods, Support Vector Machine (SVM), Fisher Linear Discrimination (FLD) and Quadratic Discrimination Analysis (QDA), are employed for real-time recognition. Considering the trade-off between error rate and computation cost, SVM achieves the best overall performance.
更多查看译文
关键词
feature plane,fisher discrimination analysis,high-throughput biodosimetry tool,quadratic discrimination analysis,fisher linear discrimination,feature extraction function,characteristic feature,automated recognition,fda yield,automated failure recognition method,component analysis,feature space,robotic manipulation failure,bioinformatics,feature extraction,classification,biomedical research
AI 理解论文
溯源树
样例
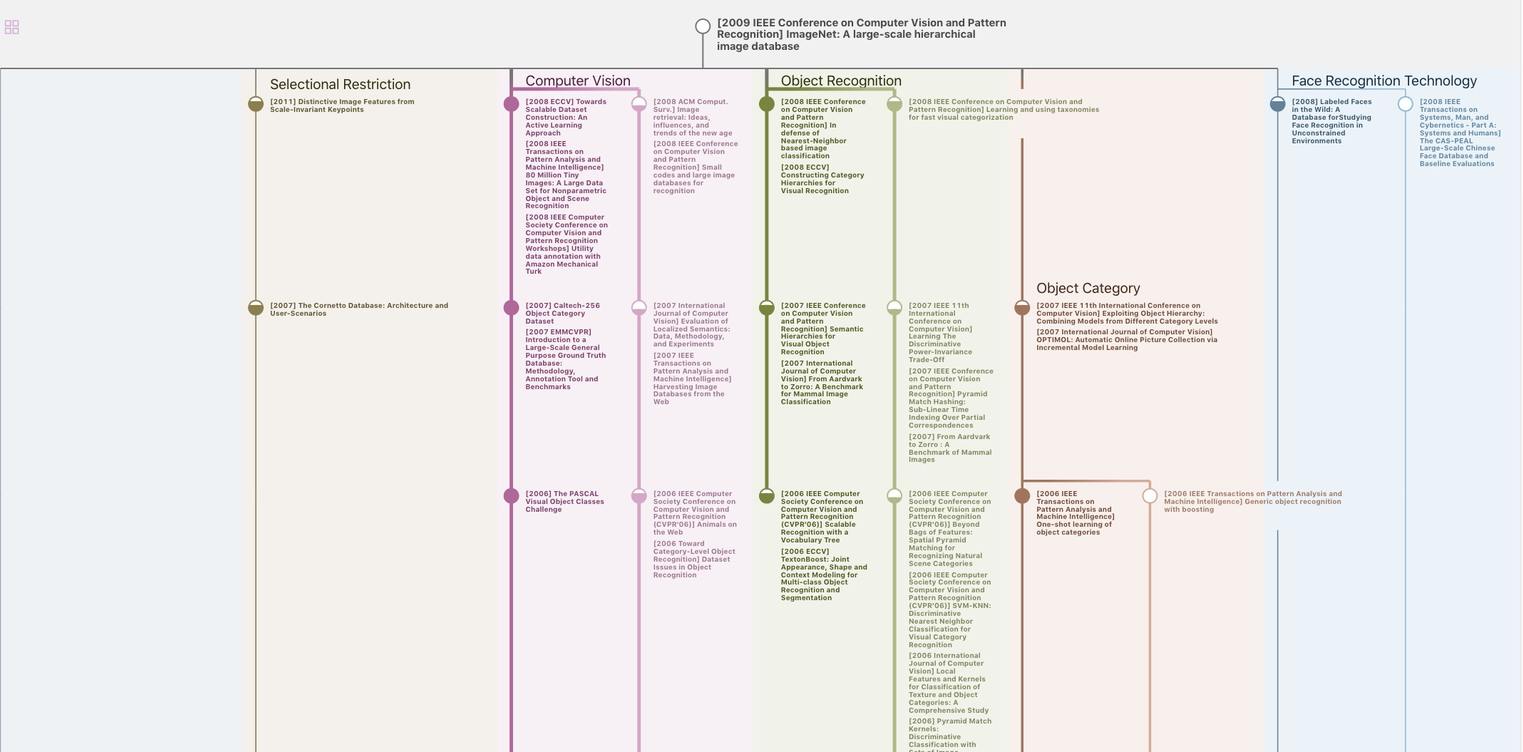
生成溯源树,研究论文发展脉络
Chat Paper
正在生成论文摘要