ProClusEnsem
Periodicals(2012)
摘要
AbstractKnowing the type of an uncharacterized membrane protein often provides a useful clue in both basic research and drug discovery. With the explosion of protein sequences generated in the post genomic era, determination of membrane protein types by experimental methods is expensive and time consuming. It therefore becomes important to develop an automated method to find the possible types of membrane proteins. In view of this, various computational membrane protein prediction methods have been proposed. They extract protein feature vectors, such as PseAAC (pseudo amino acid composition) and PsePSSM (pseudo position-specific scoring matrix) for representation of protein sequence, and then learn a distance metric for the KNN (K nearest neighbor) or NN (nearest neighbor) classifier to predicate the final type. Most of the metrics are learned using linear dimensionality reduction algorithms like Principle Components Analysis (PCA) and Linear Discriminant Analysis (LDA). Such metrics are common to all the proteins in the dataset. In fact, they assume that the proteins lie on a uniform distribution, which can be captured by the linear dimensionality reduction algorithm. We doubt this assumption, and learn local metrics which are optimized for local subset of the whole proteins. The learning procedure is iterated with the protein clustering. Then a novel ensemble distance metric is given by combining the local metrics through Tikhonov regularization. The experimental results on a benchmark dataset demonstrate the feasibility and effectiveness of the proposed algorithm named ProClusEnsem.
更多查看译文
关键词
Clustering,Distance metric,Linear dimensionality reduction,Membrane protein types prediction,Pseudo amino acid composition,Tikhonov regularization
AI 理解论文
溯源树
样例
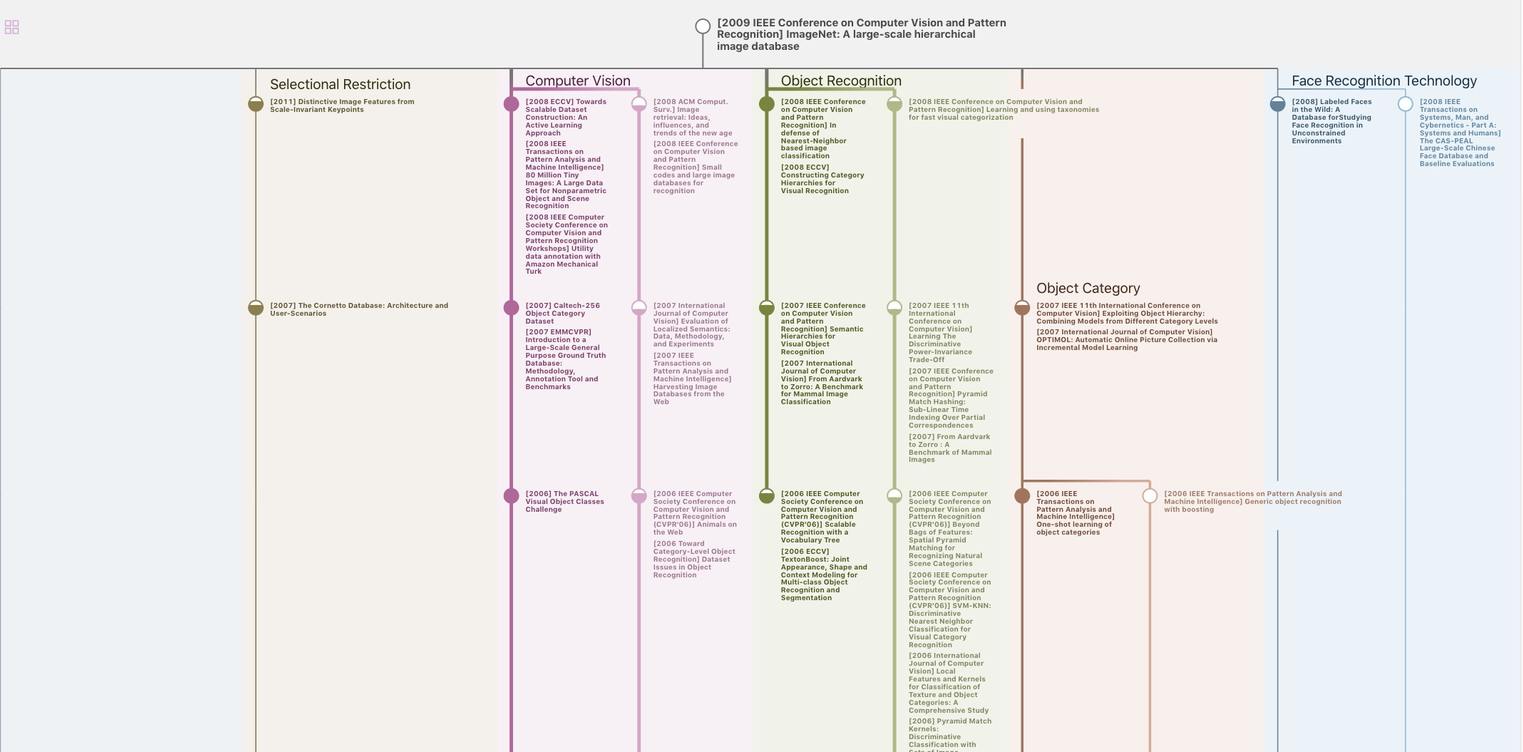
生成溯源树,研究论文发展脉络
Chat Paper
正在生成论文摘要