A new hybrid clustering method for reducing very large spatio-temporal dataset
ADVANCED DATA MINING AND APPLICATIONS, PT I(2011)
摘要
Spatio-temporal datasets are often very large and difficult to analyse. Recently a lot of interest has arisen towards data-mining techniques to reduce very large spatio-temporal datasets into relevant subsets as well as to help visualisation tools to effectively display the results. Cluster-based mining methods have proven to be successful at reducing the large size of raw data by extracting useful knowledge as representatives. As a consequence, instead of dealing with a large size of raw data, we can use these representatives to visualise or to analyse the data without losing important information. In this paper, we present a new hybrid approach for reducing large spatio-temporal datasets. This approach is based on the combination of density-based and graph-based clustering. Drawing on the Shared Nearest Neighbour concept, it applies the Euclidean metric distance to determine the nearest neighbour similarity. We also present and discuss the evaluation of the results for this approach.
更多查看译文
关键词
new hybrid clustering method,cluster-based mining method,graph-based clustering,new hybrid approach,large size,euclidean metric distance,shared nearest neighbour concept,spatio-temporal datasets,raw data,data-mining technique,large spatio-temporal datasets,data reduction
AI 理解论文
溯源树
样例
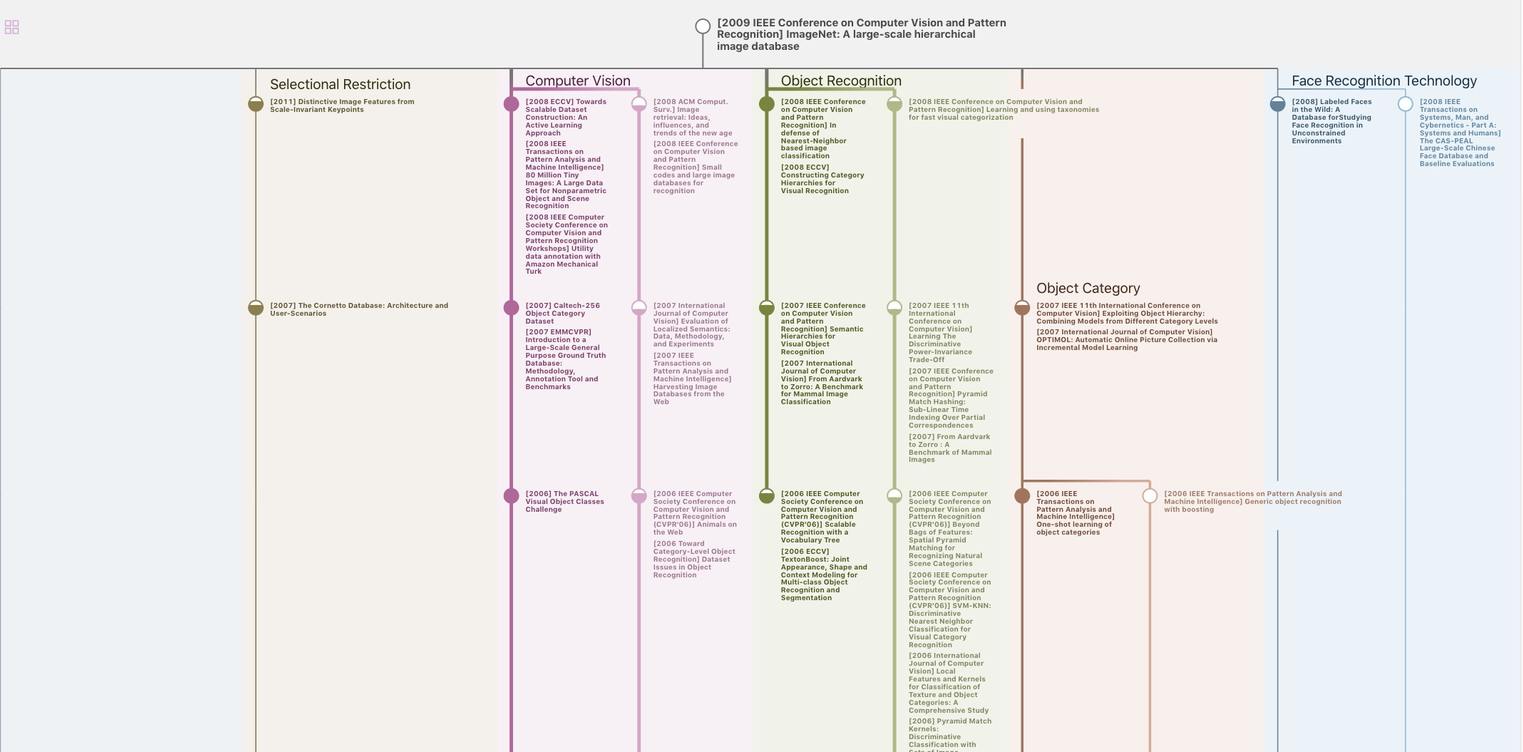
生成溯源树,研究论文发展脉络
Chat Paper
正在生成论文摘要