Nonlinear channel equalization for QAM signal constellation using artificial neural networks.
IEEE Transactions on Systems, Man, and Cybernetics, Part B: Cybernetics(1999)
摘要
Application of artificial neural networks (ANN's) to adaptive channel equalization in a digital communication system with 4-QAM signal constellation is reported in this paper. A novel computationally efficient single layer functional link ANN (FLANN) is proposed for this purpose. This network has a simple structure in which the nonlinearity is introduced by functional expansion of the input pattern by trigonometric polynomials. Because of input pattern enhancement, the FLANN is capable of forming arbitrarily nonlinear decision boundaries and can perform complex pattern classification tasks. Considering channel equalization as a nonlinear classification problem, the FLANN has been utilized for nonlinear channel equalization. The performance of the FLANN is compared with two other ANN structures [a multilayer perceptron (MLP) and a polynomial perceptron network (PPN)] along with a conventional linear LMS-based equalizer for different linear and nonlinear channel models. The effect of eigenvalue ratio (EVR) of input correlation matrix on the equalizer performance has been studied. The comparison of computational complexity involved for the three ANN structures is also provided.
更多查看译文
关键词
ANN structure,channel equalization,nonlinear channel equalization,nonlinear channel model,nonlinear classification problem,nonlinear decision boundary,complex pattern classification task,input correlation matrix,input pattern,input pattern enhancement,Nonlinear channel equalization,QAM signal constellation usingartificial,neural network
AI 理解论文
溯源树
样例
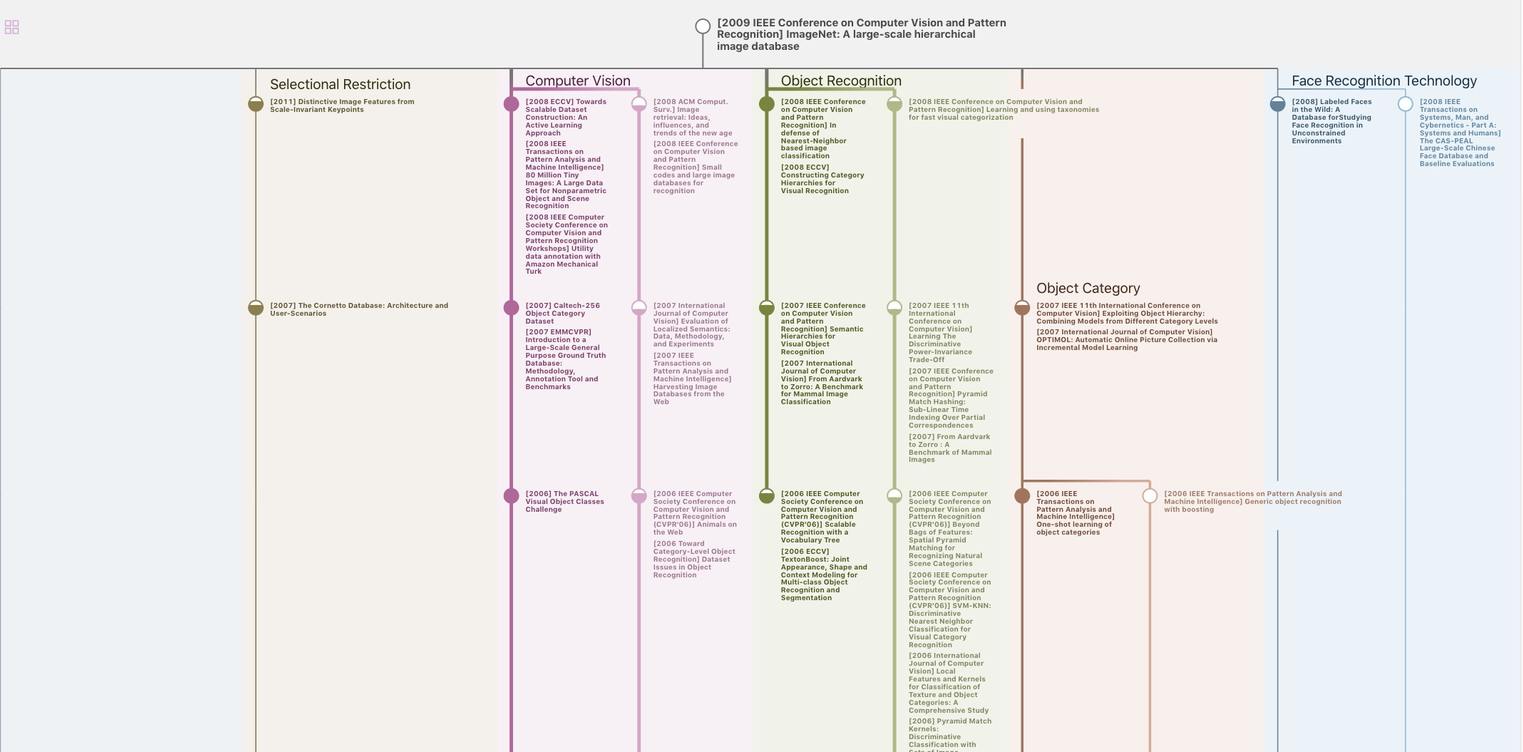
生成溯源树,研究论文发展脉络
Chat Paper
正在生成论文摘要