Gradient based algorithms with loss functions and kernels for improved on-policy control
EWRL(2012)
摘要
We introduce and empirically evaluate two novel online gradient-based reinforcement learning algorithms with function approximation --- one model based, and the other model free. These algorithms come with the possibility of having non-squared loss functions which is novel in reinforcement learning, and seems to come with empirical advantages. We further extend a previous gradient based algorithm to the case of full control, by using generalized policy iteration. Theoretical properties of these algorithms are studied in a companion paper.
更多查看译文
关键词
companion paper,function approximation,empirical advantage,generalized policy iteration,novel online gradient-based reinforcement,improved on-policy control,previous gradient,theoretical property,reinforcement learning,non-squared loss function,full control
AI 理解论文
溯源树
样例
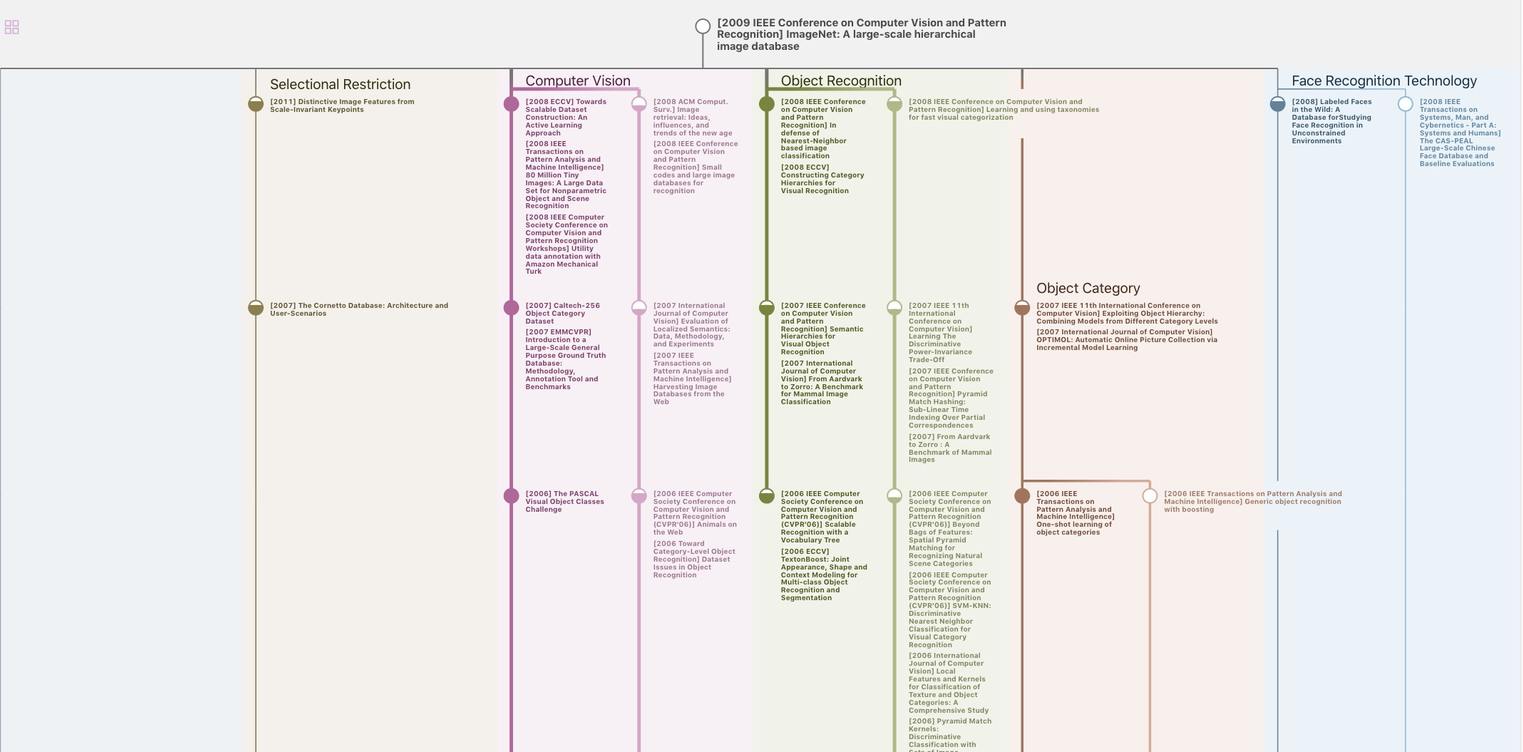
生成溯源树,研究论文发展脉络
Chat Paper
正在生成论文摘要