A Novel Approach for Raising the Accuracy of SVDD
Computing, Measurement, Control and Sensor Network(2012)
摘要
This paper presents a novel one-class classification method to improve the correct proportion by adding new outliers. General speaking, it is difficult to improve the correct proportion of the one-class classification because of few data. How to distinguish the target objects correctly from unknown samples becomes one of the most important problems. The support vector data description (SVDD) is a method proposed to solve the problem of one-class classification. It models a hyper sphere around the target set, and by the introduction of kernel functions, more flexible descriptions are obtained. In SVDD, the width parameter and the penalty parameter have to be given beforehand by the user. To automatically optimize the values for these parameters, the error on both the target and outlier data has to be estimated. Because no outlier examples are available, we propose a max-min range method for generating artificial outliers in this paper. By generating artificial outliers around the target set, the accuracy of classifiers will improve. At the last, we use four benchmark data sets: Iris, Wine, Balance-scale, and Ionosphere data base to validate the approach in this research indeed has better classification result.
更多查看译文
关键词
balance-scale database,one-class classification,error estimation,width parameter,max-min range method,ionosphere data base,pattern classification,correct proportion,one-class classification method,better classification result,support vector data description (svdd),benchmark data set,minimax techniques,hypersphere,wine database,iris database,target set,novel one-class classification method,svdd,ionosphere database,support vector data description,penalty parameter,artificial outlier,novel approach,outlier data,support vector machines,target object,kernel function,machine learning,one class classification
AI 理解论文
溯源树
样例
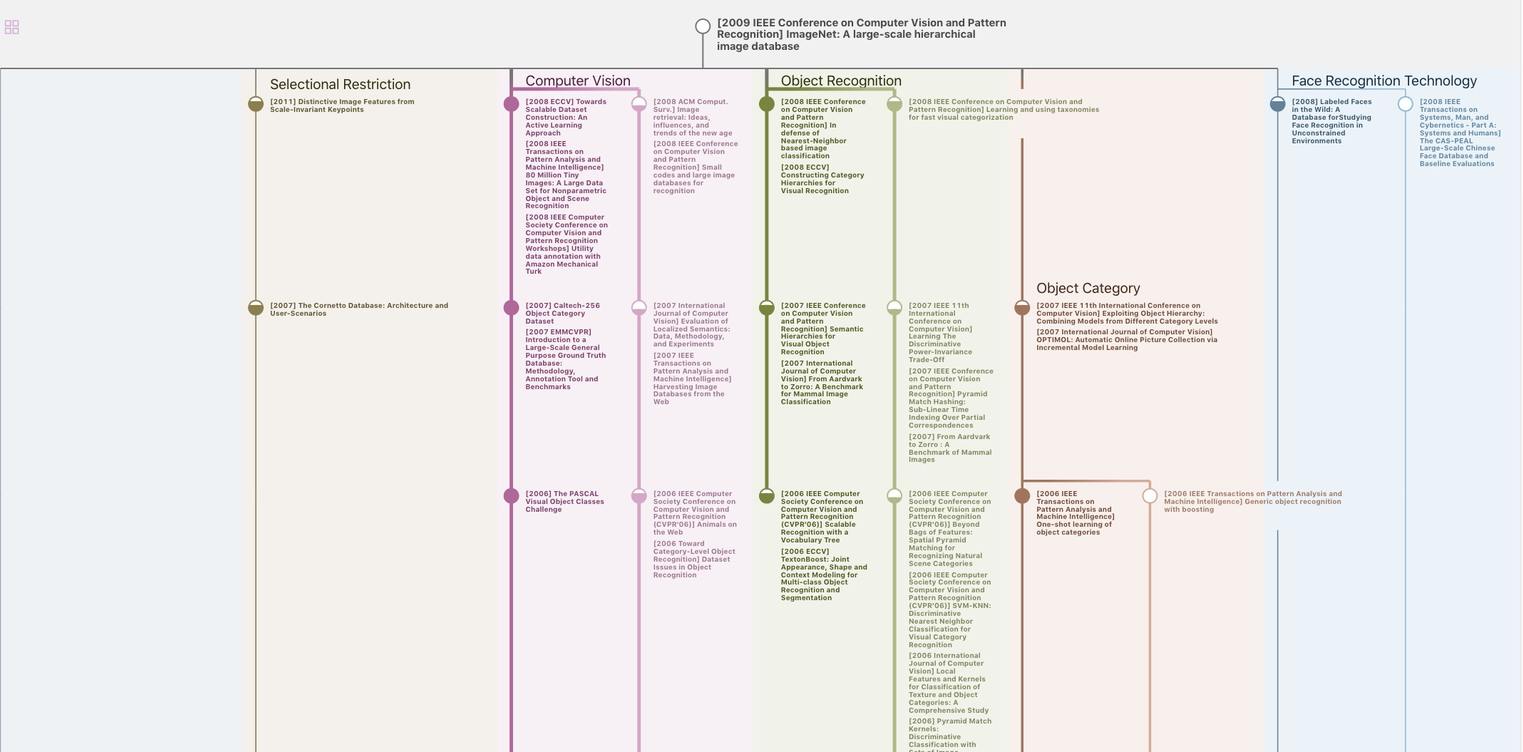
生成溯源树,研究论文发展脉络
Chat Paper
正在生成论文摘要