Revenue forecasting using a least-squares support vector regression model in a fuzzy environment
Information Sciences(2013)
摘要
Revenue forecasting is difficult but essential for companies that want to create high-quality revenue budgets, especially in an uncertain economic environment with changing government policies. Under these conditions, the subjective judgment of decision makers is a crucial factor in making accurate forecasts. This investigation develops a fuzzy least-squares support vector regression model with genetic algorithms (FLSSVRGA) to forecast seasonal revenues. The FLSSVRGA uses the H-level to control the possibility distribution range yielded by the fuzzy model and to provide the fuzzy prediction interval. Depending on various factors, such as the global economy and government policies, a decision maker can elect a different level for H using the FLSSVRGA. The proposed FLSSVRGA model is a rolling forecasting model with time series data updated monthly that predicts revenue for the coming month. Four other forecasting models: the seasonal autoregressive integrated moving average (SARIMA), generalized regression neural networks (GRNN), support vector regression with genetic algorithms (SVRGA) and least-squares support vector regression with genetic algorithms (LSSVRGA), are employed to forecast the same data sets. The experimental results indicate that the FLSSVRGA model outperforms all four models in terms of forecasting accuracy. Thus, the FLSSVRGA model is a useful alternative for forecasting seasonal time series data in an uncertain environment; it can provide a user-defined fuzzy prediction interval for decision makers.
更多查看译文
关键词
Least-squares support vector regression,Membership function,Genetic algorithms,Revenue forecasting
AI 理解论文
溯源树
样例
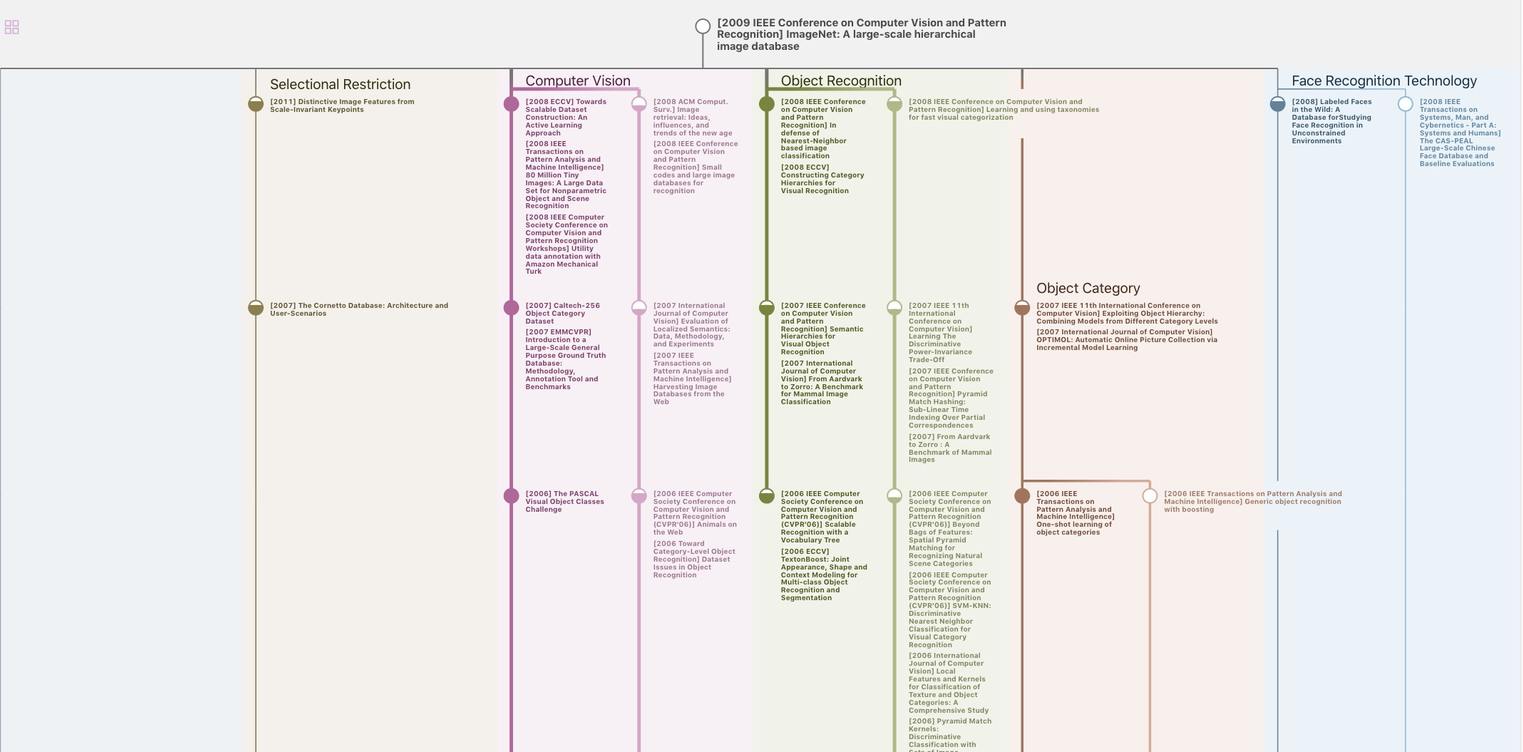
生成溯源树,研究论文发展脉络
Chat Paper
正在生成论文摘要