Towards an automated 3d reconstruction of plant architecture
AGTIVE(2011)
摘要
Non-destructive and quantitative analysis and screening of plant phenotypes throughout plants' lifecycles is essential to enable greater efficiency in crop breeding and to optimize decision making in crop management. In this contribution we propose graph grammars within a sensor-based system approach to the automated 3D reconstruction and semantic annotation of plant architectures. The plant architectures in turn will serve for reliable plant phenotyping. More specifically, we propose to employ Relational Growth Grammars to derive semantically annotated 3D reconstruction hypotheses of plant architectures from 3D sensor data, i.e., laser range measurements. Furthermore, we suggest deriving optimal reconstruction hypotheses by embedding the graph grammar-based data interpretation within a sophisticated probabilistic optimization framework, namely a Reversible Jump Markov Chain Monte Carlo sampling. This paper presents the design of the overall system framework with the graph grammar-based data interpretation as the central component. Furthermore, we present first system improvements and experimental results achieved in the application domain of grapevine breeding.
更多查看译文
关键词
sensor-based system approach,reliable plant phenotyping,optimal reconstruction hypothesis,graph grammar,graph grammar-based data interpretation,sensor data,overall system framework,plant architecture,reconstruction hypothesis,plant phenotypes
AI 理解论文
溯源树
样例
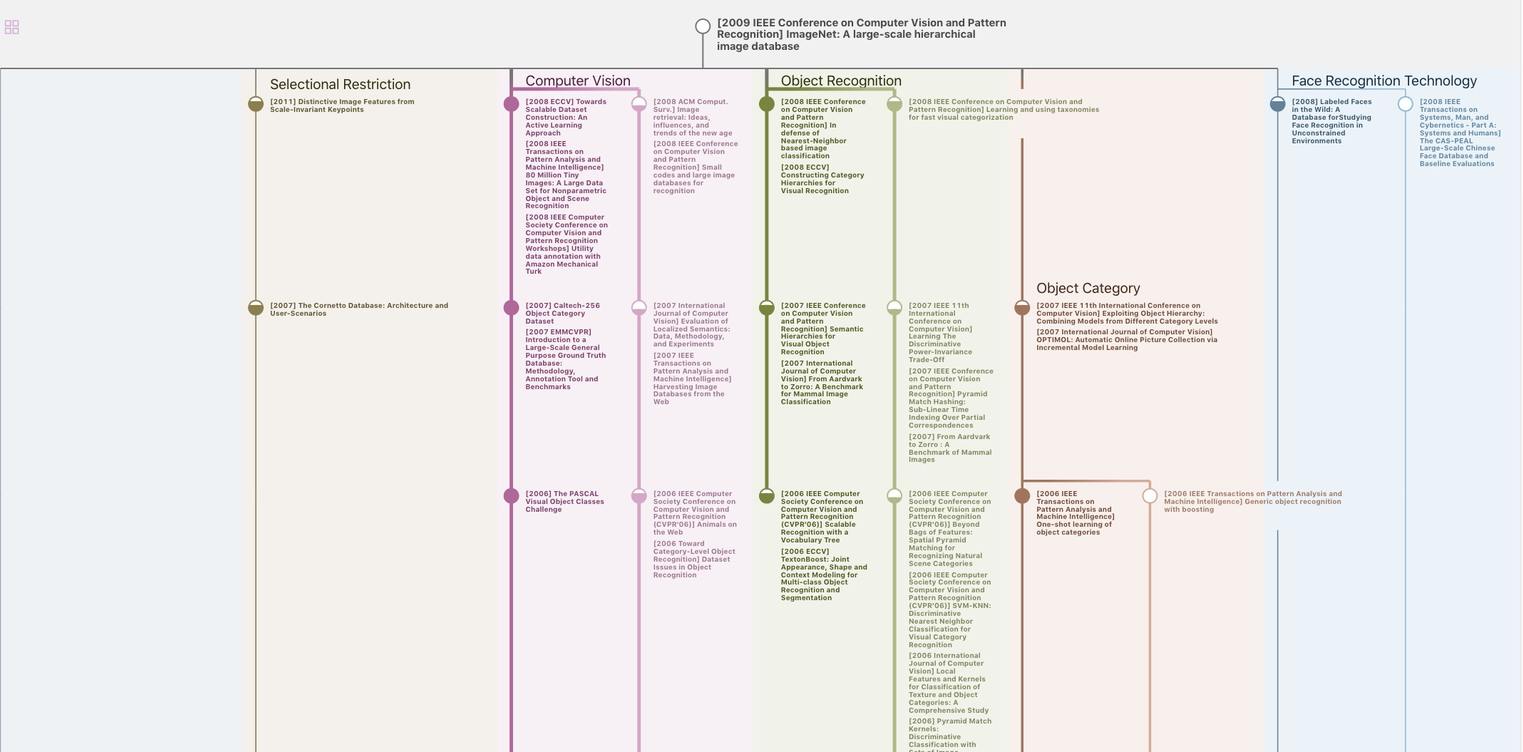
生成溯源树,研究论文发展脉络
Chat Paper
正在生成论文摘要