Bayesian Network Classifiers with Reduced Precision Parameters.
ECML/PKDD (1)(2012)
摘要
Bayesian network classifiers (BNCs) are probabilistic classifiers showing good performance in many applications. They consist of a directed acyclic graph and a set of conditional probabilities associated with the nodes of the graph. These conditional probabilities are also referred to as parameters of the BNCs. According to common belief, these classifiers are insensitive to deviations of the conditional probabilities under certain conditions. The first condition is that these probabilities are not too extreme, i.e. not too close to 0 or 1. The second is that the posterior over the classes is significantly different. In this paper, we investigate the effect of precision reduction of the parameters on the classification performance of BNCs. The probabilities are either determined generatively or discriminatively. Discriminative probabilities are typically more extreme. However, our results indicate that BNCs with discriminatively optimized parameters are almost as robust to precision reduction as BNCs with generatively optimized parameters. Furthermore, even large precision reduction does not decrease classification performance significantly. Our results allow the implementation of BNCs with less computational complexity. This supports application in embedded systems using floating-point numbers with small bit-width. Reduced bit-widths further enable to represent BNCs in the integer domain while maintaining the classification performance.
更多查看译文
关键词
classification performance,conditional probability,precision reduction,good performance,large precision reduction,acyclic graph,discriminatively optimized parameter,generatively optimized parameter,Bayesian network classifier,Reduced bit-widths,reduced precision parameter
AI 理解论文
溯源树
样例
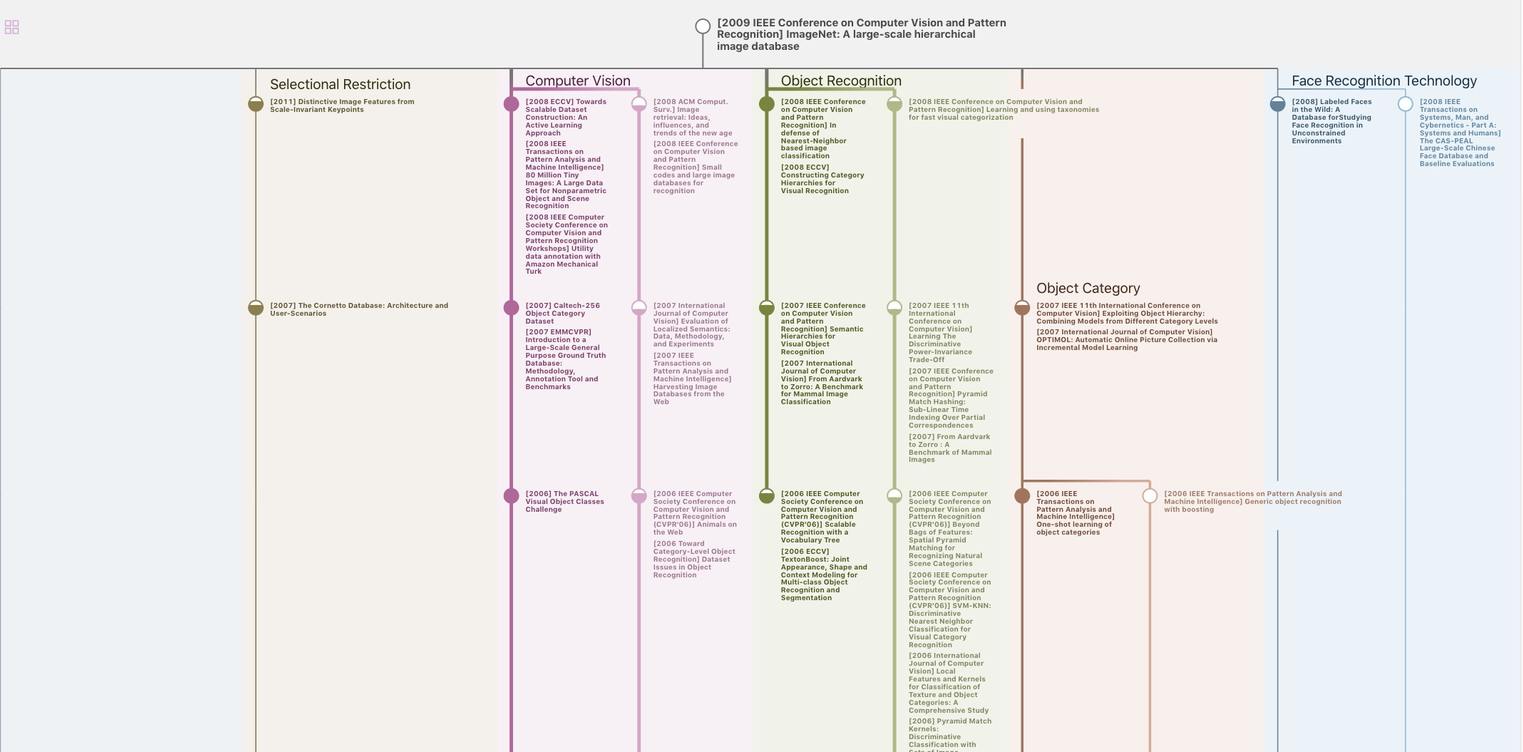
生成溯源树,研究论文发展脉络
Chat Paper
正在生成论文摘要