Hypergraph learning with hyperedge expansion
ECML/PKDD (1)(2012)
摘要
We propose a new formulation called hyperedge expansion (HE) for hypergraph learning. The HE expansion transforms the hypergraph into a directed graph on the hyperedge level. Compared to the existing works (e.g. star expansion or normalized hypergraph cut), the learning results with HE expansion would be less sensitive to the vertex distribution among clusters, especially in the case that cluster sizes are unbalanced. Because of the special structure of the auxiliary directed graph, the linear eigenvalue problem of the Laplacian can be transformed into a quadratic eigenvalue problem, which has some special properties suitable for semi-supervised learning and clustering problems. We show in the experiments that the new algorithms based on the HE expansion achieves statistically significant gains in classification performance and good scalability for the co-occurrence data.
更多查看译文
关键词
hypergraph learning,new formulation,normalized hypergraph cut,star expansion,hyperedge level,linear eigenvalue problem,hyperedge expansion,new algorithm,semi-supervised learning,clustering problem
AI 理解论文
溯源树
样例
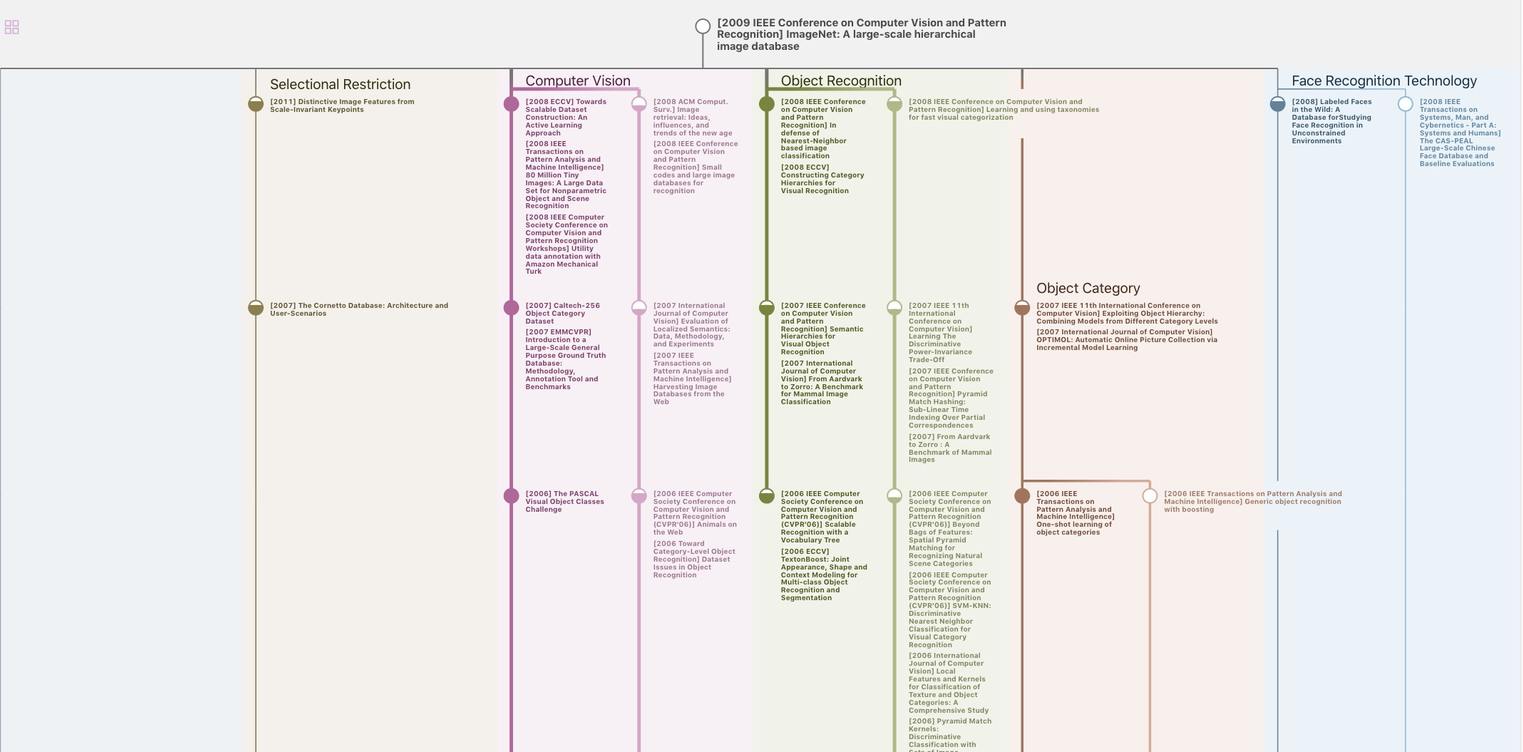
生成溯源树,研究论文发展脉络
Chat Paper
正在生成论文摘要