On graph-associated matrices and their eigenvalues for optical character recognition
ANNPR(2012)
摘要
In this paper, the classification power of the eigenvalues of six graph-associated matrices is investigated and evaluated on a benchmark dataset for optical character recognition. The extracted eigenvalues were utilized as feature vectors for multi-class classification using support vector machines. Each graph-associated matrix contains a certain type of geometric/spacial information, which may be important for the classification process. Classification results are presented for all six feature types, as well as for classifier combinations at decision level. For the decision level combination probabilistic output support vector machines have been applied. The eigenvalues of the weighted adjacency matrix provided the best classification rate of 89.9 %. Here, almost half of the misclassified letters are confusion pairs, such as I-L and N-Z. This classification performance can be increased by decision fusion, using the sum rule, to 92.4 %.
更多查看译文
关键词
graph-associated matrix,classification power,decision level combination probabilistic,multi-class classification,classification process,optical character recognition,decision fusion,classification result,best classification rate,decision level,classification performance,support vector machine,spectrum
AI 理解论文
溯源树
样例
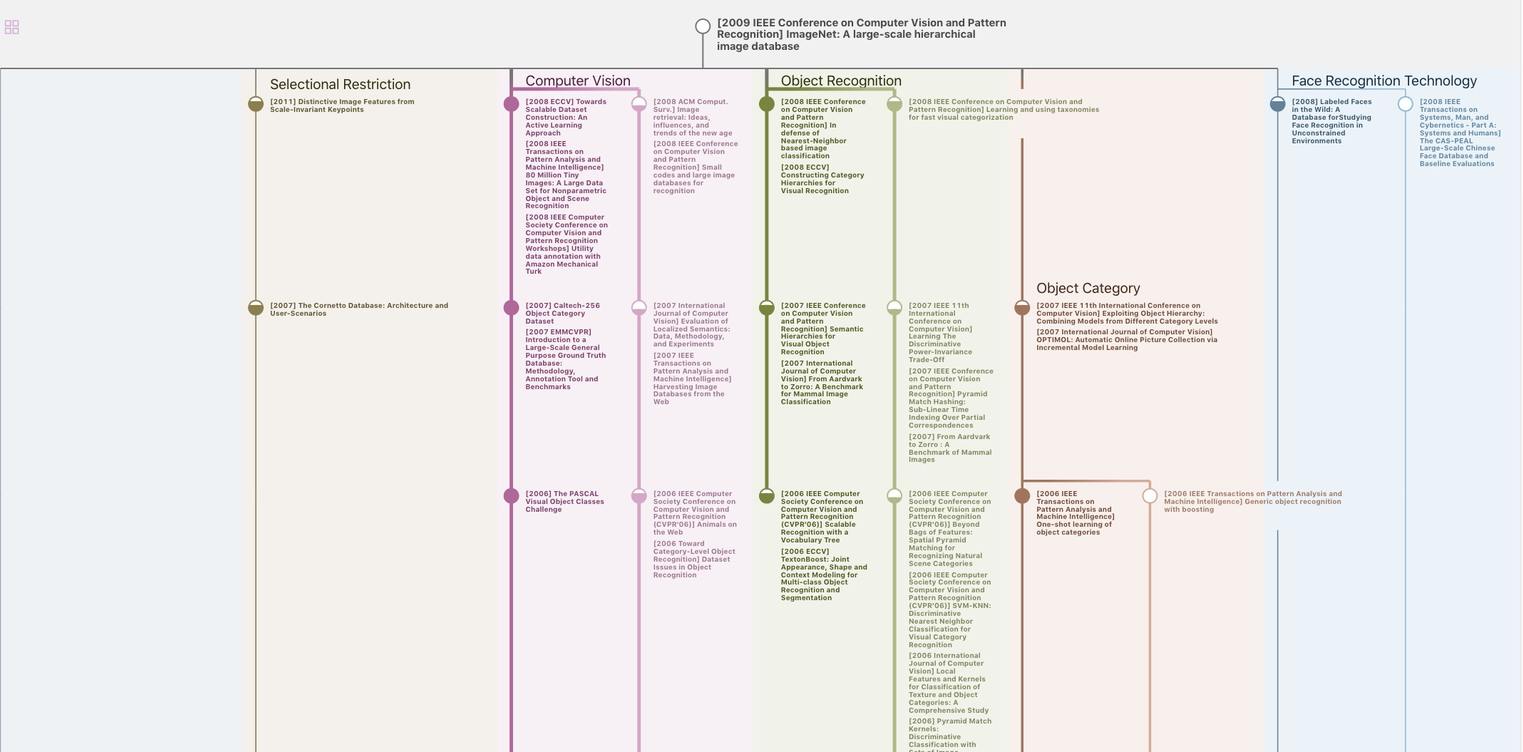
生成溯源树,研究论文发展脉络
Chat Paper
正在生成论文摘要