Multiple-index approach to multiple autoregressive time series model
STATISTICS AND COMPUTING(2011)
摘要
Time series which have more than one time dependent variable require building an appropriate model in which the variables not only have relationships with each other, but also depend on previous values in time. Based on developments for a sufficient dimension reduction, we investigate a new class of multiple time series models without parametric assumptions. First, for the dependent and independent time series, we simply use a univariate time series central subspace to estimate the autoregressive lags of the series. Secondly, we extract the successive directions to estimate the time series central subspace for regressors which include past lags of dependent and independent series in a mutual information multiple-index time series. Lastly, we estimate a multiple time series model for the reduced directions. In this article, we propose a unified estimation method of minimal dimension using an Akaike information criterion, for situations in which the dimension for multiple regressors is unknown. We present an analysis using real data from the housing price index showing that our approach is an alternative for multiple time series modeling. In addition, we check the accuracy for the multiple time series central subspace method using three simulated data sets.
更多查看译文
关键词
Multiple time series modeling,Sufficient dimension reduction,Time series central subspace,Nonlinearity
AI 理解论文
溯源树
样例
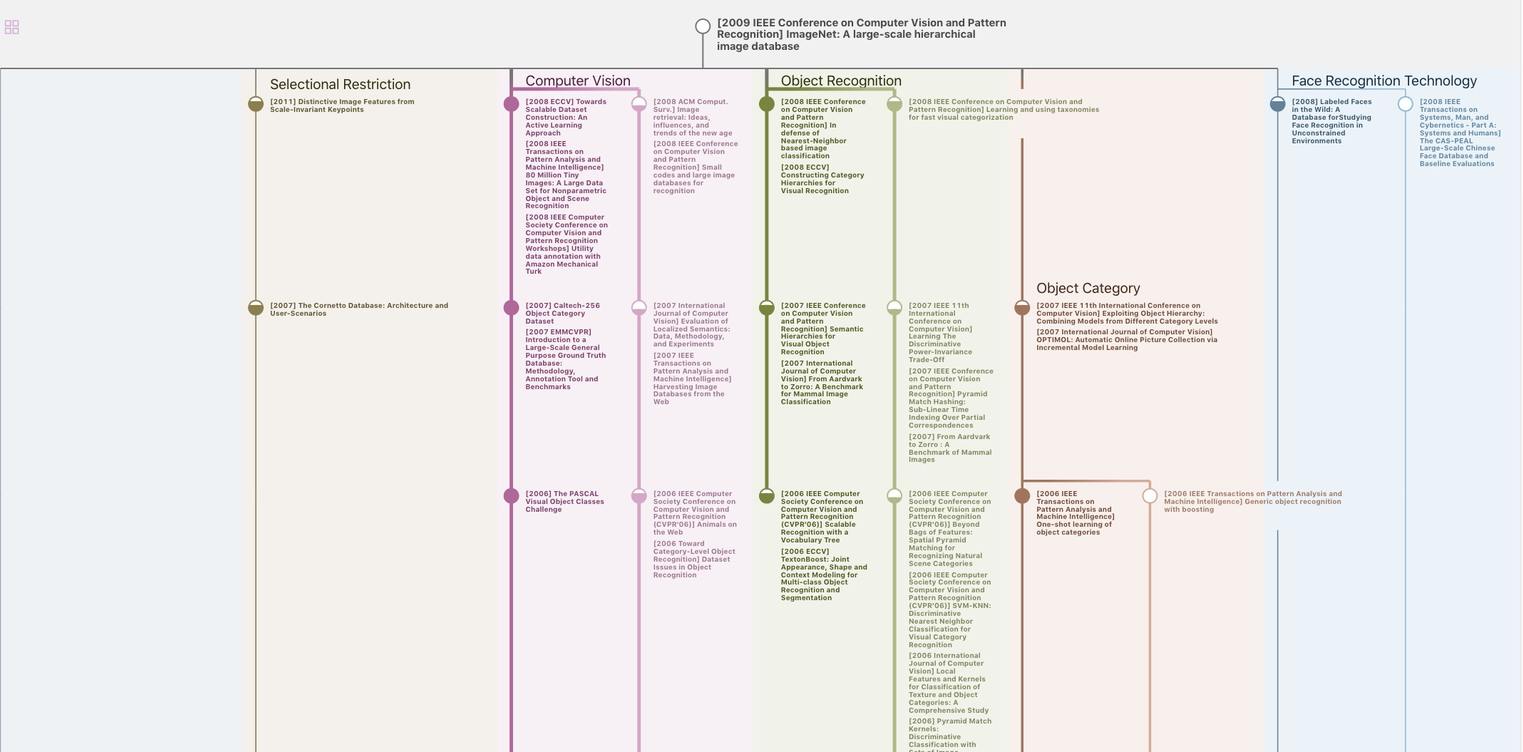
生成溯源树,研究论文发展脉络
Chat Paper
正在生成论文摘要