Preserving Privacy In Time Series Data Mining
DEVELOPMENTS IN DATA EXTRACTION, MANAGEMENT, AND ANALYSIS(2013)
摘要
Time series data mining poses new challenges to privacy. Through extensive experiments, the authors find that existing privacy-preserving techniques such as aggregation and adding random noise are insufficient due to privacy attacks such as data flow separation attack. This paper also presents a general model for publishing and mining time series data and its privacy issues. Based on the model, a spectrum of privacy preserving methods is proposed. For each method, effects on classification accuracy, aggregation error, and privacy leak are studied. Experiments are conducted to evaluate the performance of the methods. The results show that the methods can effectively preserve privacy without losing much classification accuracy and within a specified limit of aggregation error.
更多查看译文
关键词
general model,time series data mining,privacy issue,preserving privacy,privacy attack,classification accuracy,aggregation error,mining time series data,privacy leak,extensive experiment,data flow separation attack,privacy
AI 理解论文
溯源树
样例
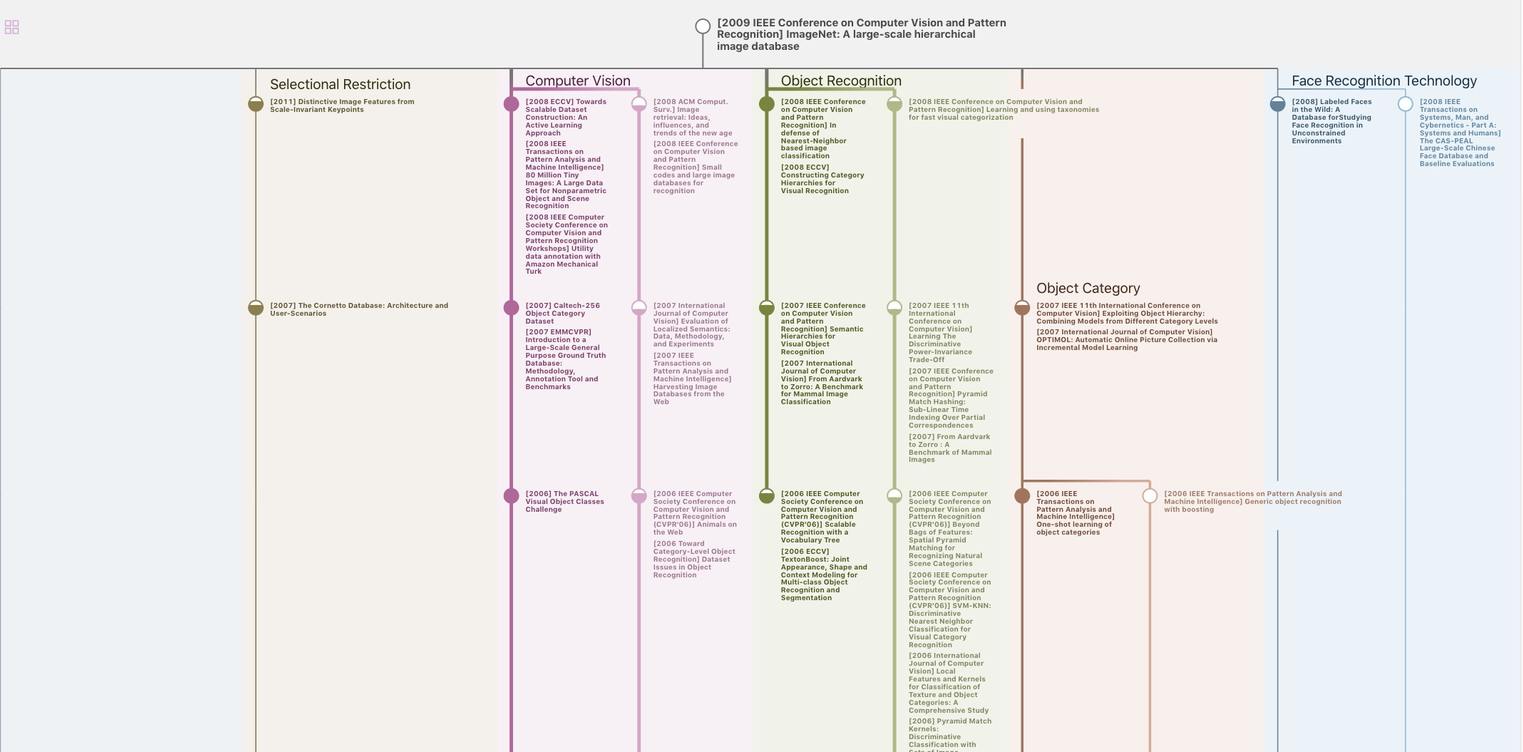
生成溯源树,研究论文发展脉络
Chat Paper
正在生成论文摘要