A preference multi-objective optimization based on adaptive rank clone and differential evolution
Natural Computing(2012)
摘要
Evolutionary multi-objective optimization (EMO) algorithms have been used in various real-world applications. However, most of the Pareto domination based multi-objective optimization evolutionary algorithms are not suitable for many-objective optimization. Recently, EMO algorithm incorporated decision maker’s preferences became a new trend for solving many-objective problems and showed a good performance. In this paper, we first use a new selection scheme and an adaptive rank based clone scheme to exploit the dynamic information of the online antibody population. Moreover, a special differential evolution (DE) scheme is combined with directional information by selecting parents for the DE calculation according to the ranks of individuals within a population. So the dominated solutions can learn the information of the non-dominated ones by using directional information. The proposed method has been extensively compared with two-archive algorithm, light beam search non-dominated sorting genetic algorithm II and preference rank immune memory clone selection algorithm over several benchmark multi-objective optimization problems with from two to ten objectives. The experimental results indicate that the proposed algorithm achieves competitive results.
更多查看译文
关键词
Preference multi-objective optimization,Artificial immune system,Differential evolution,Preference rank
AI 理解论文
溯源树
样例
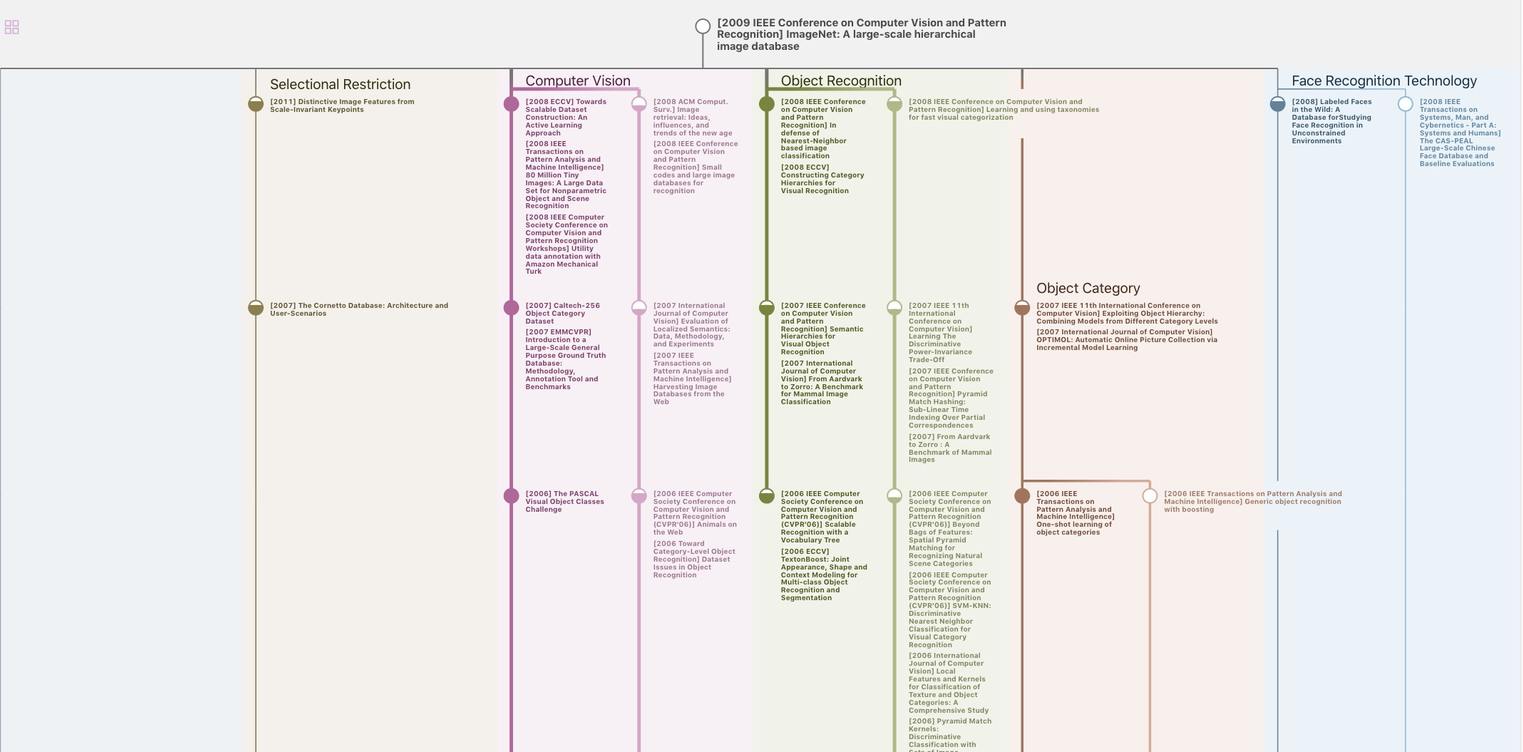
生成溯源树,研究论文发展脉络
Chat Paper
正在生成论文摘要