Modality classification for medical images using sparse coded affine-invariant descriptors
PAKDD Workshops(2012)
摘要
Modality is a key facet in medical image retrieval, as a user is likely interested in only one of e.g. radiology images, flowcharts, and pathology photos. While assessing image modality is trivial for humans, reliable automatic methods are required to deal with large un-annotated image bases, such as figures taken from the millions of scientific publications. We present a multi-disciplinary approach to tackle the classification problem by combining image features, meta-data, textual and referential information. We test our system's accuracy on the Image- CLEF 2011 medical modality classification data set. We show that using a fully affine-invariant feature descriptor and sparse coding on these descriptors in the Bag-of-Words image representation significantly increases the classification accuracy. Our best method achieves 87.89 and outperforms the state of the art.
更多查看译文
关键词
bag-of-words image representation,affine-invariant descriptors,medical modality classification data,large un-annotated image base,radiology image,image feature,classification accuracy,medical image retrieval,image modality,classification problem,affine-invariant feature descriptor,image classification,text mining,sparse coding
AI 理解论文
溯源树
样例
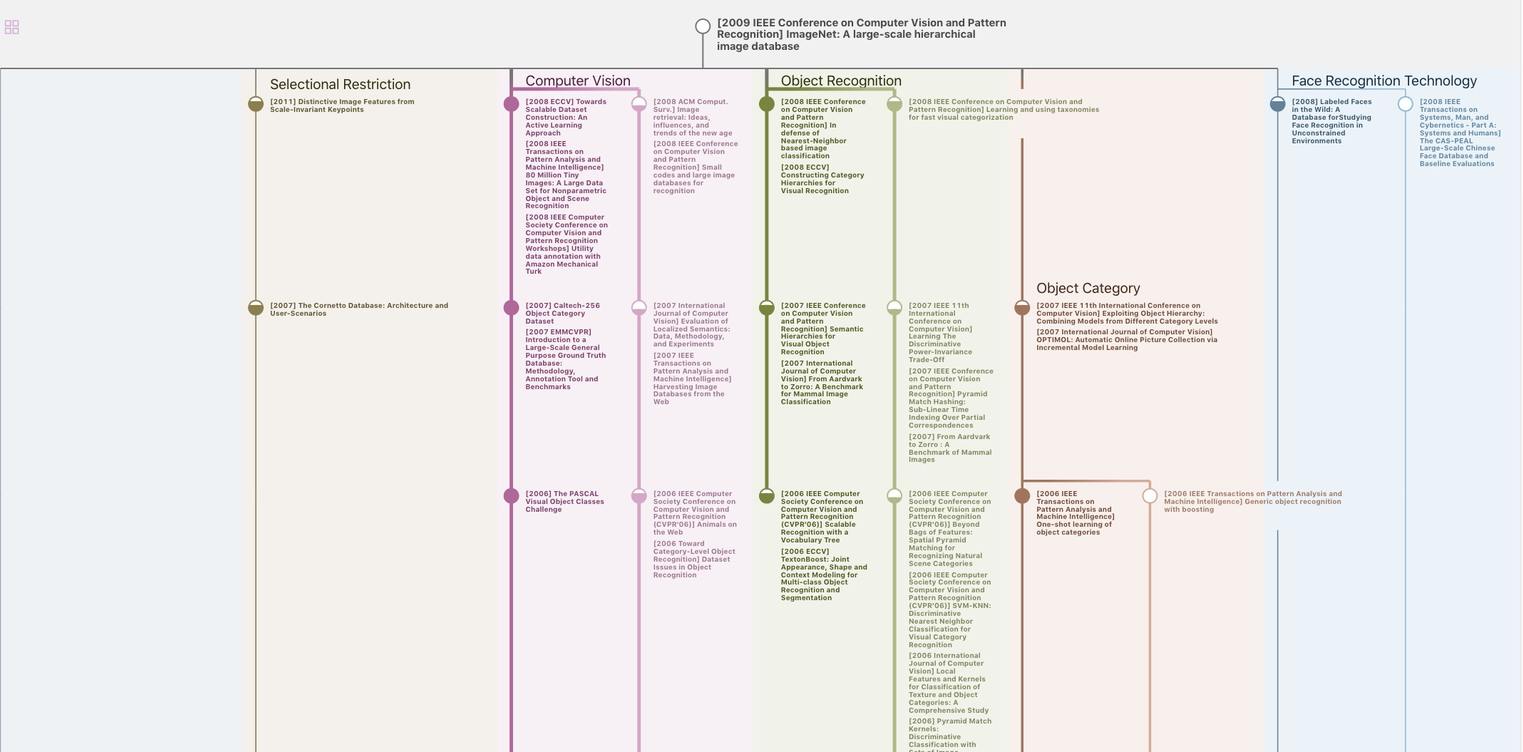
生成溯源树,研究论文发展脉络
Chat Paper
正在生成论文摘要