An Integrated Machine Learning and Case-Based Reasoning Approach to Answer Validation
ICMLA), 2012 11th International Conference(2012)
摘要
We propose a case-based reasoning (CBR) approach to answer validation/answer scoring and reranking in question answering (QA) systems, where annotated answer candidates for known questions provide evidence for validating answer candidates for new questions. The use of CBR promises a continuous increase in answer quality, given user feedback that extends the case base. In the paper, we present the complete approach, emphasizing the use of CBR techniques, namely the structural case base, built with annotated MultiNet graphs, and corresponding graph similarity measures. We cover a priori relations to experienced answer candidates for former questions. We describe the adequate structuring of the case base and develop appropriate similarity measures. Finally we integrate CBR into an existing framework for answer validation and reranking that also includes logical answer validation and a shallow linguistic validation, using a learning-to-rank approach for the final answer ranking based on CBR-related features. In our experiments on QA@CLEF questions, the best learned models make heavy use of CBR features. The advantage already achieved by CBR will increase with time due to the automatic improvement with new user annotations given by relevance feedback.
更多查看译文
关键词
experienced answer candidate,cbr feature,answer scoring,case base,annotated answer candidate,answer validation,logical answer validation,integrated machine learning,answer quality,answer candidate,final answer,case-based reasoning approach,case based reasoning,graph theory,learning artificial intelligence
AI 理解论文
溯源树
样例
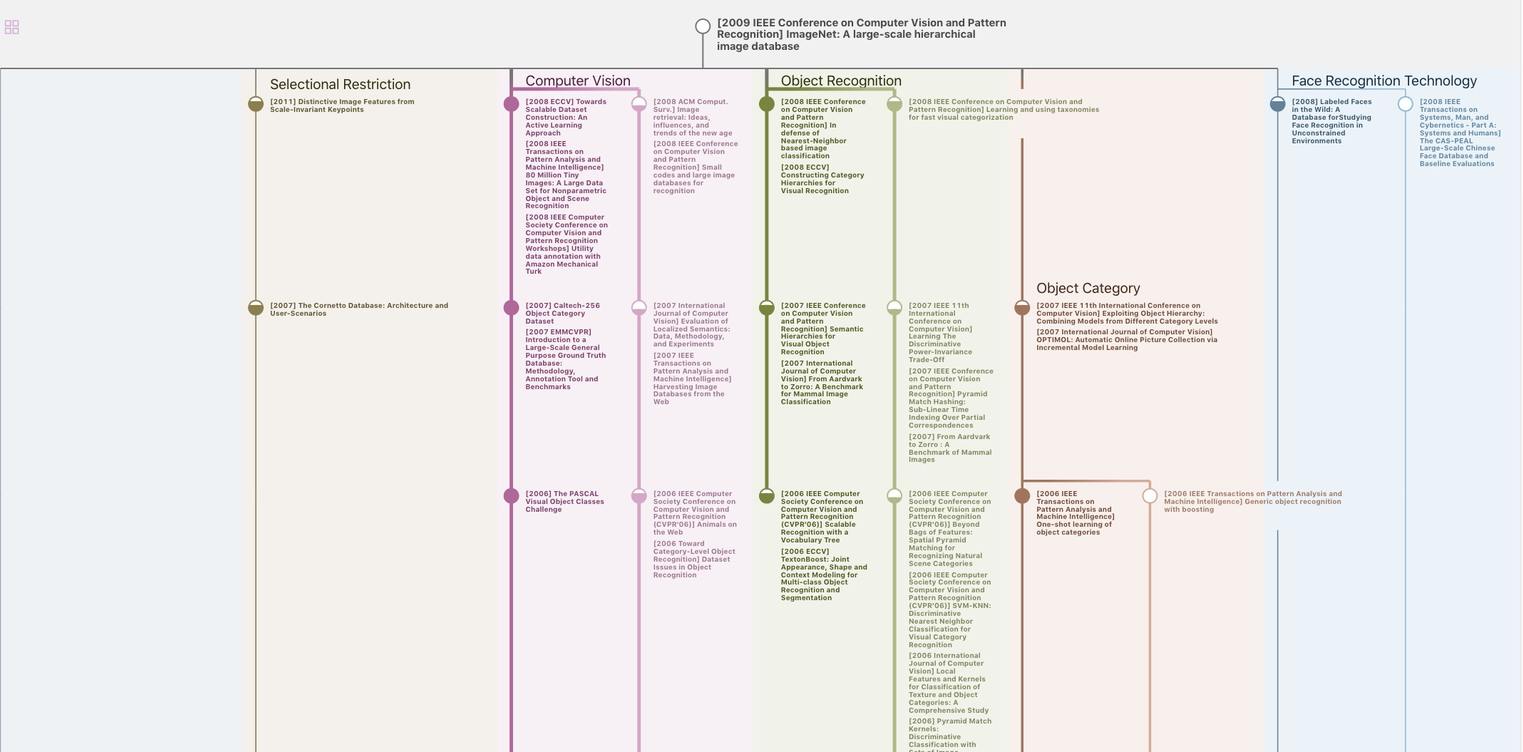
生成溯源树,研究论文发展脉络
Chat Paper
正在生成论文摘要