Improved Feature Selection by Incorporating Gene Similarity into the LASSO
International Journal of Knowledge Discovery in Bioinformatics(2012)
摘要
Personalized medicine is customizing treatments to a patientâs genetic profile, and it has the potential to revolutionize medical practice. An important process used in personalized medicine is gene expression profiling. Analyzing gene expression profiles is difficult, because there are usually few patients and thousands of genes. This leads to the curse of dimensionality. In order to combat this problem, some researchers suggest using prior knowledge to enhance feature selection for supervised learning algorithms. We propose an enhancement to the LASSO, a shrinkage and selection technique that induces parameter sparsity by penalizing a modelâs objective function. Our enhancement gives preference to the selection of genes that are involved in similar biological processes. We expect this to be the case because co-expressed genes are likely to be involved in related pathways. Our modified LASSO selects similar genes by penalizing interaction terms between genes. We devised a coordinate descent algorithm to minimize the corresponding objective function. To evaluate our method, we created simulation data where we compared our model to the standard LASSO model and an interaction LASSO model. Our model outperformed both the standard LASSO and the interaction model in terms of detecting important genes and gene interactions for a reasonable number of training samples. This preliminary study leads us to believe that our method has the potential compete with state of the art methods in gene expression analysis.
更多查看译文
关键词
gene interaction,modified lasso,incorporating gene similarity,important gene,gene expression profiling,analyzing gene expression profile,improved feature selection,similar gene,real gene expression data,interaction lasso model,personalized medicine,standard lasso model,learning artificial intelligence
AI 理解论文
溯源树
样例
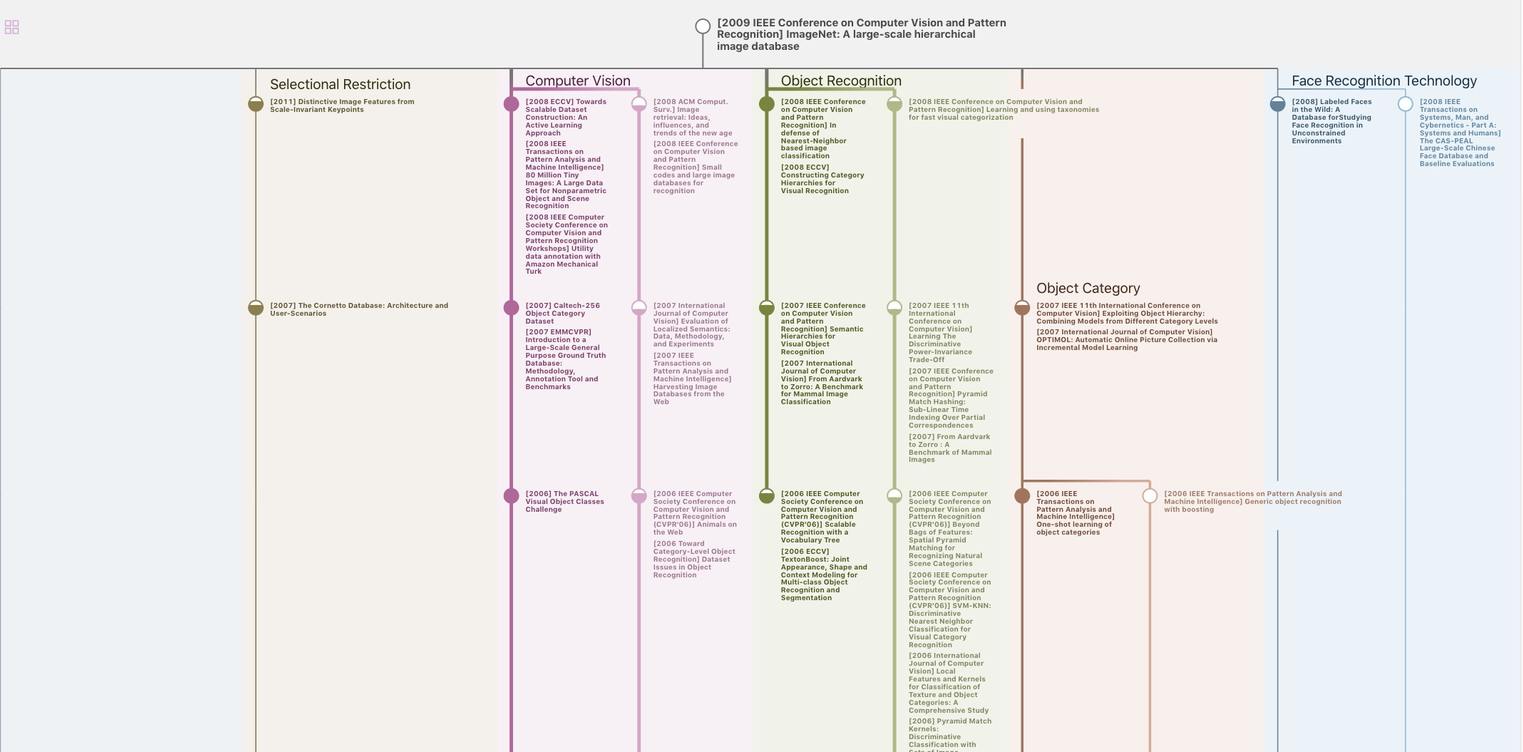
生成溯源树,研究论文发展脉络
Chat Paper
正在生成论文摘要