A discrete Bayesian network framework for discrimination of gene expression profiles
Bioinformatics and Biomedicine(2012)
摘要
Using gene expression profiles for predicting phe-notypic differences that result from cell specializations or diseases poses an important statistical problem. Graphical statistical models such as Bayesian networks may improve the prediction accuracy by identifying alternations in gene regulations due to the experimental conditions. We consider a discrete Bayesian network model that represents pairs of experimental classes by networks that share a common graph structure but have distinct probability tables. We apply a score-based network estimation procedure that maximizes the KL-divergence between class probabilities. The proposed method performs an implicit model selection and does not involve additional complexity penalization parameters. Classification of gene profiles is performed by comparing the likelihood of the estimated class networks. We evaluate the performance of the new model against support vector machine, penalized linear regression and linear Gaussian networks. The classifiers are compared by prediction accuracy across 9 independent data sets from breast and lung cancer studies. The proposed method demonstrates a strong performance against the competitors.
更多查看译文
关键词
gene expression profile,discrete bayesian network model,bayesian network,prediction accuracy,new model,discrete bayesian network framework,gene regulation,implicit model selection,graphical statistical model,gene profile,gene expression,support vector machines,molecular biophysics,cancer,classification,genetics,bayesian networks,regression analysis,graph theory,bioinformatics,gaussian processes,biomedical engineering
AI 理解论文
溯源树
样例
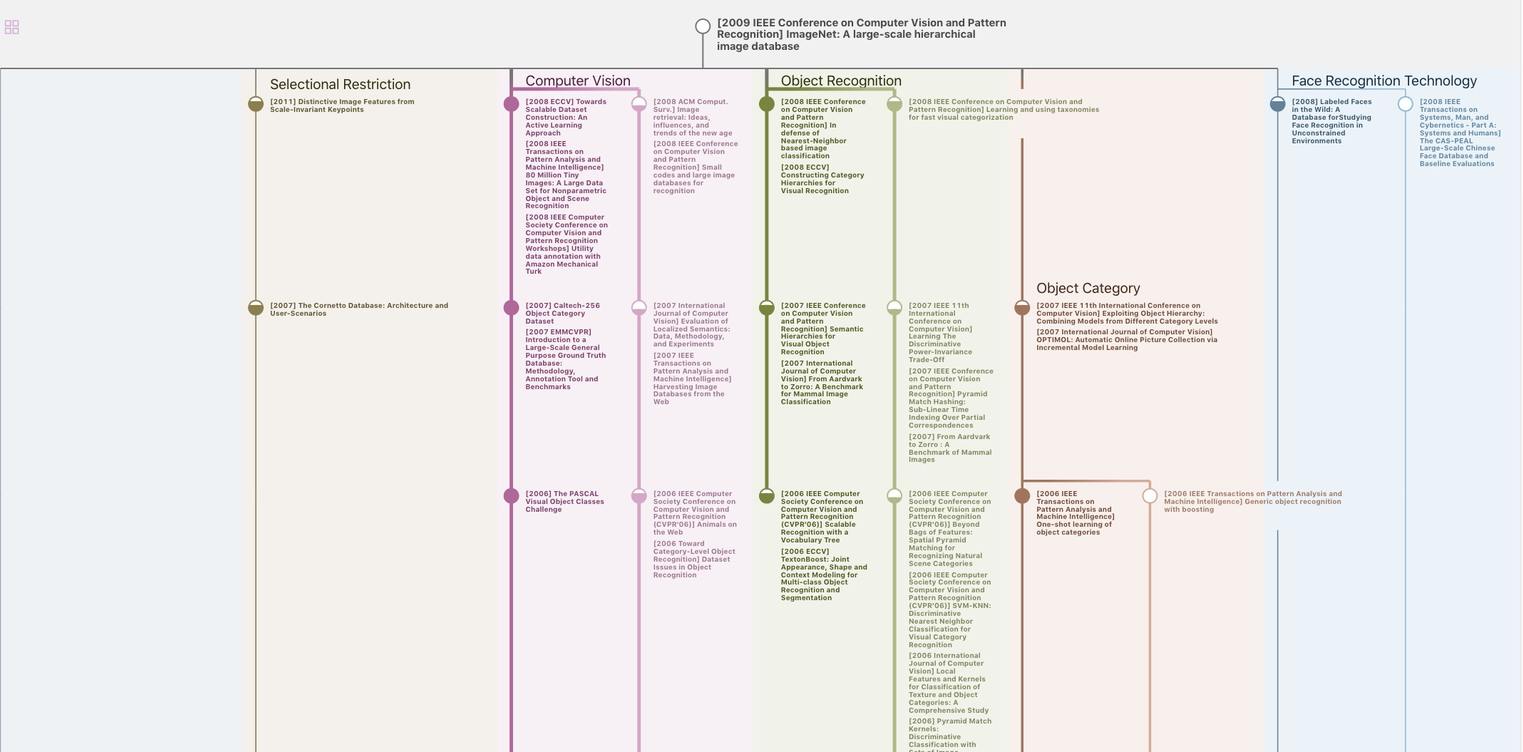
生成溯源树,研究论文发展脉络
Chat Paper
正在生成论文摘要