Low-Rank Transfer Subspace Learning
Data Mining(2012)
摘要
One of the most important challenges in machine learning is performing effective learning when there are limited training data available. However, there is an important case when there are sufficient training data coming from other domains (source). Transfer learning aims at finding ways to transfer knowledge learned from a source domain to a target domain by handling the subtle differences between the source and target. In this paper, we propose a novel framework to solve the aforementioned knowledge transfer problem via low-rank representation constraints. This is achieved by finding an optimal subspace where each datum in the target domain can be linearly represented by the corresponding subspace in the source domain. Extensive experiments on several databases, i.e., Yale B, CMU PIE, UB Kin Face databases validate the effectiveness of the proposed approach and show the superiority to the existing, well-established methods.
更多查看译文
关键词
database management systems,effective learning,aforementioned knowledge transfer problem,cmu pie database,learning (artificial intelligence),important challenge,source domain,domain adaptation,transfer learning,ub kin face database,target domain,ub kin face databases,limited training data,knowledge transfer problem,low-rank representation constraint,low-rank transfer subspace learning,transfer subspace learning,important case,machine learning,corresponding subspace,low-rank,yale b database,training data,learning artificial intelligence
AI 理解论文
溯源树
样例
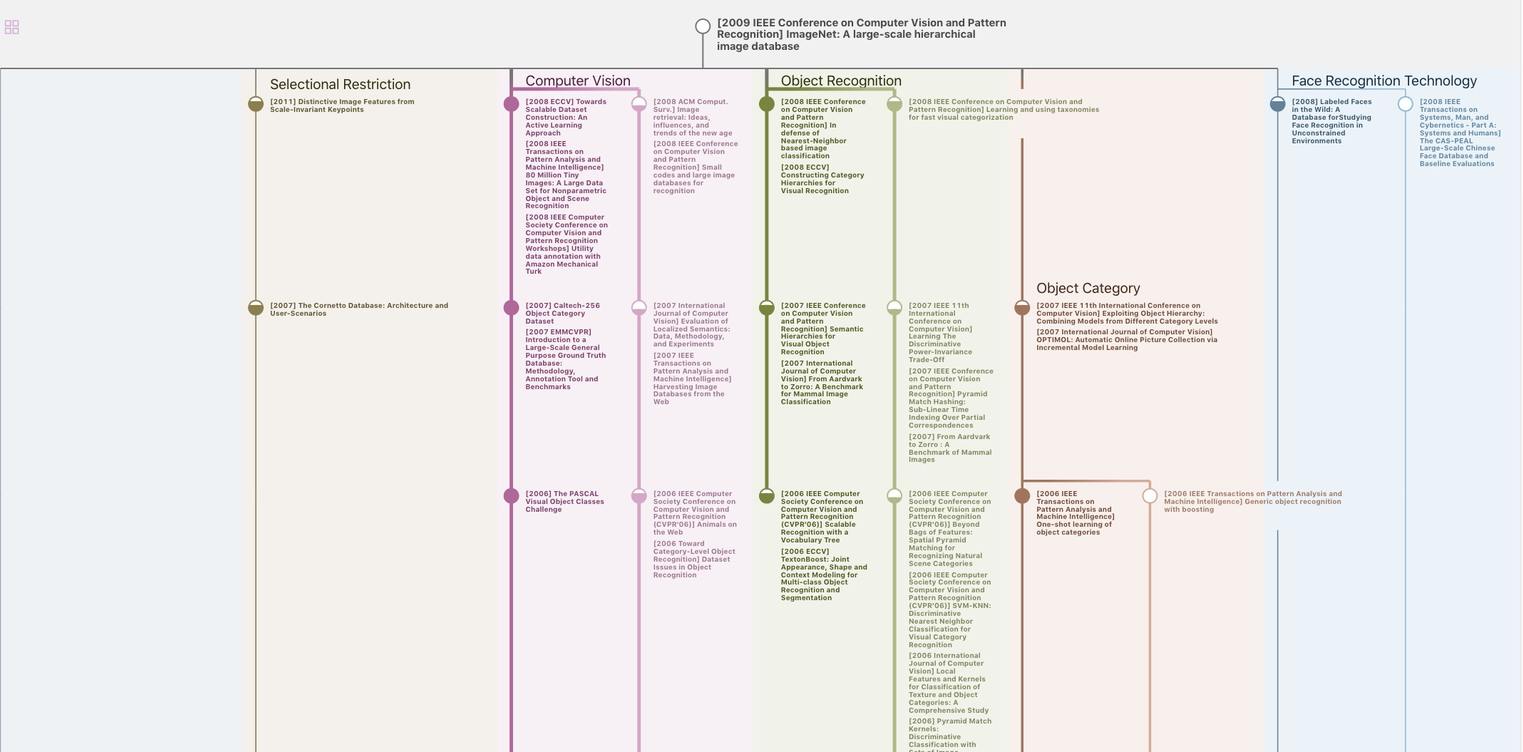
生成溯源树,研究论文发展脉络
Chat Paper
正在生成论文摘要