Stream Classification with Recurring and Novel Class Detection Using Class-Based Ensemble
Data Mining(2012)
摘要
Concept-evolution has recently received a lot of attention in the context of mining data streams. Concept-evolution occurs when a new class evolves in the stream. Although many recent studies address this issue, most of them do not consider the scenario of recurring classes in the stream. A class is called recurring if it appears in the stream, disappears for a while, and then reappears again. Existing data stream classification techniques either misclassify the recurring class instances as another class, or falsely identify the recurring classes as novel. This increases the prediction error of the classifiers, and in some cases causes unnecessary waste in memory and computational resources. In this paper we address the recurring class issue by proposing a novel "class-based" ensemble technique, which substitutes the traditional "chunk-based" ensemble approaches and correctly distinguishes between a recurring class and a novel one. We analytically and experimentally confirm the superiority of our method over state-of-the-art techniques.
更多查看译文
关键词
data mining,pattern classification,chunk- based ensemble approaches,class-based ensemble,class-based ensemble technique,classifier prediction error,computational resources,concept evolution,data stream classification techniques,data stream mining,memory waste,novel class detection,recurring class detection,state-of-the-art techniques,stream classification,novel class,recurring class,stream classification
AI 理解论文
溯源树
样例
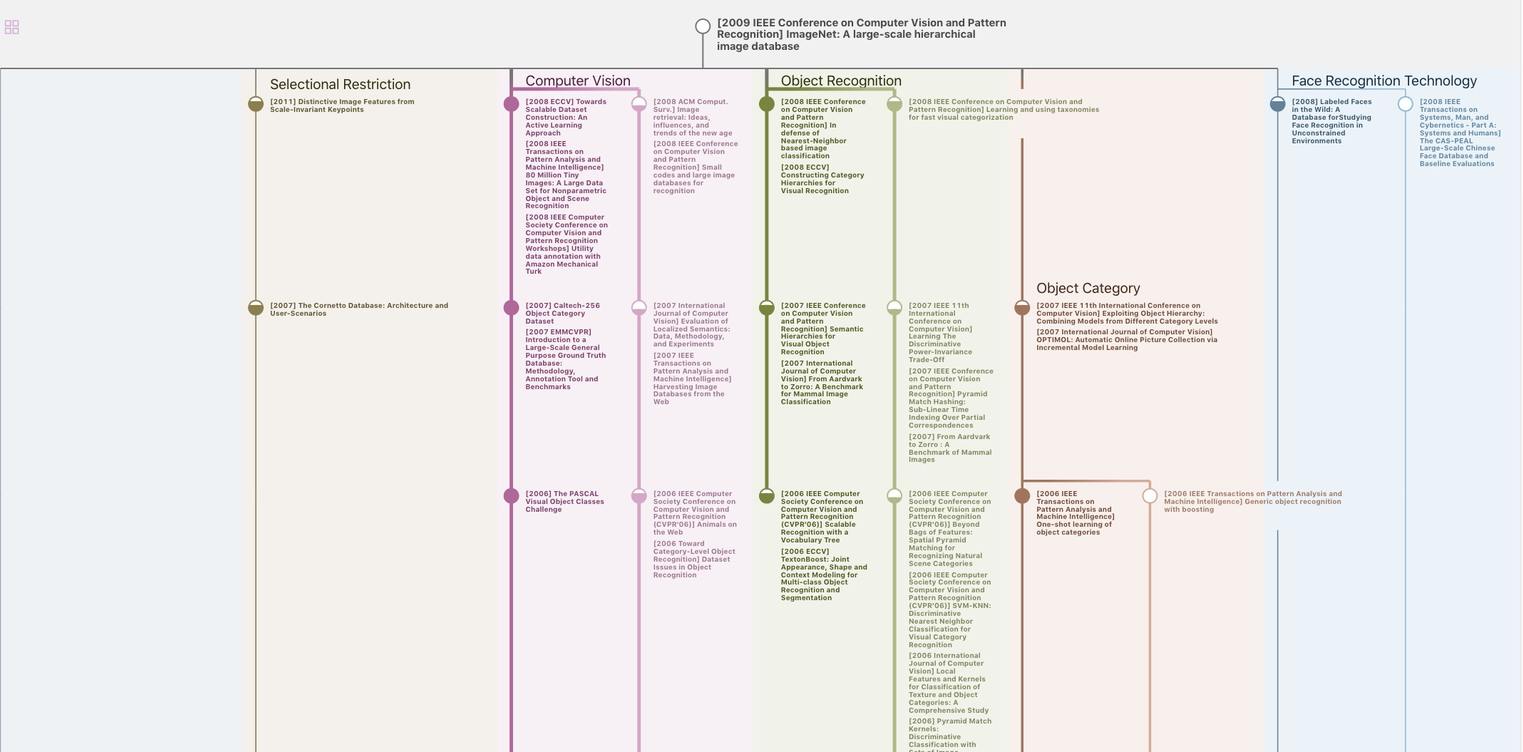
生成溯源树,研究论文发展脉络
Chat Paper
正在生成论文摘要