Hand Posture Recognition from Disparity Cost Map.
Computer Vision – ACCV 2012 Lecture Notes in Computer Science(2013)
Abstract
In this paper, we address the problem of hand posture recognition with a binocular camera. As bare hand has a few landmarks for matching, instead of using accurate matching between two views, we define a kind of mapping score–Disparity Cost Map. The disparity cost map serves as the final hand representation for recognition. As we use the disparity cost map, an explicit segmentation stage is not necessary. Local Binary Pattern (LBP) is used as feature for classification in this paper. In order to align the LBP feature, we further design an annular mask to deal with the problem of scaling, rotation, translation (RST) and search for an accurate bounding box of hand. The experimental results demonstrate the efficiency and robustness of our method. For 15 hand postures in varies cluttered background, the proposed method achieves an average recognition rate of 95% with a SVM classifier.
MoreTranslated text
Key words
disparity cost map,bare hand,final hand representation,hand posture,hand posture recognition,average recognition rate,LBP feature,accurate matching,proposed method,Disparity Cost
AI Read Science
Must-Reading Tree
Example
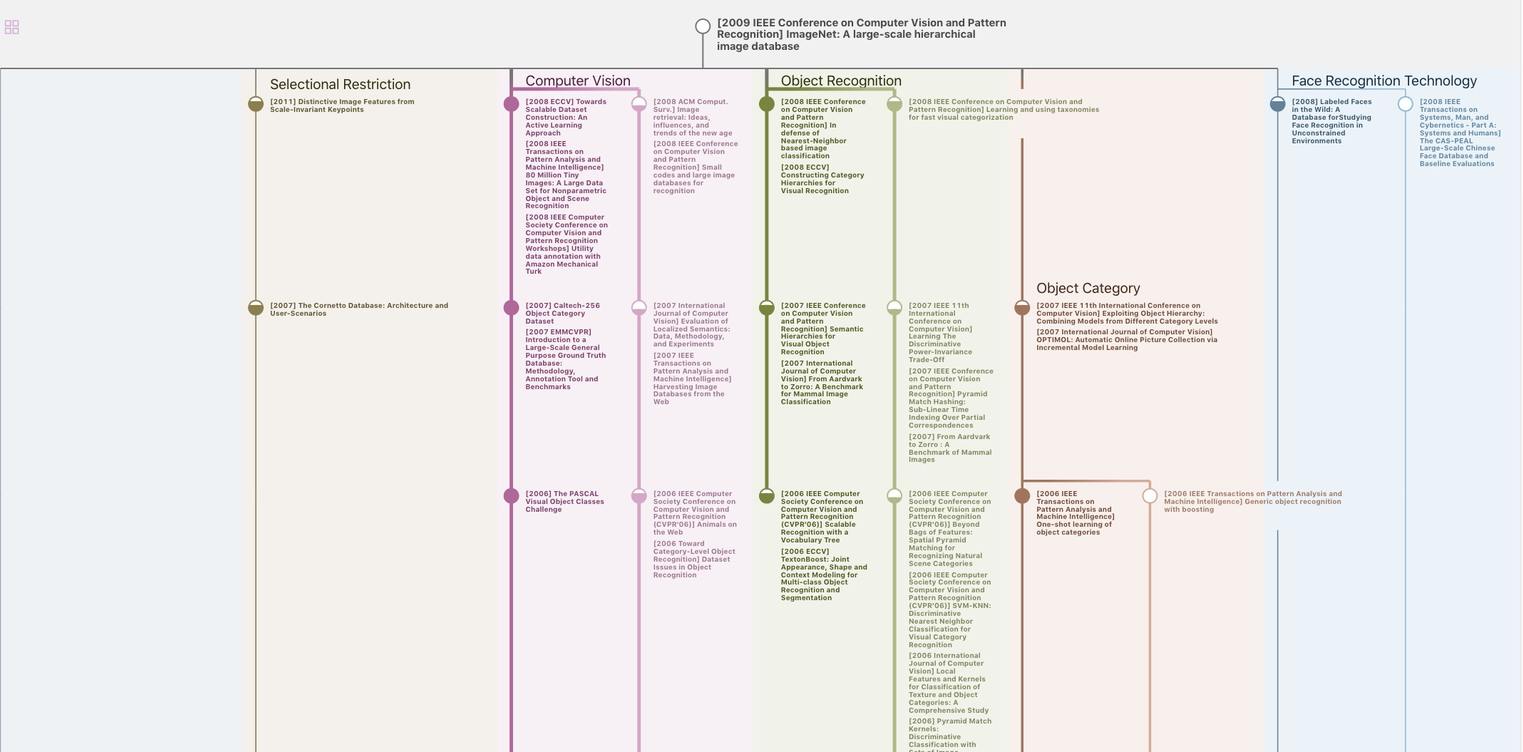
Generate MRT to find the research sequence of this paper
Chat Paper
Summary is being generated by the instructions you defined