Adaptive exploration for large-scale protein analysis in the molecular dynamics database
SSDBM '13: Proceedings of the 25th International Conference on Scientific and Statistical Database Management(2013)
摘要
Molecular dynamics (MD) simulations generate detailed time-series data of all-atom motions. These simulations are leading users of the world's most powerful supercomputers, and are standard-bearers for a wide range of high-performance computing (HPC) methods. However, MD data exploration and analysis is in its infancy in terms of scalability, ease-of-use, and ultimately its ability to answer 'grand challenge' science questions. This demonstration introduces the Molecular Dynamics Database (MDDB) project at Johns Hopkins, to study the co-design of database methods for deep on-the-fly exploratory MD analyses with HPC simulations. Data exploration in MD suffers from a \"human bottleneck\", where the laborious administration of simulations leaves little room for domain experts to focus on tackling science questions. MDDB exploits the data-rich nature of MD simulations to provide adaptive control of the exploration process with machine learning techniques, specifically reinforcement learning (RL). We present MDDB's data and queries, architecture, and its use of RL methods. Our audience will co-operate with our steering algorithm and science partners, and witness MDDB's abilities to significantly reduce exploration times and direct computation resources to where they best address science questions.
更多查看译文
关键词
science question,exploration process,molecular dynamics database,science partner,witness mddb,deep on-the-fly exploratory md,time-series data,data exploration,large-scale protein analysis,adaptive exploration,md simulation,md data exploration,exploration time,outlier detection,categorical data
AI 理解论文
溯源树
样例
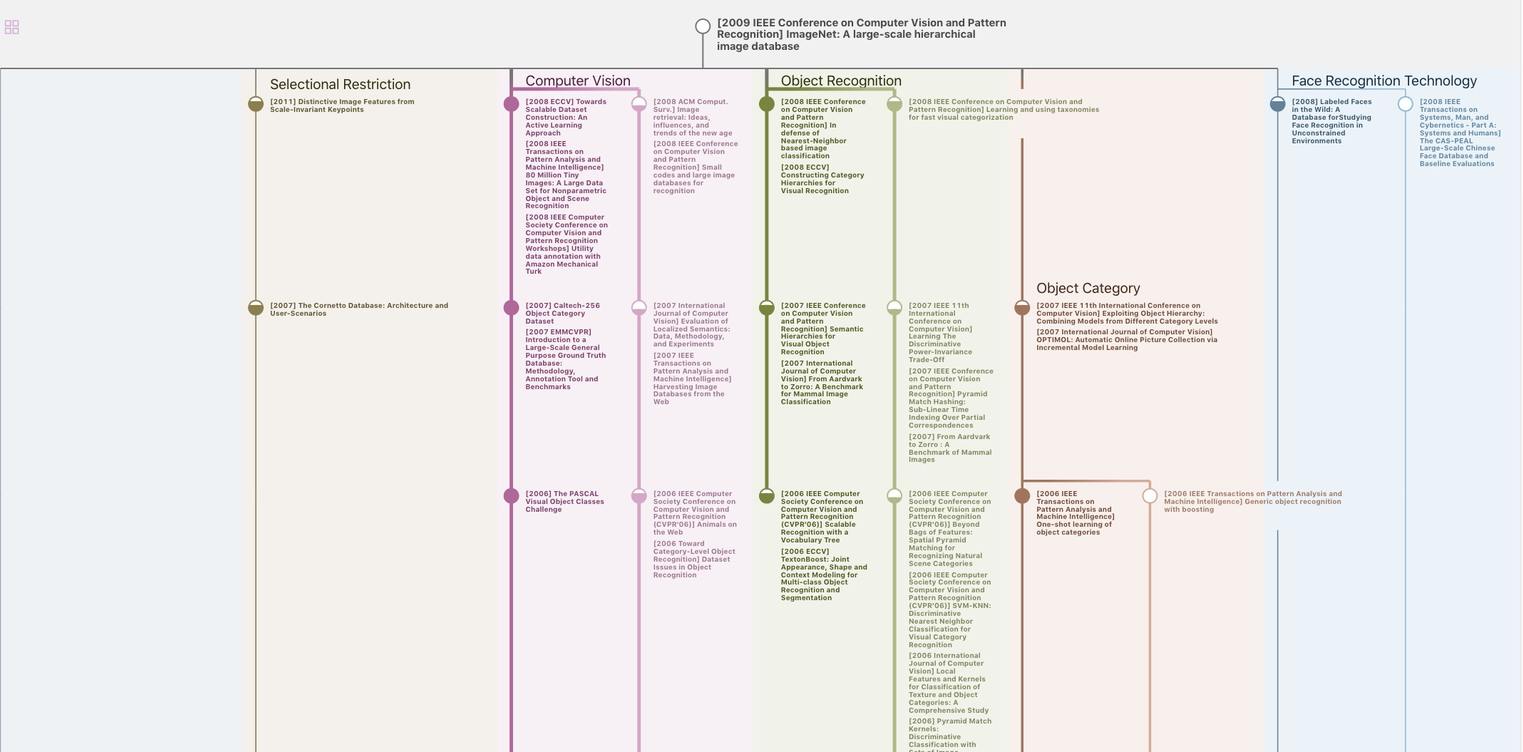
生成溯源树,研究论文发展脉络
Chat Paper
正在生成论文摘要