Spectrally adapted mercer kernels for support vector signal interpolation
Signal Processing(2011)
摘要
Interpolation of nonuniformly sampled signals in the presence of noise is a hard and deeply analyzed problem. On the one hand, classical approaches like the Wiener filter use the second order statistics of the signal, and hence its spectrum, as a priori knowledge for finding the solution. On the other hand, Support Vector Machines (SVM) with Gaussian and sinc Mercer kernels have been previously proposed for time series interpolation, with good properties in terms of regularization and sparseness. Hence, in this paper we propose to use SVM-based algorithms with kernels having their spectra adapted to the signal spectrum, and to analyze their suitability for nonuniform interpolation. For this purpose, we investigate the performance of the SVM with autocorrelation kernels for one-dimensional time series interpolation. Simulations with synthetic signals show that SVM-based algorithms with the proposed kernels provide good performance for signals with different kinds of spectrum, even in the case of highly nonuniform sampling.
更多查看译文
关键词
sinc kernel,signal processing application,low pass kernel,svm kernel,support vector nonuniform interpolation,band-pass signal,classic svm,sinc mercer kernel,modulated kernel,autocorrelation kernel,observed signal,support vector regression
AI 理解论文
溯源树
样例
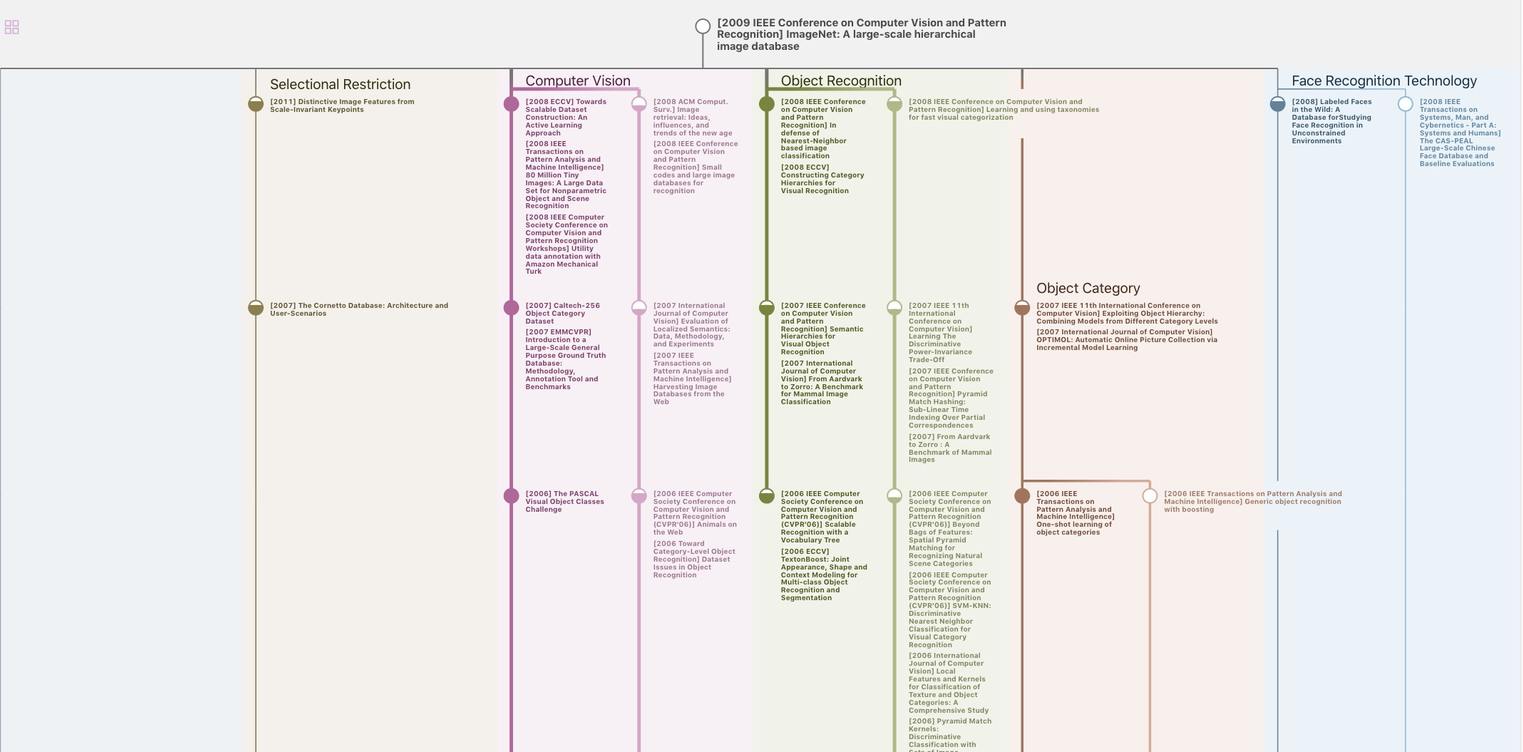
生成溯源树,研究论文发展脉络
Chat Paper
正在生成论文摘要