Integrated instance- and class-based generative modeling for text classification.
ADCS '13: Proceedings of the 18th Australasian Document Computing Symposium(2013)
摘要
Statistical methods for text classification are predominantly based on the paradigm of class-based learning that associates class variables with features, discarding the instances of data after model training. This results in efficient models, but neglects the fine-grained information present in individual documents. Instance-based learning uses this information, but suffers from data sparsity with text data. In this paper, we propose a generative model called Tied Document Mixture (TDM) for extending Multinomial Naive Bayes (MNB) with mixtures of hierarchically smoothed models for documents. Alternatively, TDM can be viewed as a Kernel Density Classifier using class-smoothed Multinomial kernels. TDM is evaluated for classification accuracy on 14 different datasets for multi-label, multi-class and binary-class text classification tasks and compared to instance- and class-based learning baselines. The comparisons to MNB demonstrate a substantial improvement in accuracy as a function of available training documents per class, ranging up to average error reductions of over 26% in sentiment classification and 65% in spam classification. On average TDM is as accurate as the best discriminative classifiers, but retains the linear time complexities of instance-based learning methods, with exact algorithms for both model estimation and inference.
更多查看译文
关键词
integrated instance,average tdm,instance-based learning method,class-based learning,text classification,instance-based learning,data sparsity,spam classification,class-based generative modeling,sentiment classification,classification accuracy,binary-class text classification task,instance based learning
AI 理解论文
溯源树
样例
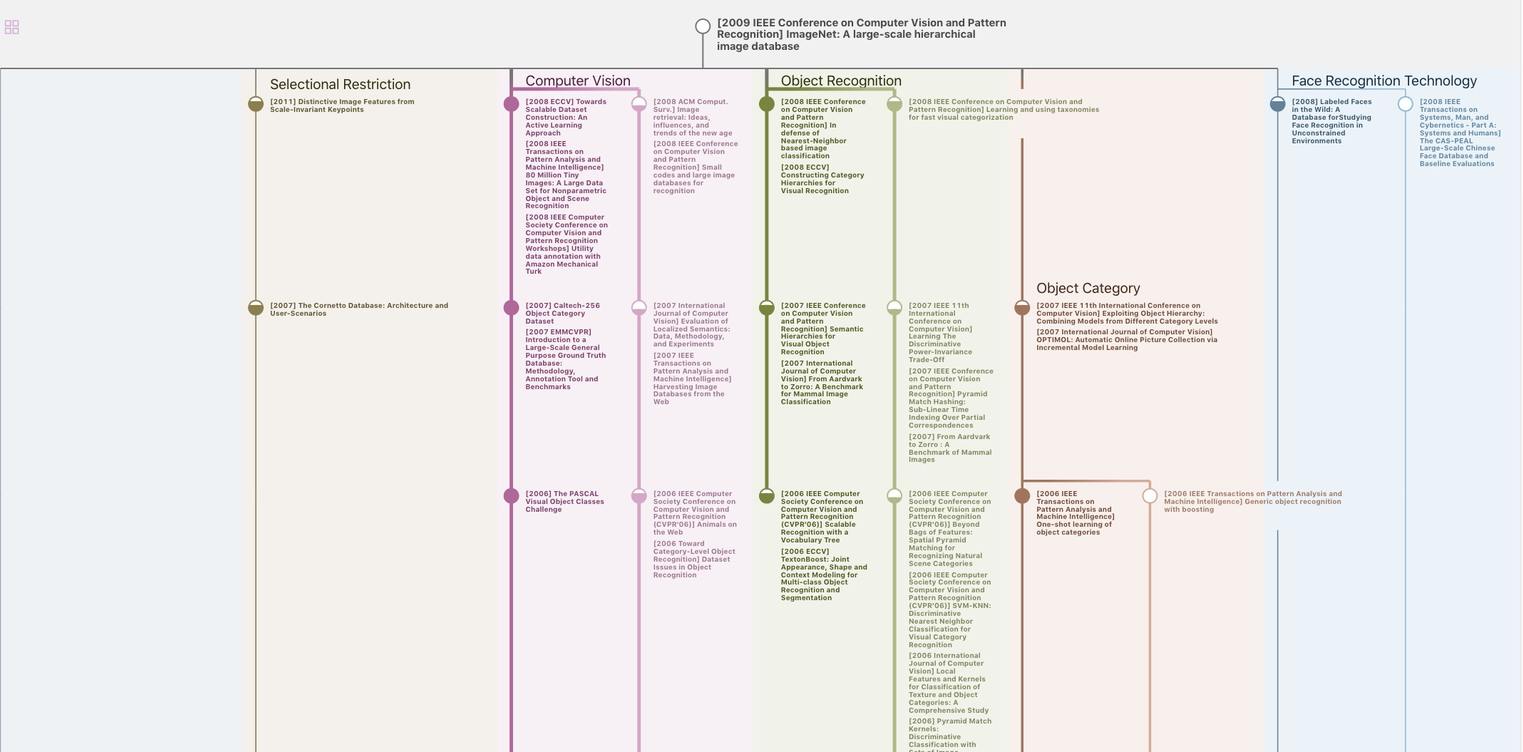
生成溯源树,研究论文发展脉络
Chat Paper
正在生成论文摘要