Handwriting Word Recognition Using Windowed Bernoulli Hmms
PATTERN RECOGNITION LETTERS(2014)
摘要
Hidden Markov Models (HMMs) are now widely used for off-line handwriting recognition in many languages. As in speech recognition, they are usually built from shared, embedded HMMs at symbol level, where state-conditional probability density functions in each HMM are modeled with Gaussian mixtures. In contrast to speech recognition, however, it is unclear which kind of features should be used and, indeed, very different features sets are in use today. Among them, we have recently proposed to directly use columns of raw, binary image pixels, which are directly fed into embedded Bernoulli (mixture) HMMs, that is, embedded HMMs in which the emission probabilities are modeled with Bernoulli mixtures. The idea is to by-pass feature extraction and to ensure that no discriminative information is filtered out during feature extraction, which in some sense is integrated into the recognition model. In this work,, column bit vectors are extended by means of a sliding window of adequate width to better capture image context at each horizontal position of the word image. Using these windowed Bernoulli mixture HMMs, good results are reported on the well-known IAM and RIMES databases of Latin script, and in particular, state-of-the-art results are provided on the IfN/ENIT database of Arabic handwritten words. (C) 2012 Elsevier B.V. All rights reserved.
更多查看译文
关键词
HTR,Bernoulli HMMs,Latin,Arabic,Sliding window
AI 理解论文
溯源树
样例
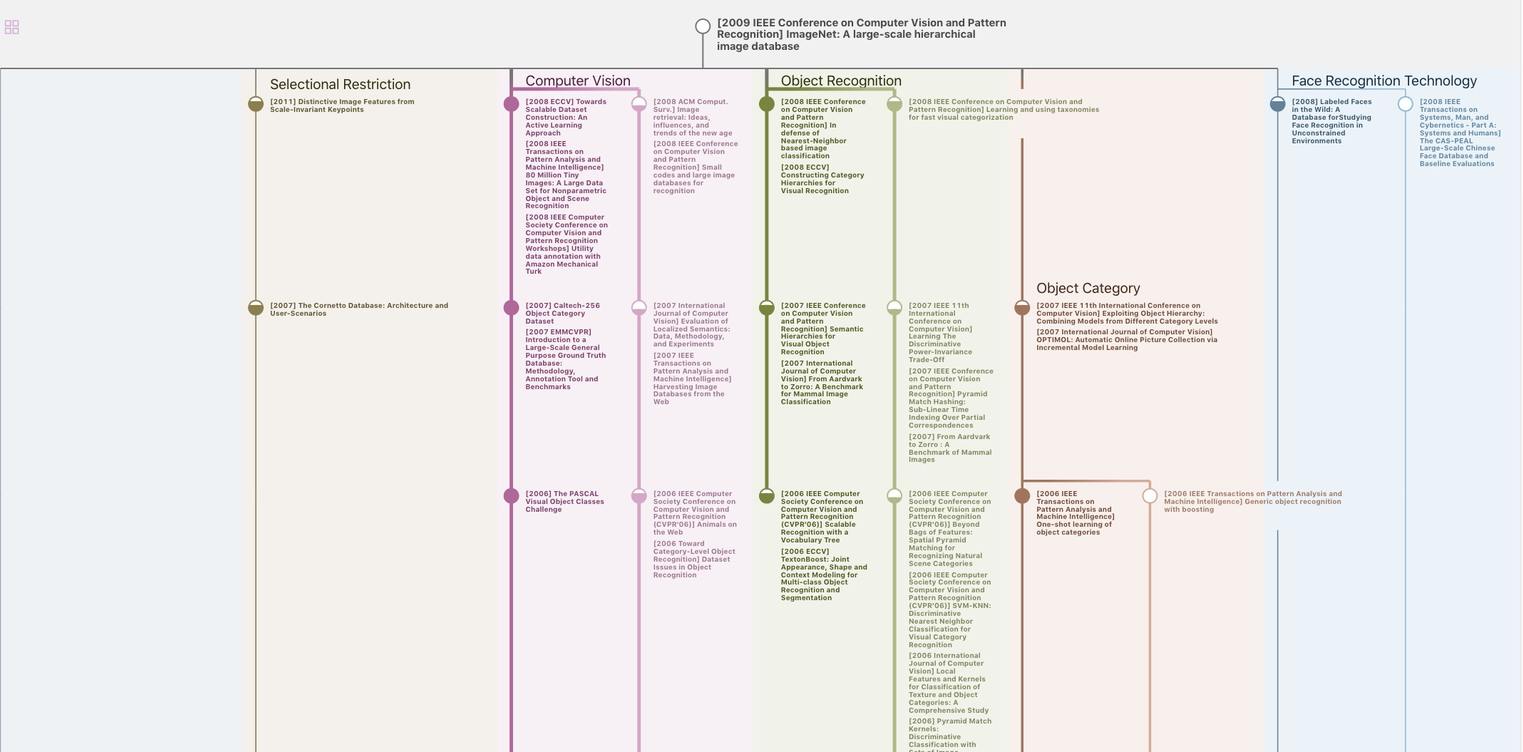
生成溯源树,研究论文发展脉络
Chat Paper
正在生成论文摘要