Asymmetric Least Squares Support Vector Machine Classifiers
Computational Statistics & Data Analysis(2014)
摘要
In the field of classification, the support vector machine (SVM) pursues a large margin between two classes. The margin is usually measured by the minimal distance between two sets, which is related to the hinge loss or the squared hinge loss. However, the minimal value is sensitive to noise and unstable to re-sampling. To overcome this weak point, the expectile value is considered to measure the margin between classes instead of the minimal value. Motivated by the relation between the expectile value and the asymmetric squared loss, an asymmetric least squares SVM (aLS-SVM) is proposed. The proposed aLS-SVM can also be regarded as an extension to the LS-SVM and the L2-SVM. Theoretical analysis and numerical experiments on the aLS-SVM illustrate its insensitivity to noise around the boundary and its stability to re-sampling.
更多查看译文
关键词
Classification,Support vector machine,Least squares support vector machine,Asymmetric least squares
AI 理解论文
溯源树
样例
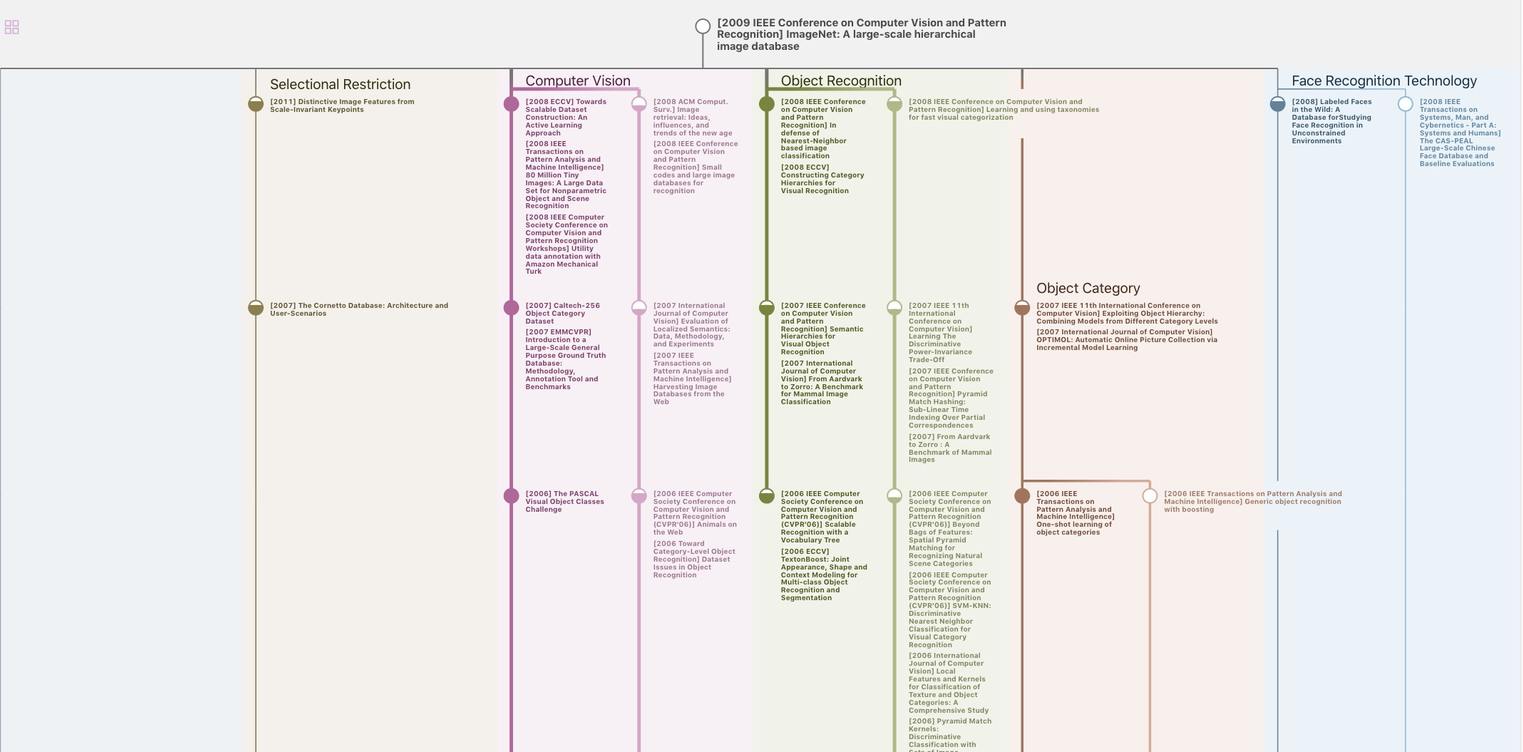
生成溯源树,研究论文发展脉络
Chat Paper
正在生成论文摘要