Multiscale TILT Feature Detection with Application to Geometric Image Segmentation
Computer Vision Workshops(2013)
摘要
Motivated by the theory of low-rank matrix representation, a new type of invariant image feature, called transform-invariant low-rank texture (TILT), has been recently proposed. However, the application of TILT features in computer vision has been severely limited by two major problems. First, TILT feature representation is based on the assumption that the given image contains only one dominant low-rank region, which typically does not hold in natural images. Second, when multiple low-rank regions are present, the existing TILT detection methods either randomly sample the image or apply to fixed grid coordinates, both of which cannot guarantee good recovery of salient low-rank image features. In this paper, we propose a novel algorithm to address these two important issues. First, utilizing super pixels and the concept of canonical rank derived from TILT, we introduce a method to segment natural images into a geometric layer and a non-geometric layer. Second, we apply a Markov random field model to a multiscale low-rank representation of the image geometric layer, and obtain an effective algorithm to detect TILT features. Finally, we present an application of the multiscale TILT detection algorithm to the classical problem of building facade segmentation. Extensive experiments are conducted on the Pankrac building database to demonstrate the efficacy of the algorithms.
更多查看译文
关键词
tilt feature,image representation,low-rank matrix representation,dominant low-rank region,multiscale low-rank representation,markov random field model,multiple low-rank region,building facade segmentation,image segmentation,feature detection,existing tilt detection method,salient low-rank image feature,geometric layer,invariant image feature,pankrac building database,multiscale tilt feature detection,transform-invariant low-rank texture,image sampling,fixed grid coordinates,multiscale tilt,tilt feature representation,feature extraction,natural image segmentation,object detection,geometric image segmentation,computer vision,geometric image,image texture,super pixels,markov processes,nongeometric layer,multiscale tilt detection algorithm,canonical rank concept
AI 理解论文
溯源树
样例
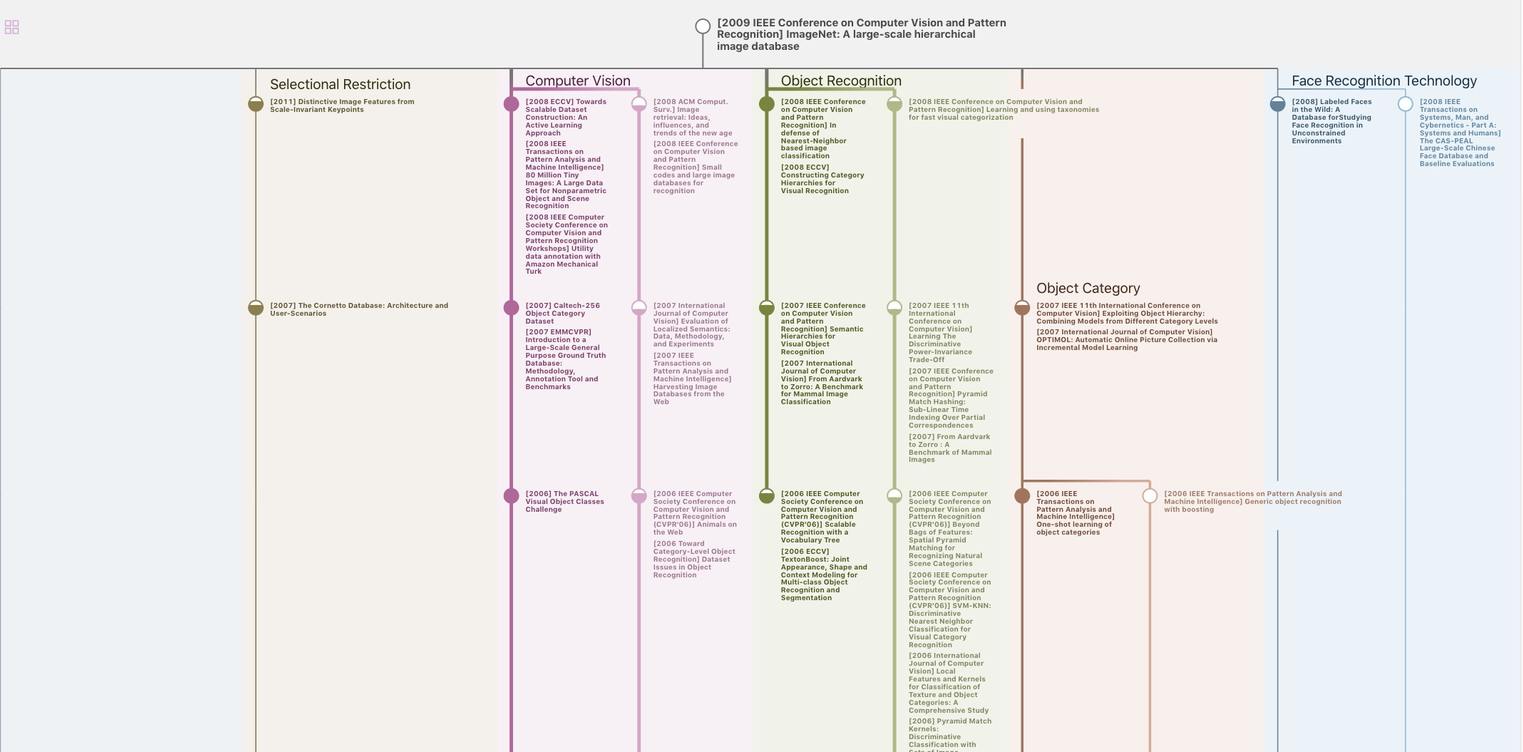
生成溯源树,研究论文发展脉络
Chat Paper
正在生成论文摘要