Latent Data Association: Bayesian Model Selection for Multi-target Tracking
Computer Vision(2013)
摘要
We propose a novel parametrization of the data association problem for multi-target tracking. In our formulation, the number of targets is implicitly inferred together with the data association, effectively solving data association and model selection as a single inference problem. The novel formulation allows us to interpret data association and tracking as a single Switching Linear Dynamical System (SLDS). We compute an approximate posterior solution to this problem using a dynamic programming/message passing technique. This inference-based approach allows us to incorporate richer probabilistic models into the tracking system. In particular, we incorporate inference over inliers/outliers and track termination times into the system. We evaluate our approach on publicly available datasets and demonstrate results competitive with, and in some cases exceeding the state of the art.
更多查看译文
关键词
belief networks,dynamic programming,message passing,sensor fusion,target tracking,Bayesian model selection,SLDS,data association problem,dynamic programming,inference-based approach,latent data association,message passing technique,multitarget tracking,single inference problem,switching linear dynamical system,bayesian inference,data association,hybrid inference,message passing,model selection,multi-target tracking
AI 理解论文
溯源树
样例
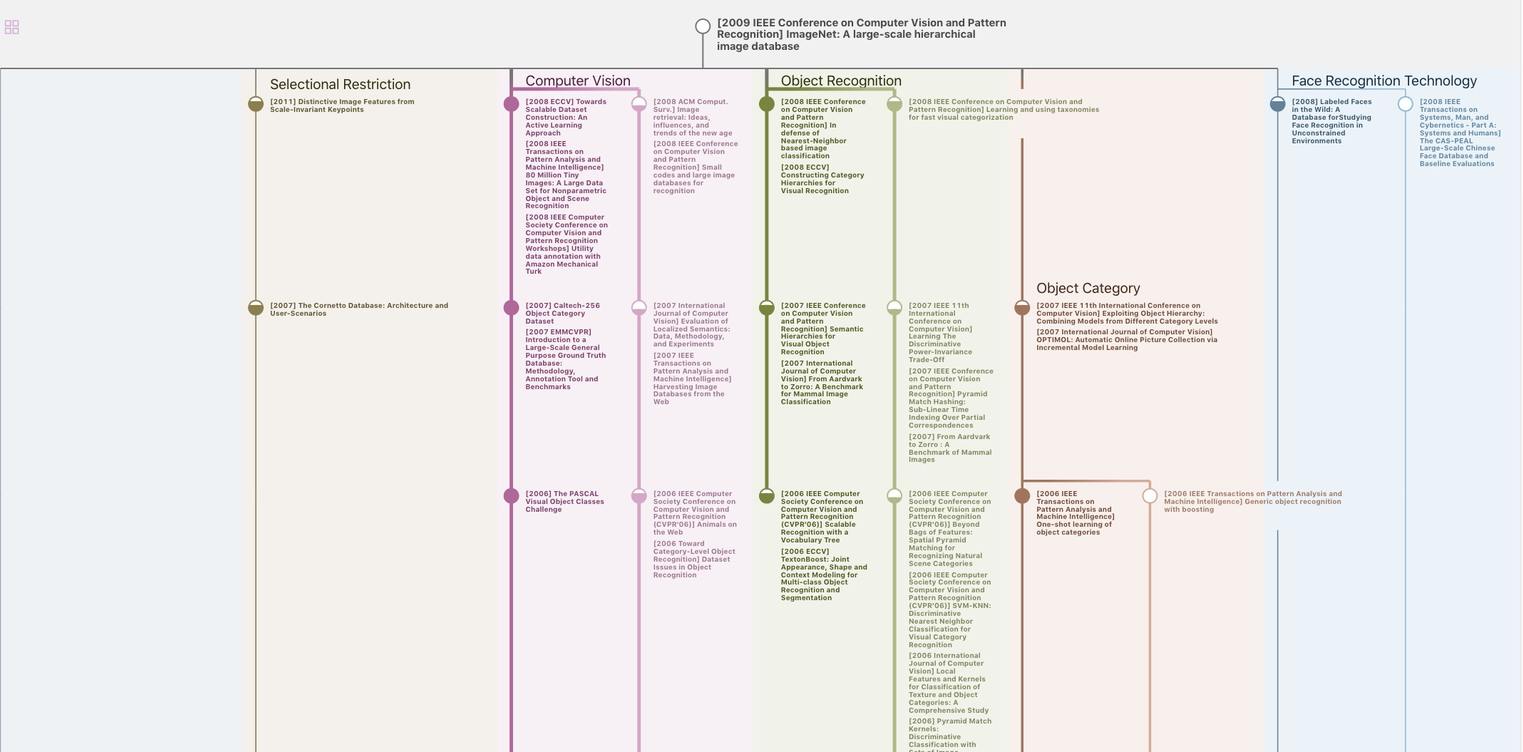
生成溯源树,研究论文发展脉络
Chat Paper
正在生成论文摘要