A General Dense Image Matching Framework Combining Direct And Feature-Based Costs
2013 IEEE INTERNATIONAL CONFERENCE ON COMPUTER VISION (ICCV)(2013)
摘要
Dense motion field estimation (typically optical flow, stereo disparity and surface registration) is a key computer vision problem. Many solutions have been proposed to compute small or large displacements, narrow or wide baseline stereo disparity, but a unified methodology is still lacking. We here introduce a general framework that robustly combines direct and feature-based matching. The feature-based cost is built around a novel robust distance function that handles keypoints and "weak" features such as segments. It allows us to use putative feature matches which may contain mismatches to guide dense motion estimation out of local minima. Our framework uses a robust direct data term (AD-Census). It is implemented with a powerful second order Total Generalized Variation regularization with external and self-occlusion reasoning. Our framework achieves state of the art performance in several cases (standard optical flow benchmarks, wide-baseline stereo and non-rigid surface registration). Our framework has a modular design that customizes to specific application needs.
更多查看译文
关键词
computer vision,feature extraction,image matching,image sequences,inference mechanisms,motion estimation,stereo image processing,AD-Census,dense image matching framework,dense motion field estimation,direct costs,direct-based matching,feature-based costs,feature-based matching,key computer vision problem,local minima,narrow baseline stereo disparity,nonrigid surface registration,putative feature matches,robust direct data term,robust distance function,second order total generalized variation regularization,self-occlusion reasoning,standard optical flow benchmarks,stereo disparity,surface registration,wide baseline stereo disparity,SIFT,features,large displacements,non-rigid surface registration,optical flow,segments,stereo,wide-baseline,
AI 理解论文
溯源树
样例
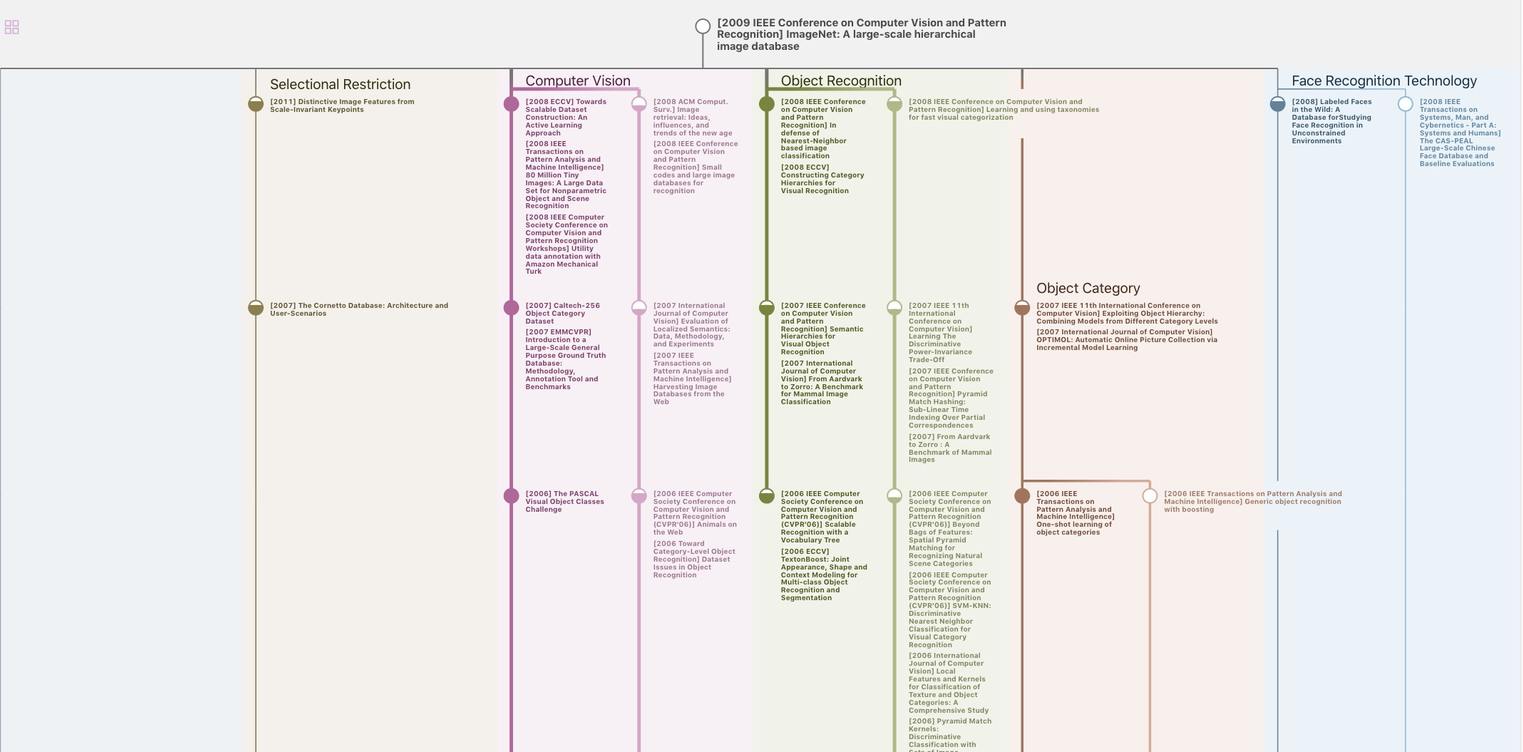
生成溯源树,研究论文发展脉络
Chat Paper
正在生成论文摘要