A particle swarm optimization based simultaneous learning framework for clustering and classification
Pattern Recognition(2014)
摘要
A particle swarm optimization based simultaneous learning framework for clustering and classification (PSOSLCC) is proposed in this paper. Firstly, an improved particle swarm optimization (PSO) is used to partition the training samples, the number of clusters must be given in advance, an automatic clustering algorithm rather than the trial and error is adopted to find the proper number of clusters, and a set of clustering centers is obtained to form classification mechanism. Secondly, in order to exploit more useful local information and get a better optimizing result, a global factor is introduced to the update strategy update strategy of particle in PSO. PSOSLCC has been extensively compared with fuzzy relational classifier (FRC), vector quantization and learning vector quantization (VQ+LVQ3), and radial basis function neural network (RBFNN), a simultaneous learning framework for clustering and classification (SCC) over several real-life datasets, the experimental results indicate that the proposed algorithm not only greatly reduces the time complexity, but also obtains better classification accuracy for most datasets used in this paper. Moreover, PSOSLCC is applied to a real world application, namely texture image segmentation with a good performance obtained, which shows that the proposed algorithm has a potential of classifying the problems with large scale.
更多查看译文
关键词
particle swarm optimization,classification mechanism,clustering center,vector quantization,improved particle swarm optimization,better classification accuracy,automatic clustering algorithm,proper number,proposed algorithm,simultaneous learning framework,classification,image segmentation,clustering
AI 理解论文
溯源树
样例
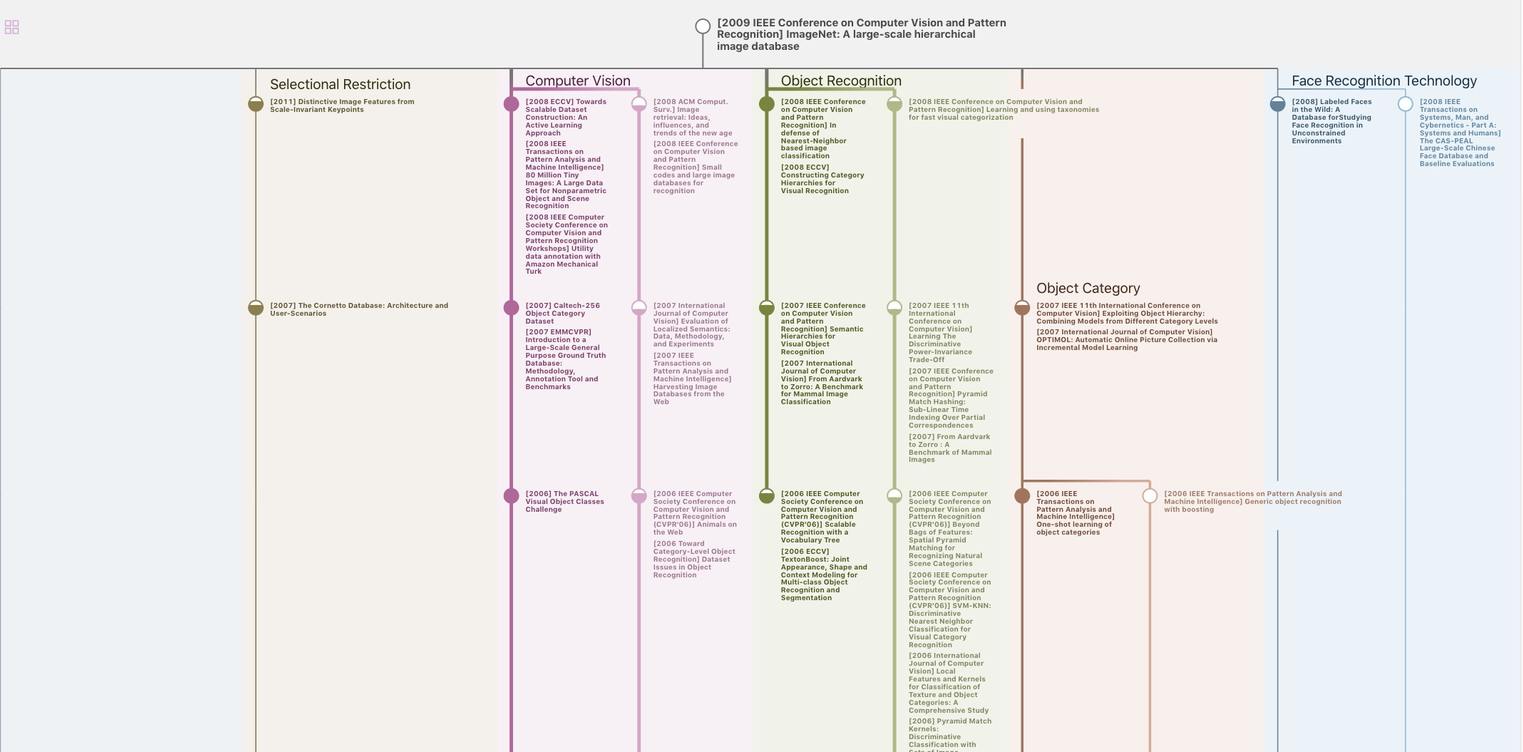
生成溯源树,研究论文发展脉络
Chat Paper
正在生成论文摘要