Collaborative information acquisition for data-driven decisions
MACHINE LEARNING(2013)
摘要
Data-driven predictive models are routinely used by government agencies and industry to improve the efficiency of their decision-making. In many cases, agencies acquire training data over time, incurring both direct and opportunity costs. Active learning can be used to acquire particularly informative training data that improve learning cost-effectively. However, when multiple models are used to inform decisions, prior work on active learning has significant limitations: either it improves the accuracy of predictive models without regard to how accuracy affects decision making or it addresses only decisions informed by a single predictive model. We propose that decisions informed by multiple models warrant a new kind of Collaborative Information Acquisition (CIA) policy that allows multiple learners to reason collaboratively about informative acquisitions. This paper focuses on tax audit decisions, which affect a vital revenue source for governments worldwide. Because audits are costly to conduct, active learning policies can help identify particularly informative audits to improve future decisions. However, existing active learning models are poorly suited to audit decisions, because audits are best informed by multiple predictive models. We develop a CIA policy to improve the decisions the models inform, and we demonstrate that CIA can substantially increase sales tax revenues. We also demonstrate that the CIA policy can improve decisions to target directly individuals in a donation campaign. Finally, we discuss and demonstrate the risks for decision making of the naive use of existing active learning policies.
更多查看译文
关键词
Active learning,Predictive modeling,Decision making,Audit selection,Direct targeting,Tax audit
AI 理解论文
溯源树
样例
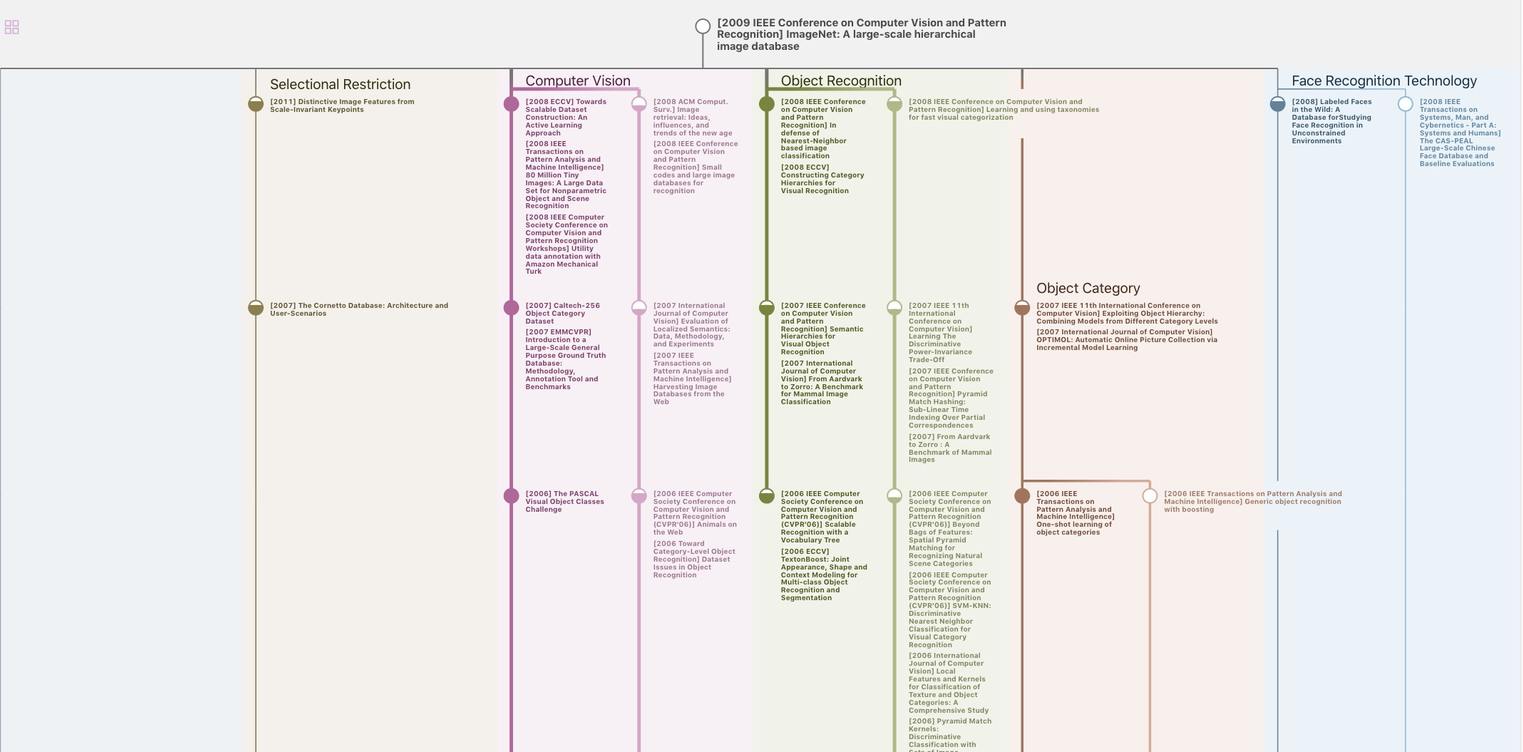
生成溯源树,研究论文发展脉络
Chat Paper
正在生成论文摘要