Adaptive on-line similarity measure for direct visual tracking
Image and Vision Computing(2014)
摘要
This paper presents an on-line adaptive metric to estimate the similarity between the target representation model and new image received at every time instant. The similarity measure, also known as observation likelihood, plays a crucial role in the accuracy and robustness of visual tracking. In this work, an L2-norm is adaptively weighted at every matching step to calculate the similarity between the target model and image descriptors. A histogram-based classifier is learned on-line to categorize the matching errors into three classes namely i) image noise, ii) significant appearance changes, and iii) outliers. A robust weight is assigned to each matching error based on the class label. Therefore, the proposed similarity measure is able to reject outliers and adapt to the target model by discriminating the appearance changes from the undesired outliers. The experimental results show the superiority of the proposed method with respect to accuracy and robustness in the presence of severe and long-term occlusion and image noise in comparison with commonly used robust regressors.
更多查看译文
关键词
image noise,matching error,matching step,target model,adaptive on-line similarity measure,similarity measure,new image,direct visual tracking,image descriptors,target representation model,proposed similarity measure,appearance change
AI 理解论文
溯源树
样例
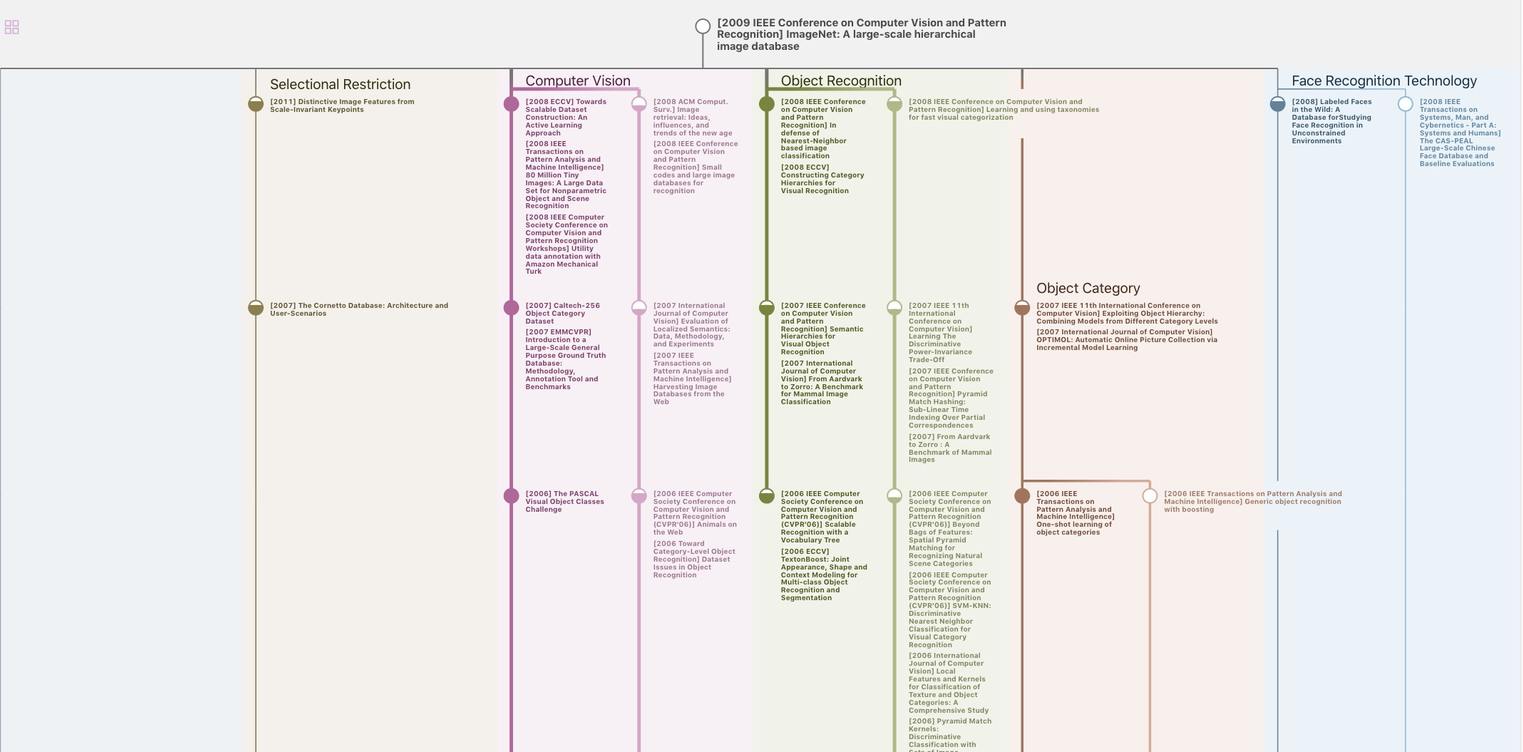
生成溯源树,研究论文发展脉络
Chat Paper
正在生成论文摘要