Autoregressive Networks
JOURNAL OF MACHINE LEARNING RESEARCH(2023)
摘要
We propose a first-order autoregressive (i.e. AR(1)) model for dynamic network processes in which edges change over time while nodes remain unchanged. The model depicts the dynamic changes explicitly. It also facilitates simple and efficient statistical inference meth-ods including a permutation test for diagnostic checking for the fitted network models. The proposed model can be applied to the network processes with various underlying structures but with independent edges. As an illustration, an AR(1) stochastic block model has been investigated in depth, which characterizes the latent communities by the transition proba-bilities over time. This leads to a new and more effective spectral clustering algorithm for identifying the latent communities. We have derived a finite sample condition under which the perfect recovery of the community structure can be achieved by the newly defined spec-tral clustering algorithm. Furthermore the inference for a change point is incorporated into the AR(1) stochastic block model to cater for possible structure changes. We have derived the explicit error rates for the maximum likelihood estimator of the change-point. Appli-cation with three real data sets illustrates both relevance and usefulness of the proposed AR(1) models and the associate inference methods.
更多查看译文
关键词
AR(1) networks,Change point,Dynamic stochastic block model,Hamming distance,Maximum likelihood estimation,Spectral clustering algorithm,Yule-Walker equa-tion
AI 理解论文
溯源树
样例
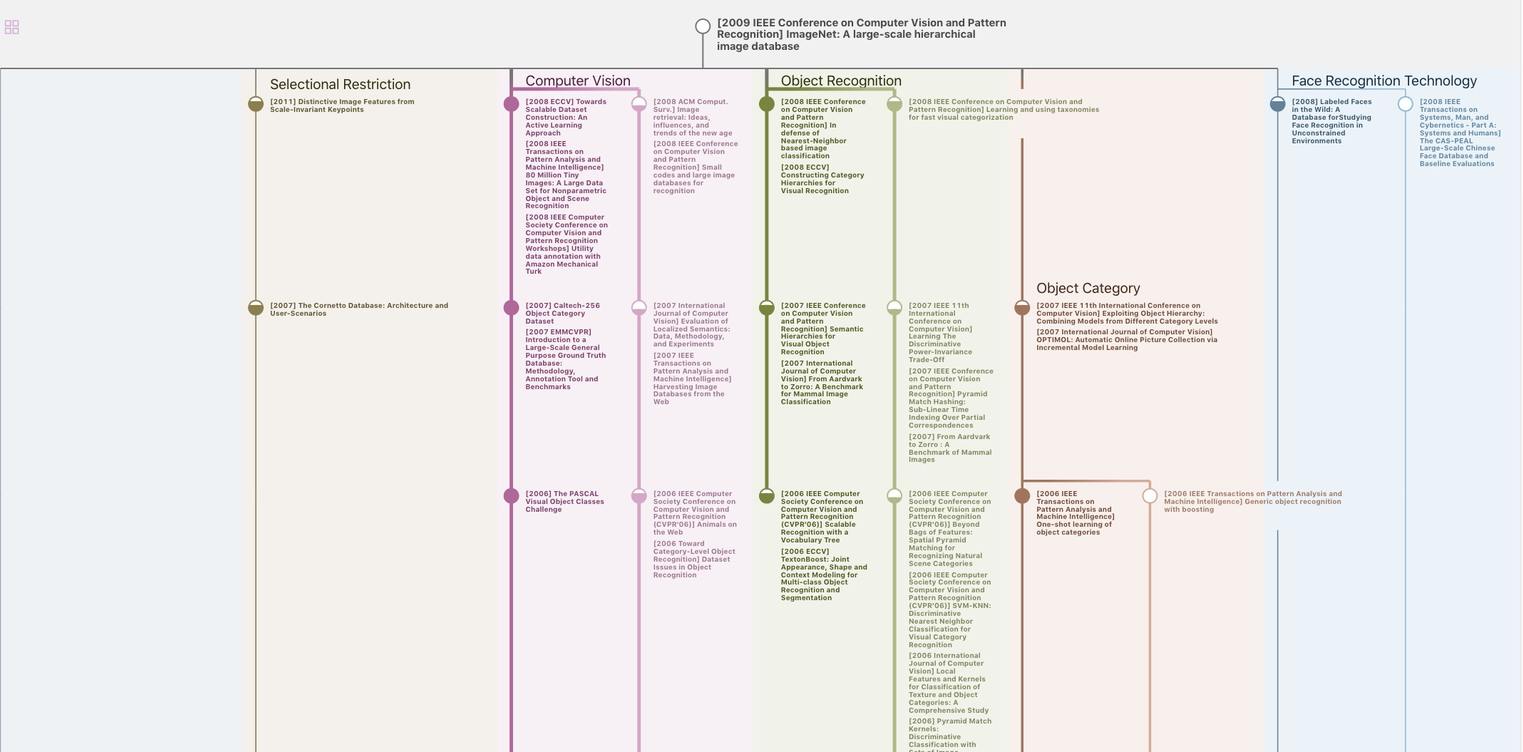
生成溯源树,研究论文发展脉络
Chat Paper
正在生成论文摘要