Value methods for efficiently solving stochastic games of complete and incomplete information.
NIPS(2014)
摘要
Solving multi-agent reinforcement learning problems has proven difficult because of the lack of tractable algorithms. We provide the first approximation algorithm which solves stochastic games with cheap-talk to within ∊ absolute error of the optimal game-theoretic solution, in time polynomial in 1/∊. Our algorithm extends Murray's and Gordon's (2007) modified Bellman equation which determines the set of all possible achievable utilities; this provides us a truly general framework for multi-agent learning. Further, we empirically validate our algorithm and find the computational cost to be orders of magnitude less than what the theory predicts.
更多查看译文
关键词
games
AI 理解论文
溯源树
样例
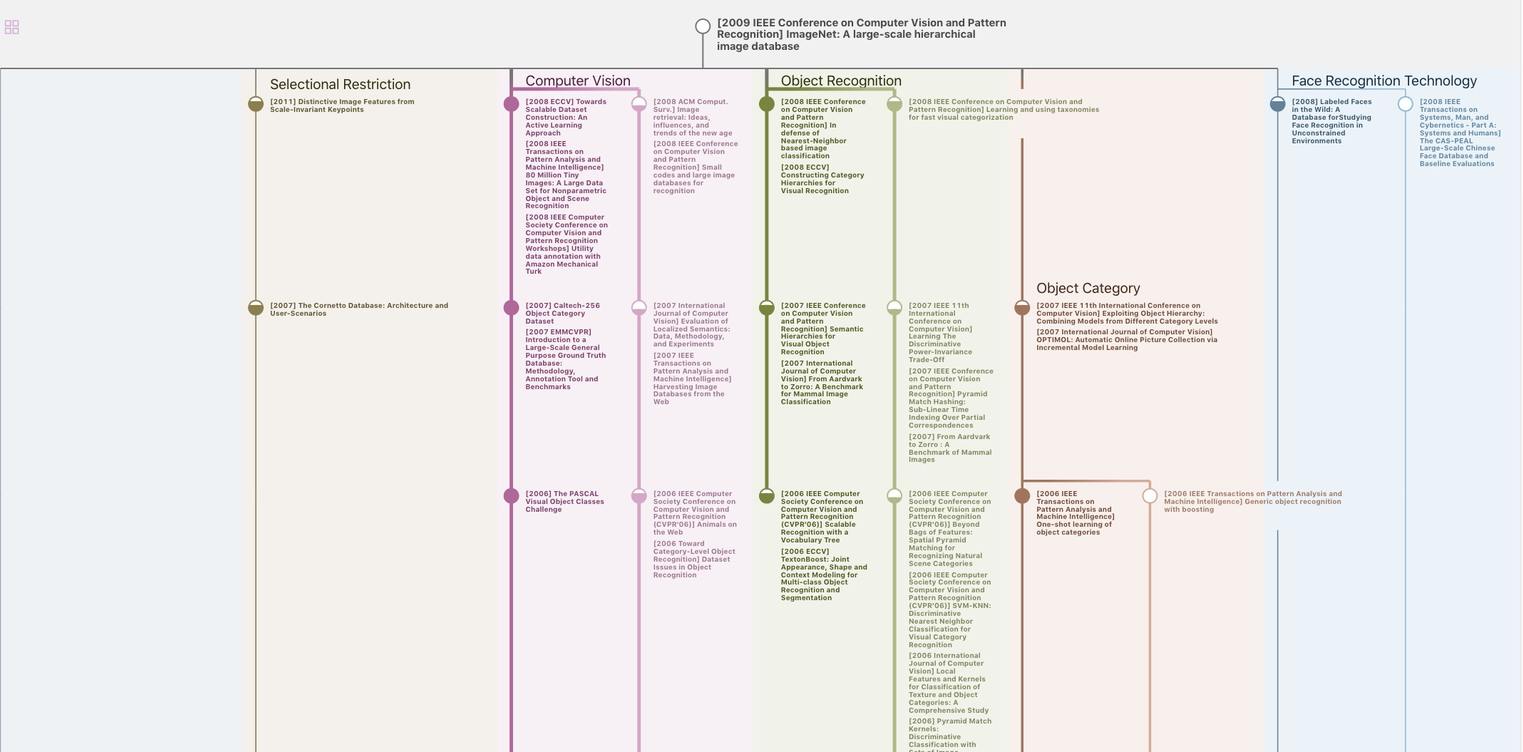
生成溯源树,研究论文发展脉络
Chat Paper
正在生成论文摘要