Multi-Stage Classification
IEEE International Conference on Data Mining(2005)
摘要
While much research has focused on methods for evaluating and maximizing the accuracy of classifiers either individually or in ensembles, little effort has been devoted to analyzing how classifiers are typically deployed in practice. In many domains, classifiers are used as part of a multi-stage process that increases accuracy at the expense of more data collection and/or more processing resources as the likelihood of a positive class label increases. This paper systematically explores the tradeoffs inherent in constructing these multi-stage classifiers from a series of increasingly accurate and expensive individual classifiers, considering a variety of metrics such as accuracy, cost/benefit ratio, and lift. It suggests architectures appropriate for both independent instances and for highly linked data.
更多查看译文
关键词
data collection,multi-stage classifier,multi-stage process,benefit ratio,expensive individual classifier,independent instance,paper systematically,positive class label increase,processing resource,Multi-Stage Classification
AI 理解论文
溯源树
样例
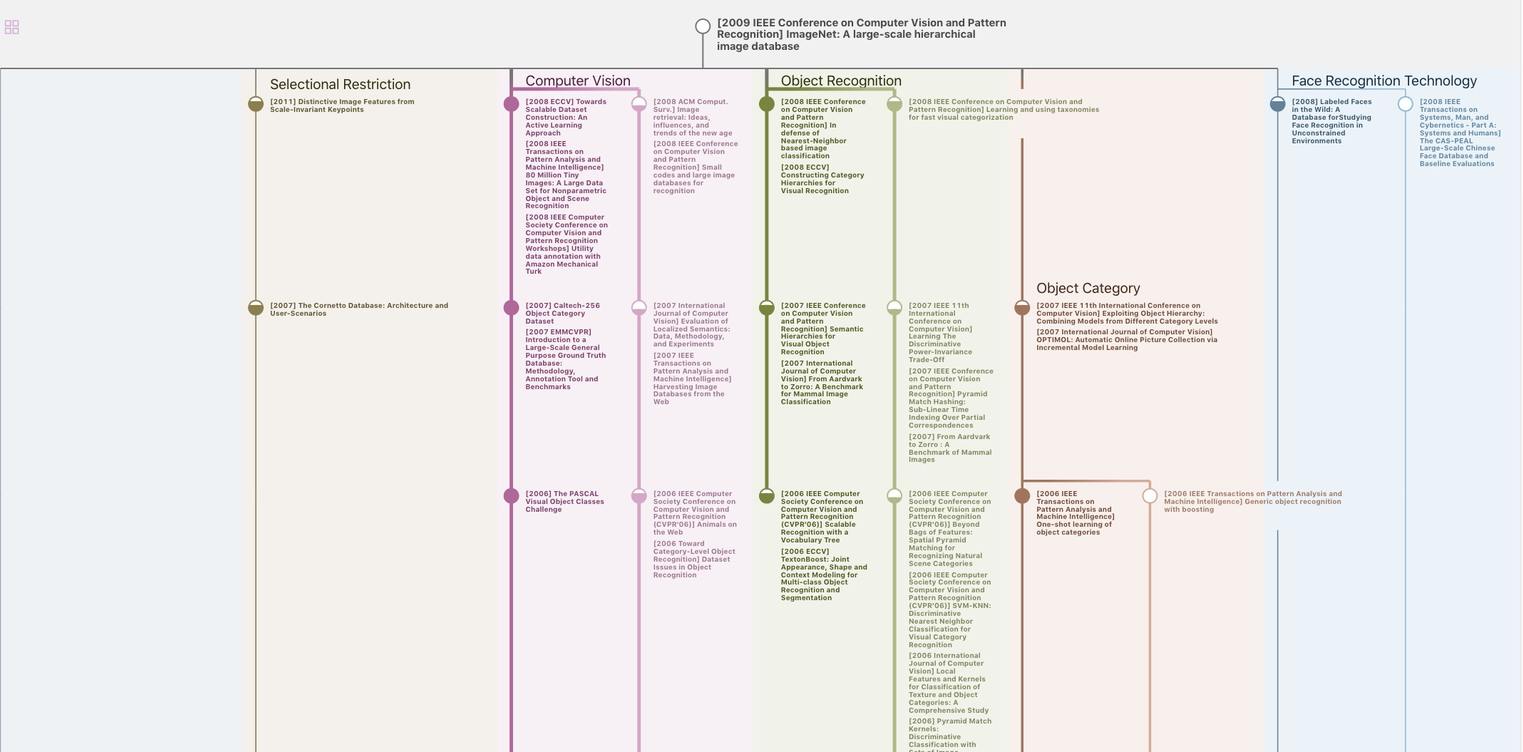
生成溯源树,研究论文发展脉络
Chat Paper
正在生成论文摘要