Unified Expectation Maximization.
NAACL HLT '12: Proceedings of the 2012 Conference of the North American Chapter of the Association for Computational Linguistics: Human Language Technologies(2012)
摘要
We present a general framework containing a graded spectrum of Expectation Maximization (EM) algorithms called Unified Expectation Maximization (UEM.) UEM is parameterized by a single parameter and covers existing algorithms like standard EM and hard EM, constrained versions of EM such as Constraint-Driven Learning (Chang et al., 2007) and Posterior Regularization (Ganchev et al., 2010), along with a range of new EM algorithms. For the constrained inference step in UEM we present an efficient dual projected gradient ascent algorithm which generalizes several dual decomposition and Lagrange relaxation algorithms popularized recently in the NLP literature (Ganchev et al., 2008; Koo et al., 2010; Rush and Collins, 2011). UEM is as efficient and easy to implement as standard EM. Furthermore, experiments on POS tagging, information extraction, and word-alignment show that often the best performing algorithm in the UEM family is a new algorithm that wasn't available earlier, exhibiting the benefits of the UEM framework.
更多查看译文
关键词
standard EM,Expectation Maximization,UEM family,UEM framework,hard EM,new EM algorithm,Lagrange relaxation algorithm,ascent algorithm,new algorithm,dual decomposition,Unified expectation maximization
AI 理解论文
溯源树
样例
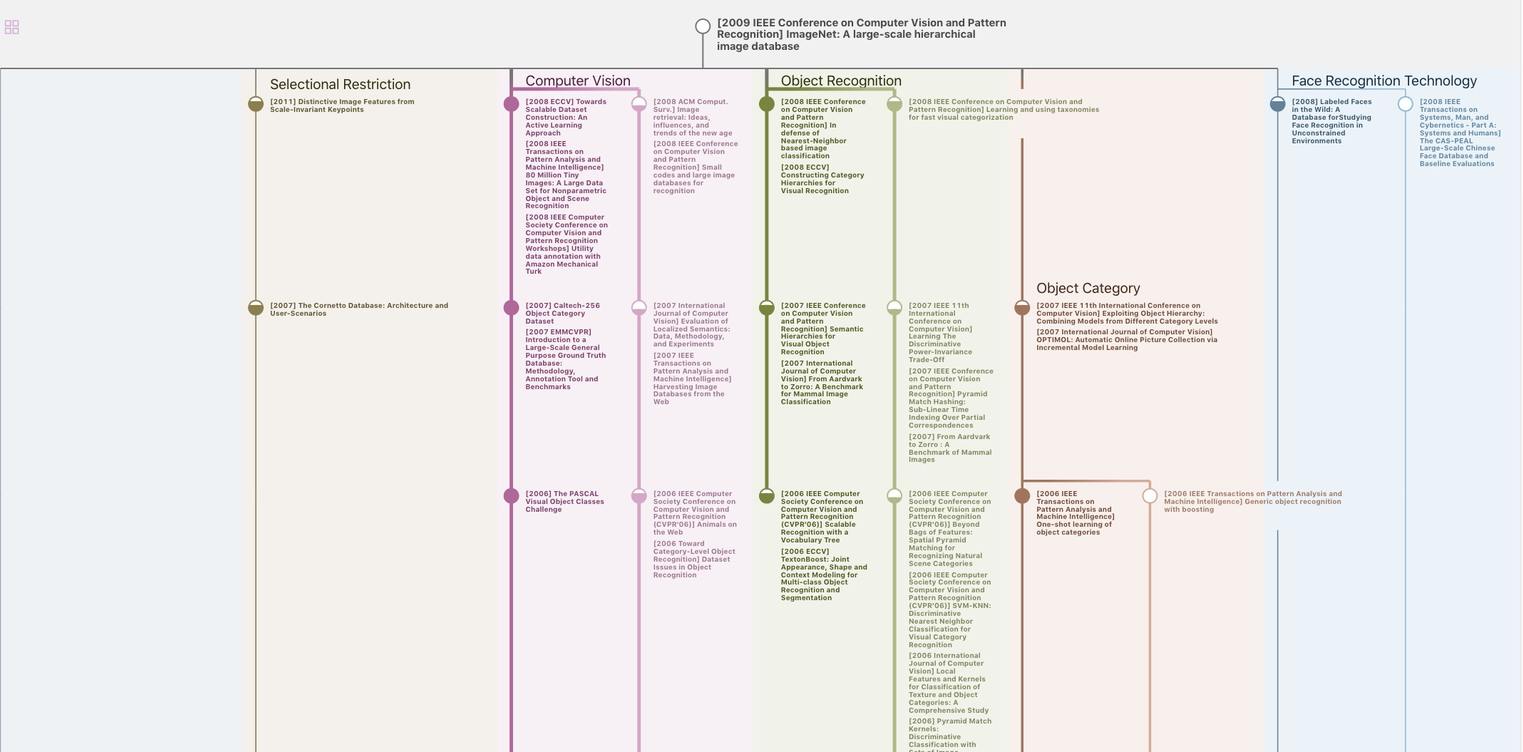
生成溯源树,研究论文发展脉络
Chat Paper
正在生成论文摘要