Robust hmm training and adaptation in hands-free speech recognition
msra(2007)
摘要
In this paper a challenging scenario is addressed in which a hands-free speech recognizer operates in a noisy office envi ronment with either batch or incremental model adaptation. The application of a microphone array processing compensates only for part of the mismatch between training and test ing acoustic conditions. In a previous work it was shown that the acoustic mismatch can be further reduced by conditioning hi dden Markov models to certain assumed operating acoustic condit i s. Conditioned HMMs are obtained by training using a filtered ve rsion of the clean speech corpus. In this work, starting from that result, we investigate the u se of conditioned models as initial models for both supervised batch adaptation and unsupervised incremental adaptation. Expe rimental results obtained for a hands-free connected digit recog niti n task show that models trained with filtered clean speech allo w to obtain better recognition performance than using models tr ained with clean speech with both batch and incremental model adap t tion.
更多查看译文
AI 理解论文
溯源树
样例
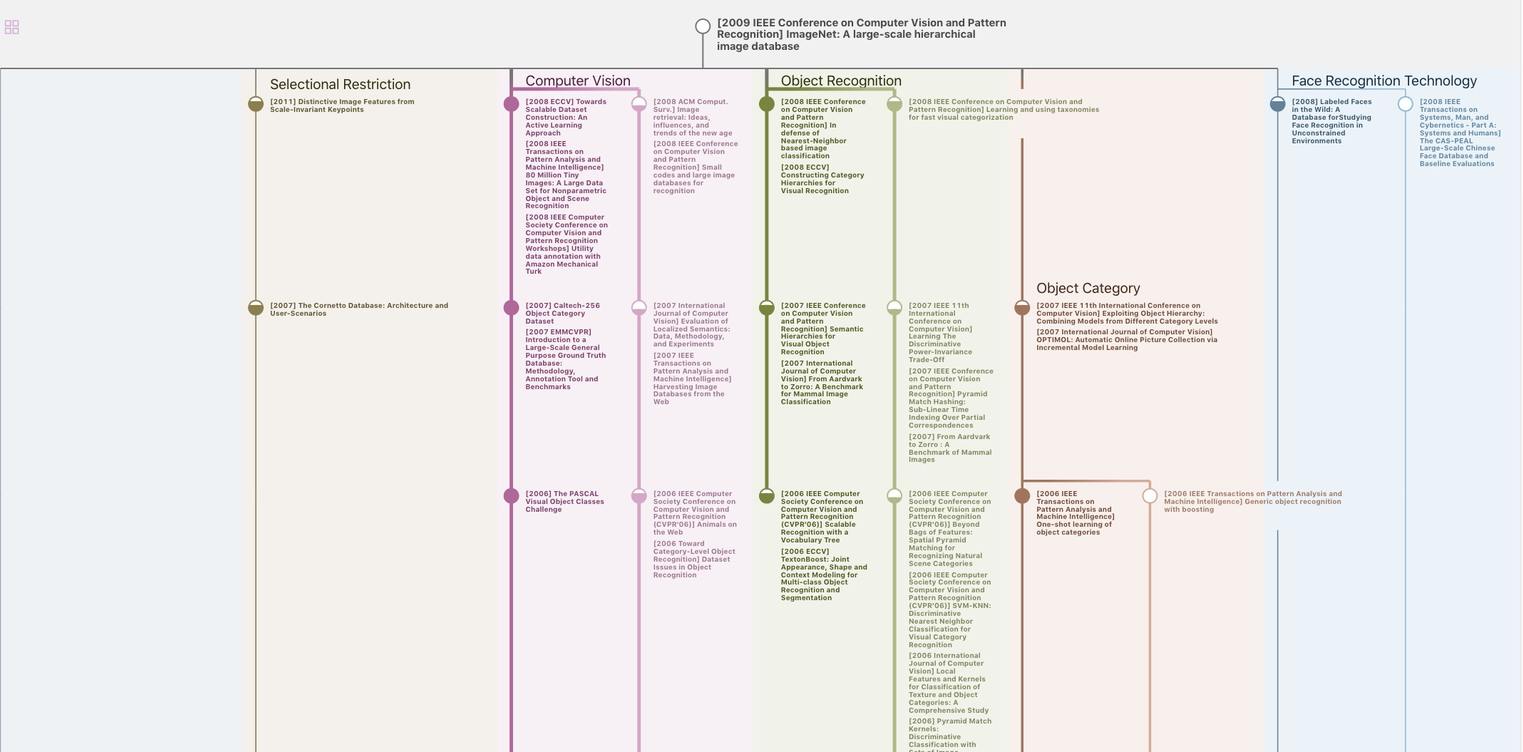
生成溯源树,研究论文发展脉络
Chat Paper
正在生成论文摘要