Distribution-Sensitive Construction of the Greedy Spanner
Algorithmica(2016)
摘要
The greedy spanner is the highest quality geometric spanner (in e.g. edge count and weight, both in theory and practice) known to be computable in polynomial time. Unfortunately, all known algorithms for computing it on n points take \(\varOmega (n^2)\) time, limiting its applicability on large data sets. We propose a novel algorithm design which uses the observation that for many point sets, the greedy spanner has many ‘short’ edges that can be determined locally and usually quickly. To find the usually few remaining ‘long’ edges, we use a combination of already determined local information and the well-separated pair decomposition. We give experimental results showing large to massive performance increases over the state-of-the-art on nearly all tests and real-life data sets. On the theoretical side we prove a near-linear expected time bound on uniform point sets and a near-quadratic worst-case bound. Our bound for point sets drawn uniformly and independently at random in a square follows from a local characterization of t-spanners we give on such point sets. We give a geometric property that holds with high probability, which in turn implies that if an edge set on these points has t-paths between pairs of points ‘close’ to each other, then it has t-paths between all pairs of points. This characterization gives an \(O(n \log ^2 n \log ^2 \log n)\) expected time bound on our greedy spanner algorithm, making it the first subquadratic time algorithm for this problem on any interesting class of points. We also use this characterization to give an \(O((n + |E|) \log ^2 n \log \log n)\) expected time algorithm on uniformly distributed points that determines whether E is a t-spanner, making it the first subquadratic time algorithm for this problem that does not make assumptions on E.
更多查看译文
关键词
Computational geometry,Spanners,Greedy spanner,Uniform points
AI 理解论文
溯源树
样例
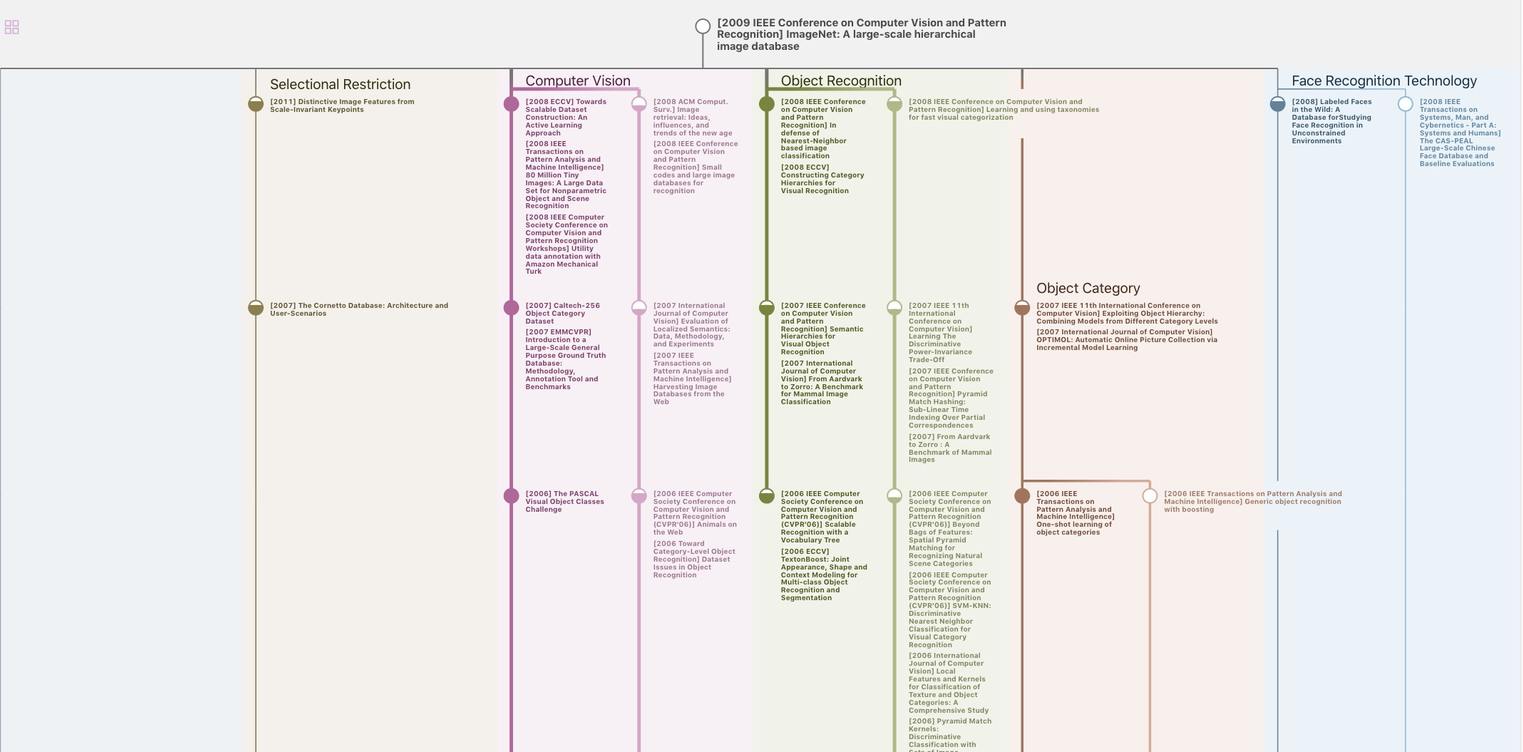
生成溯源树,研究论文发展脉络
Chat Paper
正在生成论文摘要