A Population Monte Carlo Scheme For Computational Inference In High Dimensional Spaces
2013 IEEE INTERNATIONAL CONFERENCE ON ACOUSTICS, SPEECH AND SIGNAL PROCESSING (ICASSP)(2013)
摘要
In this paper we address the Monte Carlo approximation of integrals with respect to probability distributions in high-dimensional spaces. In particular, we investigate the population Monte Carlo (PMC) scheme, which is based on an iterative importance sampling (IS) approach. Both IS and PMC suffer from the well known problem of degeneracy of the importance weights (IWs), which is closely related to the curse-of-dimensionality, and limits their applicability in large-scale practical problems. In this paper we investigate a novel PMC scheme that consists in performing nonlinear transformations of the IWs in order to smooth their variations and avoid degeneracy. We apply the modified IS scheme to the well-known mixture-PMC (MPMC) algorithm, which constructs the importance functions as mixtures of kernels. We present numerical results that show how the modified version of MPMC clearly outperforms the original scheme.
更多查看译文
关键词
Importance sampling, population Monte Carlo, mixture-PMC, degeneracy of importance weights
AI 理解论文
溯源树
样例
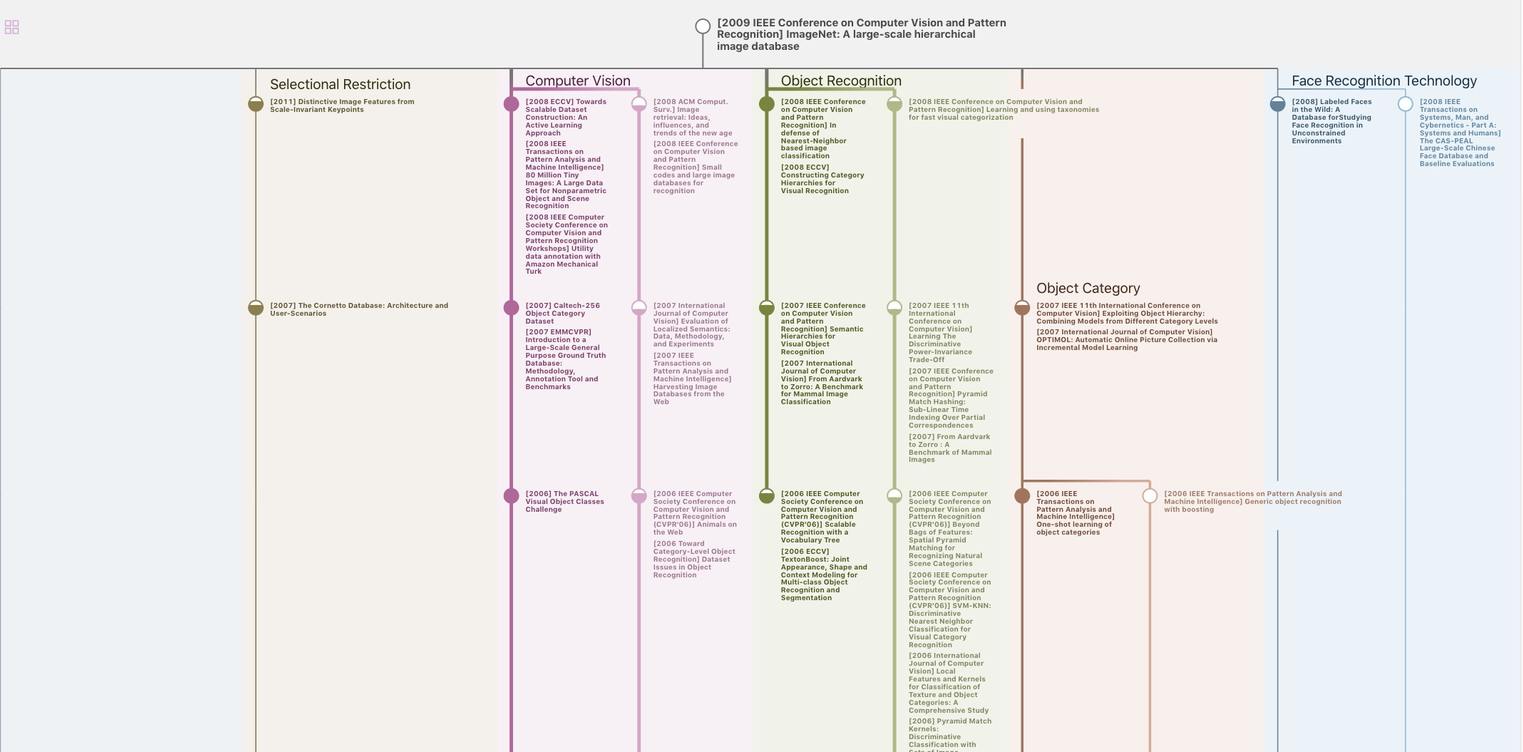
生成溯源树,研究论文发展脉络
Chat Paper
正在生成论文摘要