Efficient Market Making via Convex Optimization, and a Connection to Online Learning.
ACM Transactions on Economics and Computation(2013)
摘要
We propose a general framework for the design of securities markets over combinatorial or infinite state or outcome spaces. The framework enables the design of computationally efficient markets tailored to an arbitrary, yet relatively small, space of securities with bounded payoff. We prove that any market satisfying a set of intuitive conditions must price securities via a convex cost function, which is constructed via conjugate duality. Rather than deal with an exponentially large or infinite outcome space directly, our framework only requires optimization over a convex hull. By reducing the problem of automated market making to convex optimization, where many efficient algorithms exist, we arrive at a range of new polynomial-time pricing mechanisms for various problems. We demonstrate the advantages of this framework with the design of some particular markets. We also show that by relaxing the convex hull we can gain computational tractability without compromising the market institution’s bounded budget. Although our framework was designed with the goal of deriving efficient automated market makers for markets with very large outcome spaces, this framework also provides new insights into the relationship between market design and machine learning, and into the complete market setting. Using our framework, we illustrate the mathematical parallels between cost-function-based markets and online learning and establish a correspondence between cost-function-based markets and market scoring rules for complete markets.
更多查看译文
关键词
Market design,automated market maker,convex analysis,online linear optimization,prediction market,securities market
AI 理解论文
溯源树
样例
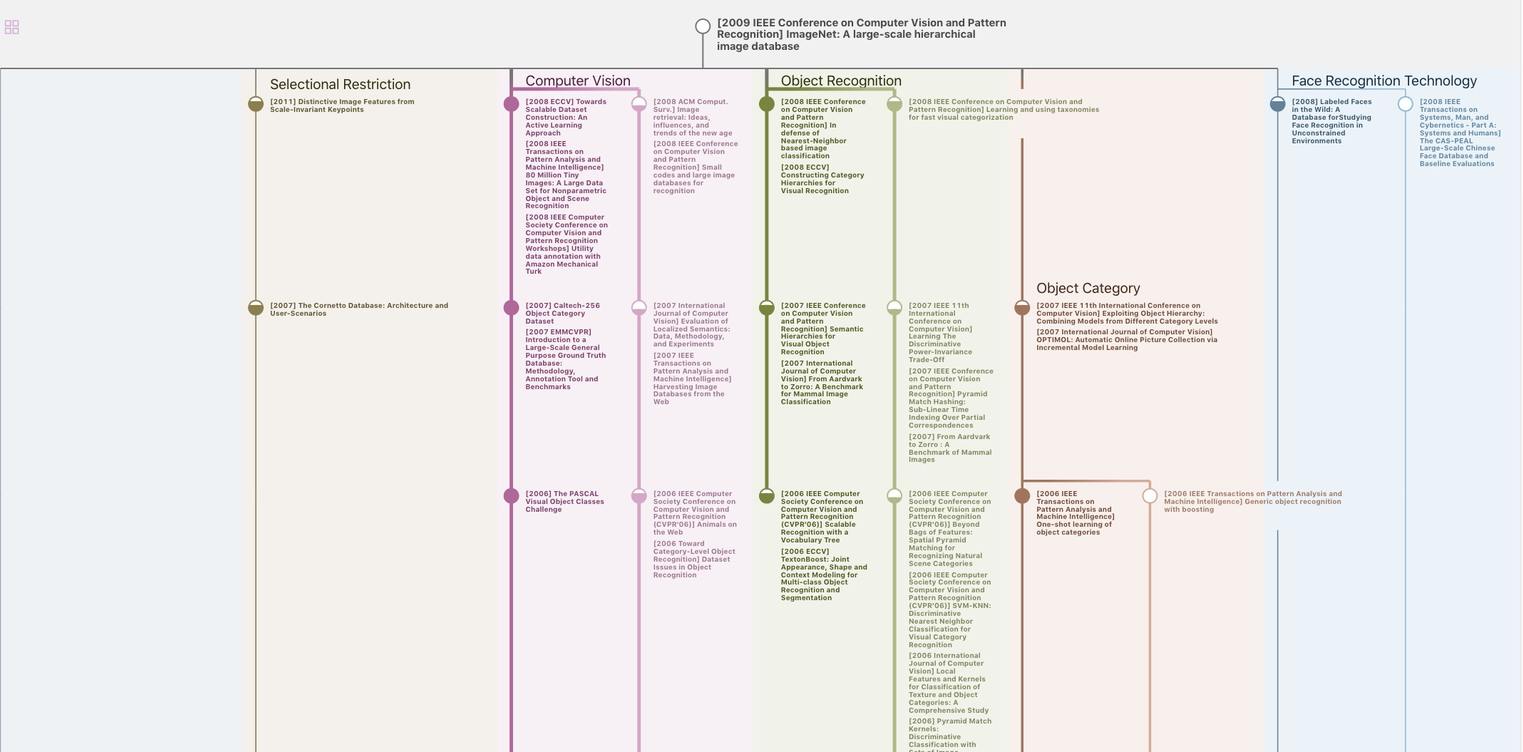
生成溯源树,研究论文发展脉络
Chat Paper
正在生成论文摘要