Online, Ga Based Mixture Of Experts: A Probabilistic Model Of Ucs
GECCO '11: Genetic and Evolutionary Computation Conference Dublin Ireland July, 2011(2011)
摘要
In recent years there have been efforts to develop a probabilistic framework to explain the workings of a Learning Classifier System. This direction of research has met with limited success due to the intractability of complicated heuristic training rules used by the learning classifier systems. In this paper, we derive a learning classifier system from a mixture of experts that is similar to a sUpervised Classifier System (UCS) in terms of its training and prediction routines. We start by framing the learning model as a mixture of experts which uses an Expectation Maximisation (EM) procedure to learn its parameters. The batch updates of the EM is then converted into online updates and finally into a GA based sampled online update thus ending up with a classifier system similar to a sUpervised Classifier System. In this paper, we show the effectiveness of such a system as compared to UCS through a series of comparative studies on test datasets.
更多查看译文
关键词
Learning Classifier System,probabilistic modeling,mixture of experts,UCS
AI 理解论文
溯源树
样例
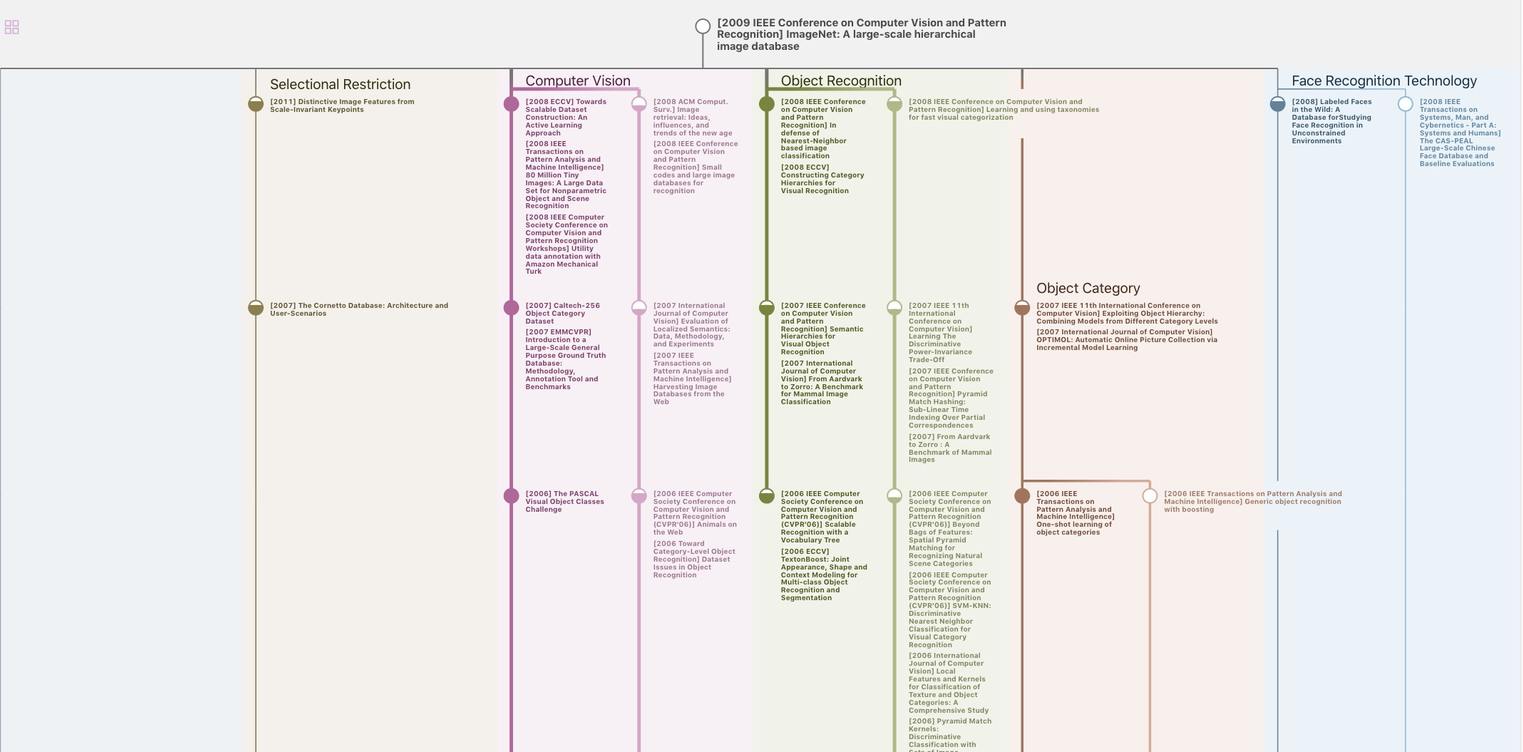
生成溯源树,研究论文发展脉络
Chat Paper
正在生成论文摘要