Prediction Of Long-Memory Time Series: A Tutorial Review
PROCESSES WITH LONG-RANGE CORRELATIONS: THEORY AND APPLICATIONS(2003)
摘要
Two different approaches, called Type-I and Type-II, to linear least-squares prediction of a long-memory time series are distinguished. In the former, no new theory is required and a long-memory time series is treated on par with a standard short-memory time series and its multistep predictions are obtained by using the existing modelling approaches to prediction of such time series. The latter, by contrast, seeks to model the long-memory stochastic characteristics of the observed time series by a fractional process such that its dth fractional difference, 0 < d < 0.5, follows a standard short-memory process. The various approaches to constructing long-memory stochastic models are reviewed, and the associated question of parameter estimation for these models is discussed. Having fitted a long-memory stochastic model to a time series, linear multi-step forecasts of its future values are constructed from the model itself. The question of how to evaluate the multistep prediction constants is considered and three different methods proposed for doing so are outlined, it is further noted that, under appropriate regularity conditions, these methods apply also to the class of linear long memory processes with infinite variance. In addition, a brief review of the class of non-linear chaotic maps implying long-memory is given.
更多查看译文
关键词
stochastic model,long memory,time series,parameter estimation
AI 理解论文
溯源树
样例
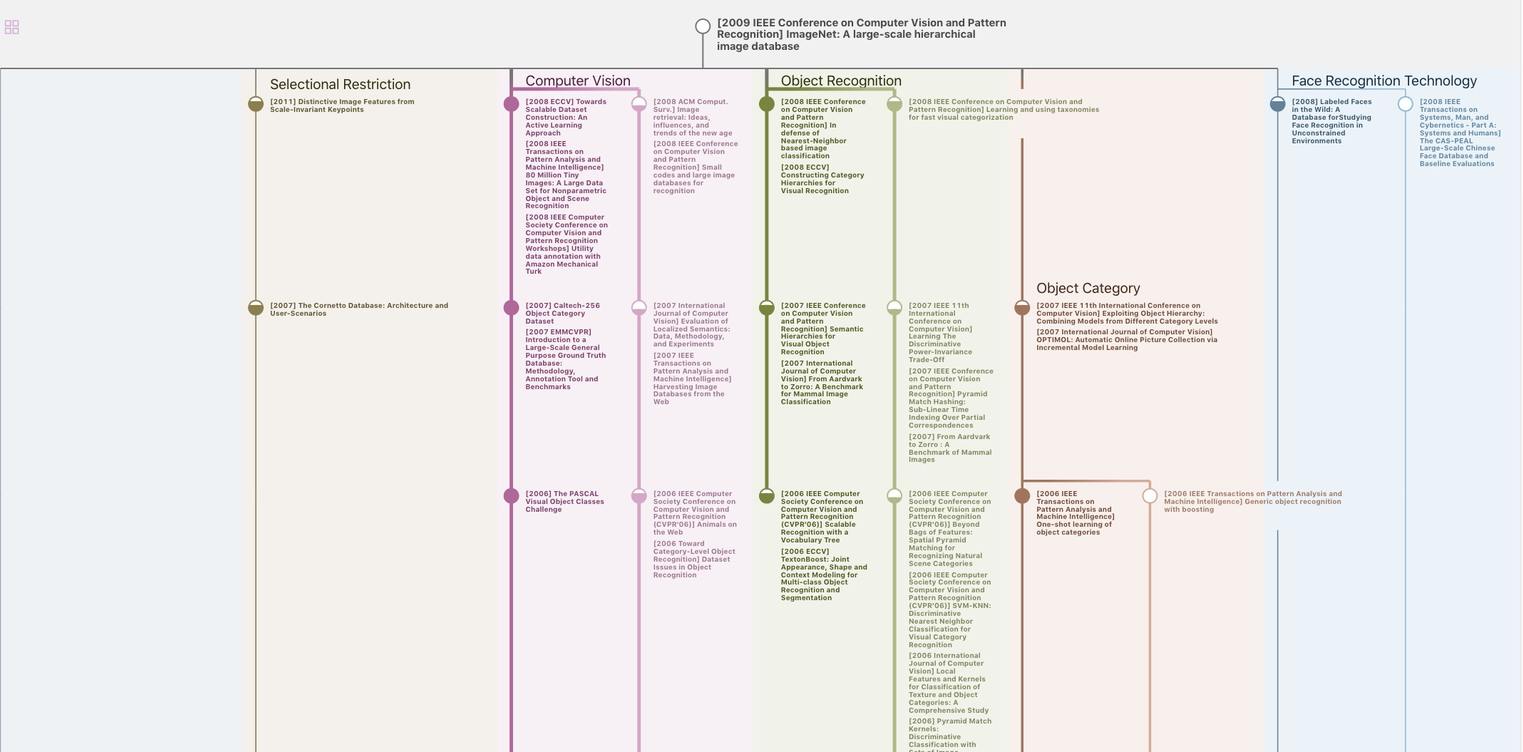
生成溯源树,研究论文发展脉络
Chat Paper
正在生成论文摘要