Napping for functional representation of policy
AAMAS(2014)
摘要
Reinforcement learning aims at learning a policy from interactions with the environment to maximize the long-term reward. In practice, we commonly expect that the policy can be a nonlinear mapping from the state features to the candidate actions, and thus has the ability to fit complex decision situations. Functional representation, by which a function is represented as a combination of basis functions, is a powerful tool for learning non-linear functions, and has been used in policy learning (e.g., the non-parametric policy gradient (NPPG) method). Despite the many unique advantages of functional representation, it has a practical defect that a functional represented policy involves a lot of basis functions, and consequently the policy learning algorithm will be costed a lot of time in calculating the many constituting basis functions. This defect will badly hamper the functional representation from being practically applicable in reinforcement learning tasks, as the complex policies are to be continually evaluated. In this work, we proposed the napping mechanism to improve the efficiency of using the functional representation, which periodically simplifies the generated function by a simple approximation model along with the learning process. We integrated the napping mechanism into the NPPG algorithm, and carried out empirical studies. Experiment results showed that the NPPG with napping can not only drastically improve the training and predicting speed from the original NPPG, but also improve the performance significantly.
更多查看译文
关键词
constituting basis function,complex policy,complex decision situation,policy learning,non-parametric policy gradient,nppg algorithm,basis function,original nppg,functional representation,napping mechanism
AI 理解论文
溯源树
样例
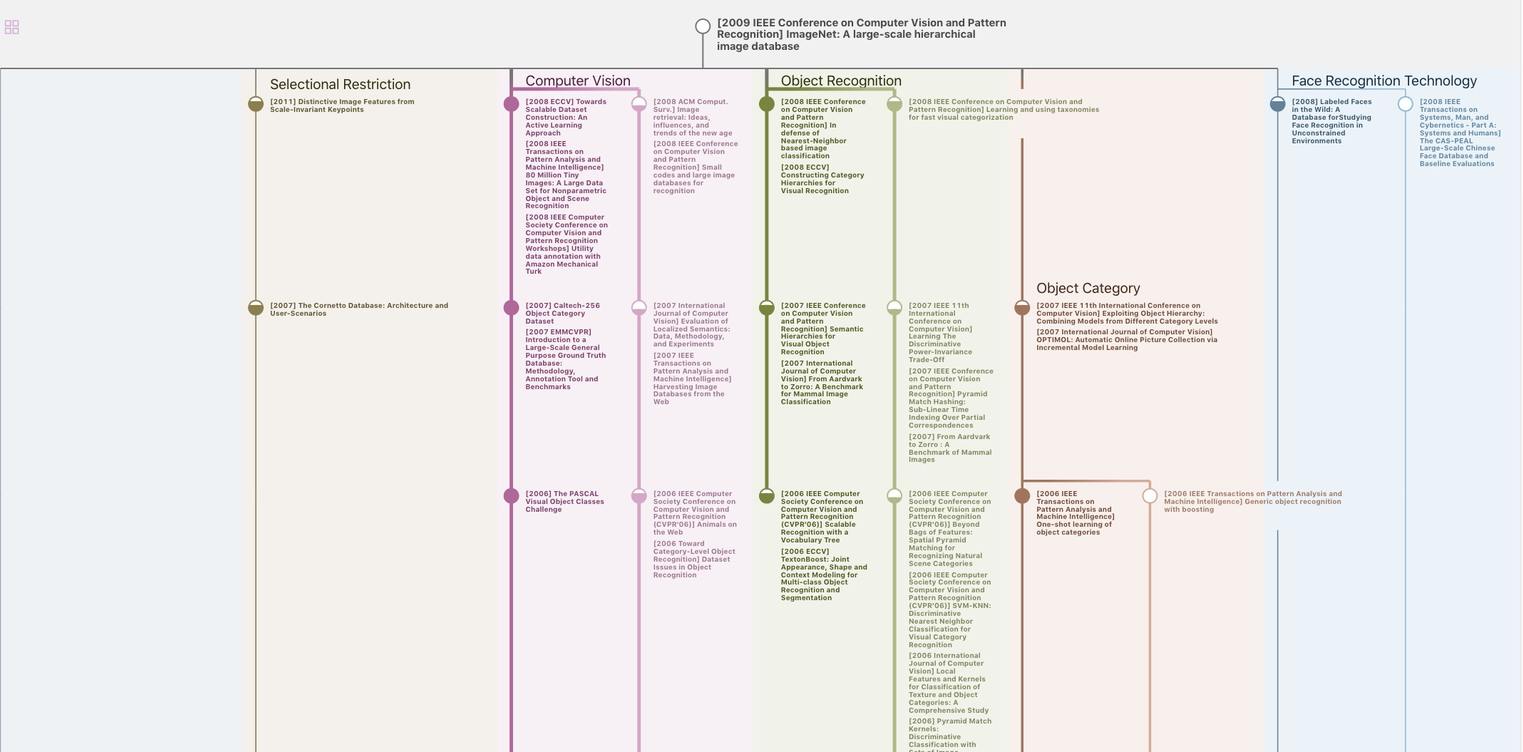
生成溯源树,研究论文发展脉络
Chat Paper
正在生成论文摘要